RDP 2020-01: Credit Spreads, Monetary Policy and the Price Puzzle 1. Introduction
January 2020
- Download the Paper 460KB
A higher cash rate dampens economic activity and reduces inflation. This is the standard prediction of conventional macroeconomic theory and guides policy decisions of all inflation-targeting central banks. Yet identifying and quantifying this relationship using standard empirical methods proves to be a challenge. Instead, researchers frequently find the opposite: increases in the policy rate – in Australia, the cash rate – are followed by higher inflation.[1] This ‘price puzzle’ is commonly believed to result from the inability of researchers' models to fully capture the central bank's anticipatory response to future inflation (Sims 1992; Hanson 2004; Romer and Romer 2004). As the central bank raises the policy rate when it expects inflation to rise in the future, the policy rate and inflation will be positively correlated unless the empirical model fully captures the central bank's inflation outlook. According to this explanation, causality runs in reverse – higher future inflation causes the central bank to raise its policy rate.
To resolve this issue, Romer and Romer ((2004), hereafter RR) propose removing the anticipatory component of monetary policy by purging policy rate changes of the central bank's systematic response to its own inflation forecasts. Controlling directly for the central bank's outlook in this way successfully removes the price puzzle in US data. But following the same approach for Australia, Bishop and Tulip ((2017), hereafter BT) find the price puzzle to persist across a wide range of model specifications. If anything, estimates from a standard SVAR using cash rate changes purged of the Reserve Bank of Australia's (the Bank) forecasts show an even stronger price puzzle (Figure 1, right panel) than estimates that use the raw cash rate series (Figure 1, left panel). Similarly puzzling findings are obtained for the effects on the unemployment rate. An increase in the cash rate leads to lower unemployment initially.
Having accurate estimates of the effects of monetary policy is obviously crucial for an inflation-targeting central bank. Therefore, the robustness of these puzzles to a range of specifications shown in BT has cast doubt on the reliability of the RR approach (and time series methods more generally) to accurately quantify the causal effects of cash rate changes not only on inflation and the unemployment rate, but on other key variables of interest as well. So what explains the robust emergence of the price puzzle even when the Bank's inflation outlook is accounted for? And what are the effects of a cash rate change on key macroeconomic outcomes such as inflation?
1994:Q1–2018:Q4
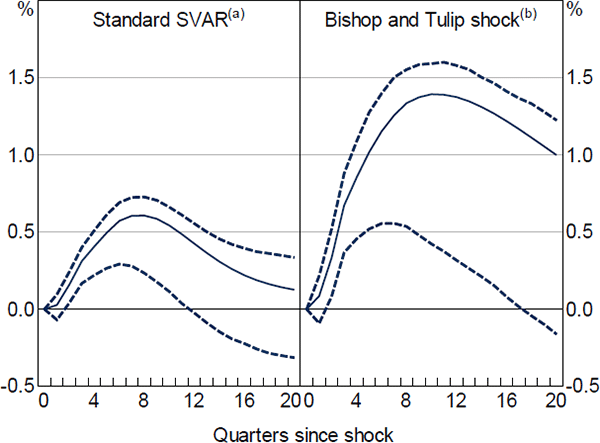
Notes:
Impulse responses from a recursive SVAR(4) including log underlying CPI, log real GDP, the unemployment rate and a monetary policy variable, in this order; dashed lines show 90% confidence intervals (bootstrapped)
(a) Policy variable is the cash rate
(b) Policy variable is the cumulated policy shock from Bishop and Tulip ((2017), updated)
In this paper I follow Caldara and Herbst (2019) and argue that the RR approach used by BT yields biased estimates of the true causal effects of monetary policy due to an omitted systematic and contemporaneous response of the cash rate to credit and money market conditions. Specifically, I show that the Reserve Bank Board responds immediately to expansionary financial market conditions (as indicated by low risk premia in lending and money market rates) by raising the cash rate over and above what the Bank's forecasts would demand. The cash rate response to these credit market conditions is sizeable. All else equal, a 100 basis point decline in money market risk spreads leads to a contemporaneous 60 basis point increase in the cash rate, and a 100 basis point decline in risk premia in large business lending rates is met by an 8 basis point cut to the cash rate.
