RDP 2015-13: Seasonal Adjustment of Chinese Economic Statistics 1. Introduction
November 2015 – ISSN 1448-5109 (Online)
- Download the Paper 1.18MB
The growth in importance of China as a driver of global trade flows, and its resilience in the face of slower growth in the advanced economies since the global financial crisis, have led to a much greater degree of attention being focused on monthly and quarterly releases of Chinese macroeconomic statistics. For China's trading partners, such as Australia, rapid or unexpected changes in Chinese data have the ability to move markets, and can lead to revised assessments of domestic economic prospects.
Most monthly and quarterly macroeconomic time series in China are subject to seasonal fluctuations. Agricultural production, for instance, naturally varies with the seasons, giving rise to seasonal movements in food prices (especially for products that are costly to store). Sales of consumer goods tend to spike prior to public holidays, resulting in a strong seasonal pattern in Chinese retail sales. The purpose of seasonal adjustment is to filter, or ‘look through’, the volatility resulting from the effects of seasonality in original data. Ideally, seasonal adjustment should clarify whether a movement in a given series is larger than would be expected, given knowledge of the regular seasonal pattern for that series. But otherwise regular seasonal patterns in China are often clouded by calendar effects associated with traditional festivals, the timing of which is dictated by the lunar calendar and therefore varies from year to year on the Gregorian calendar.
The timing of Chinese New Year on the Gregorian calendar fluctuates between 21 January and 20 February. This gives rise to distortions in simple computations for assessing the rate of change in Chinese indicators that are widely used in practice, such as year-on-year (year-ended) growth or inflation rates. The reliance on year-on-year growth rates to assess economic conditions is related to the emphasis that has, historically, been placed on these comparisons by China's National Bureau of Statistics (NBS) (Orlik 2011). Until 2011, the NBS did not publish seasonally adjusted data, and at present only a handful of series are available in this form.
The most obvious counterpart of Chinese New Year in Western countries is Easter, which moves between March and April on the Gregorian calendar. Figure 1 compares estimates of Easter holiday factors for the level of the money supply (M2) stock in the United States, and Chinese New Year holiday factors for the same series in the People's Republic of China (see the next section for details), normalised in each case by subtracting the mean and dividing by the standard deviation. Similar to Easter, the effects of the week-long Chinese New Year public holiday vary over time, and give rise to a substantial distortion of economic time series. The magnitude of the effect is apparent from visual inspection of a range of Chinese data, and is consistent with casual observation. Every year, the Chinese New Year holiday receives international attention as the ‘world's largest human migration’ (Larson 2014), with millions of Chinese citizens travelling home for the holidays. The associated mass movement of people gives rise to noticeable variation in consumption and production patterns across the country. This is easily observable from visual inspection of a typical data series, such as Chinese power generation (Figure 2).