But why does this additional response lead to the price puzzle when applying the RR approach to Australia? I document two further empirical relationships – each one on its own implying that the misspecification of the Bank's reaction function in BT violates two crucial conditions for the RR approach to provide unbiased estimates of the effects of monetary policy.
First, credit and money market risk spreads contain considerable additional predictive information for future inflation that is not captured by the Bank's forecast. In particular, I find inflation two to six quarters out to print systematically higher than forecast by the Bank when credit market conditions ease. While this supports the Board's decision to raise the cash rate to offset some of the inflationary impulse of easing financial conditions, it also violates the RR assumption that the Bank's forecasts contain all relevant information about the economic outlook on which the cash rate decision is based (RR; Ramey 2016). As a result, the RR ‘shock’ used by BT is, in fact, a mix between the true policy shock unrelated to the Bank's inflation outlook and the systematic, endogenous cash rate response to the inflationary effects of changing credit market conditions.[2] Accordingly, when estimates fail to take account of this systematic response, a future increase in inflation would be wrongly attributed to the higher cash rate rather than to expansionary financial market conditions.
Second, I show that cash rate changes – after accounting for the Bank's response to its own forecasts but not for the response to credit market conditions – are well anticipated by financial markets. This is unsurprising: if financial market participants were systematically surprised by the Board's response to publicly available financial market information, considerable profits could be earned by better predicting future cash rate changes. Accordingly, the RR shocks used by BT are anticipated and hence cannot be true structural policy shocks reflecting a primitive, unanticipated economic force that is unforecastable (and uncorrelated with other shocks) as in the definition of Ramey (2016) and Stock and Watson (2018). As a result, part of the effect of the BT shock would have affected some economic variables (e.g. the exchange rate) already before it is measured. This may be a further source of bias for the estimated policy effects in BT.[3] I demonstrate that a considerable part of this anticipatory component reflects the fact that market participants understand the Reserve Bank Board's systematic response to financial market conditions. As soon as the cash rate response to financial market conditions is taken into account, this predictable component shrinks considerably.
Taking these findings into account successfully resolves the price puzzle. To gauge the relevance of both potential sources of bias, I address them in turn. Specifically, I first construct a new series of monetary policy shocks that purges cash rate changes not only of the Bank's forecasts for inflation and economic activity but also of the response to several measures of risk premia in lending and money market rates. This shock still conceptually follows the RR approach and identifies shocks as a deviation of monetary policy from its historical reaction function. Using this series of shocks removes the price puzzle and leads to an earlier rise of the unemployment rate in response to a contractionary policy shock. However, I find that this policy shock measure is still partially anticipated by financial market participants.
To further remove this predictable component, I then also purge this shock series of the cash rate change expected by financial market participants ahead of the Board meeting. This two-step procedure follows Barakchian and Crowe (2013) and Miranda-Agrippino and Ricco (2018) and relates to the large literature that uses (high frequent) financial market information to identify policy surprises and uses these surprises as ‘shocks’ (e.g. Kuttner 2001; Cochrane and Piazzesi 2002; Gürkaynak, Sack and Swanson 2005; Gertler and Karadi 2015). While this information is ideal to ensure that the shock is unanticipated, in itself, it does not necessarily ensure that it is also exogenous to the Bank's outlook for the economy. However, this is not an issue for my final, preferred shock series which is purged of this component in the first step and thereby combines the benefits of both strands of the literature on identifying monetary policy shocks.
Using this new series of shocks I find that inflation, economic activity and the labour market respond in line with expectations. My results suggest that prices fall by around 0.7 per cent over the two years following a 100 basis point temporary increase in the cash rate, the unemployment rate peaks after six quarters at around one-third of a percentage point above its previous level and output falls by around 0.8 per cent over the course of one year. While subject to a considerable degree of uncertainty, the estimated response of inflation is about twice as large as the inflationary effects of a cash rate increase in MARTIN, the Bank's preferred macroeconometric model (Ballantyne et al 2019). In contrast, the estimated responses of output and the unemployment rate are closely in line with the dynamic responses in MARTIN. While this provides some comfort that MARTIN accurately captures the macroeconomic effects of a change to the Bank's key policy tool, it suggests that monetary policy may be more effective in raising or reducing inflation than suggested by MARTIN.[4]
Overall, my findings emphasise the importance for understanding the effect of credit market shocks not only to the wider economy, but also for the conduct and efficacy of monetary policy. As such, my results are closely related to the findings of Caldara and Herbst (2019) for the United States. Similarly to these authors, I find that omitting the systematic, contemporaneous response of monetary policy to exogenous changes in credit market conditions leads to biased estimates of the effect of policy on inflation. As the cash rate rises in response to a decline in credit risk premia, which in itself raises credit supply, economic activity and, ultimately, inflation, the bias is positive and the price puzzle emerges. For the RR identification strategy, however, this rests on my novel finding that changes in credit market conditions are an important source of the central bank's inflation forecast errors.
1.1 Why Does the Bank Adjust the Cash Rate but Not the Inflation Forecast?
The active cash rate response to credit market spreads in the apparent absence of an adjustment of the Bank's central forecasts to information in those spreads appears puzzling at first. I consider several explanations for this tension. First, the Reserve Bank Board (the Board) may rely on their own central forecasts when setting the cash rate. However, this appears unlikely since both forecasts and policy decisions are led by the Bank's Governor and Deputy Governor (Bishop and Tulip 2017). Second, when producing the forecasts, the Bank's staff may anticipate the Board's offsetting cash rate response. Again, this appears unlikely since changes to the cash rate are typically believed to take some time to affect inflation and I find credit spreads to explain the Bank's short- to medium-term forecast errors. Third, my proxies for credit market conditions may capture international (financial or monetary policy) conditions more broadly (Rey 2015). While international financial conditions are certainly important to Australia, I find that domestic money and credit market spreads explain the cash rate response even after accounting for the US policy rate or exchange rate movements.
Fourth, the predictive content of credit market indicators for the Bank's inflation forecast errors may reflect a lack of understanding on part of the Bank of the importance of smooth credit market functioning for the real economy prior to the global financial crisis (GFC) – a common criticism of central banks and the macroeconomics profession in the aftermath of the GFC (Debelle 2018).[5] Perhaps in line with this, I find some evidence that the Bank has learned from the GFC experience and now better integrates financial market developments into its economic outlook. In particular, the predictive content of credit market indicators for the Bank's forecast errors breaks down (in the admittedly short sample) after the GFC. Furthermore, I find that the cash rate has responded more decisively to changes in business lending rate spreads since the GFC, thereby more strongly offsetting any macroeconomic effects. However, the hypothesis of a lack of understanding of the importance the credit market conditions does not explain why the Board has responded to financial market conditions over the entire sample when setting the cash rate.
Finally, and most plausibly in my view, credit spreads may provide important information to the Board about the risks around the Bank's central, model-based forecasts. Accordingly, the Board may follow a risk management approach in which it weighs both ‘hard’, quantitative information that enters the Bank's central forecasts with ‘soft’, qualitative advice on the risks around those forecasts when setting the cash rate. Evans et al (2015) and Caggiano, Castelnuovo and Nodari (2018) provide evidence for this for the US Federal Reserve (the Fed), showing that it sets a lower policy rate than what its internal forecasts would suggest when uncertainty is elevated. Similarly, Sharpe, Sinha and Hollrah (2017) find that ‘tonality’ of the narrative around the Fed's forecasts predicts not only the Fed's forecast errors for GDP and unemployment, but also explains the policy decision over and above the Fed's forecasts. As I outline in the next section, credit and money market spreads may serve as a similar instrument informing the Reserve Bank Board about risks around the staff's central forecasts. During the GFC, this motivated strong cuts to the cash rate. Theoretical support for such a risk management approach has since been provided by Adrian and Duarte (2016), who argue that such an approach may be optimal at times of financial stress.[6] Importantly, while risks from financial stress are regularly discussed in the supporting documents provided to the Board by the Bank staff and in the Board meetings, they are not observed by the researcher who follows the RR approach. This may explain why the RR approach fails to recover the true causal effects of a cash rate change.
The body of this paper presents the arguments for bias in the RR approach arising from an omitted response to credit market conditions in detail. I begin by formally showing under which conditions the RR approach suffers from the same omitted variable bias that it aims to solve. I also provide some narrative evidence around the GFC, during which financial market conditions and risks to the outlook strongly influenced the decisions to lower the cash rate whilst the Bank's central forecasts were adjusted only with a considerable delay. In Sections 3 and 4, I then show that this relationship between credit spreads, the cash rate and the Bank's forecast errors is systematic such that both necessary conditions for bias in the RR approach are fulfilled. The cash rate responds to money market and credit spreads over and above the Bank's forecasts (Section 3), and these spreads explain the Bank's forecast errors for inflation (Section 4). I build on these findings in Section 5 to construct a new measure of monetary policy shocks for Australia and show that an increase in the cash rate reduces inflation and output, and raises the unemployment rate. I discuss the robustness of my results along several dimensions in Section 6. Section 7 concludes.
Footnotes
Rusnak, Havranek and Horvath (2013) find that half of 70 studies using structural vector autoregressions (SVARs) exhibit the price puzzle. But even for seminal studies that are free of the price puzzle, Ramey (2016) finds that many are not robust to small modifications to specification choices or identifying assumptions. For Australia, only one-third of the studies using SVARs find no price puzzle (see Appendix A). [1]
In other words, the identified policy ‘shock’ cannot be the true monetary policy shock as it is contemporaneously correlated with a financial market shock. In the related literature identifying the effects of macroeconomic shocks using external instruments this represents a violation of the exogeneity condition (Mertens and Ravn 2013; Gertler and Karadi 2015; Stock and Watson 2018). [2]
This relates to the issue of non-fundamentalness in SVARs when policy shocks are anticipated or announced prior to implementation, such as in the case of forward guidance (Leeper, Walker and Yang 2013; Ramey 2016). [3]
It should be noted that my estimates suggest smaller cash rate effects than recent estimates from a factor augmented VAR model by Hartigan and Morley (2018). Providing external validity to models like MARTIN is important since the dynamic properties of MARTIN (or any large-scale macroeconometric model) may be sensitive to misspecification of only a few of the model's many equations. Such misspecifications can, for example, arise from the fact that MARTIN relies partly on calibrated relationships between some variables to ensure satisfactory long-run properties of the model. [4]
This is not to say that the role of financial markets for the real economy was ignored prior to the GFC. Examples include Bernanke and Gertler (1989), Bernanke, Gertler and Gilchrist (1999) and Kiyotaki and Moore (1997), who emphasise the role of household and firm balance sheets as amplifiers of business cycles. But since the GFC, this literature has grown rapidly to show that shocks originating in credit markets can have considerable effects on the real economy (see Gilchrist and Zakrajšek (2012), Bassett et al (2014), Gambetti and Musso (2017) and López-Salido, Stein and Zakrajšek (2017) for international evidence; and Jacobs and Rayner (2012) and Finlay and Jääskelä (2014) for Australia). [5]
The additional response of monetary policy to financial conditions suggested by Adrian and Duarte (2016) is, however, motivated by the predictive value of credit market indicators for future output over and above past realisations of all other variables. If the central bank's forecasts were to fully centralise this information, responding to these forecasts should deliver the same policy decision. [6]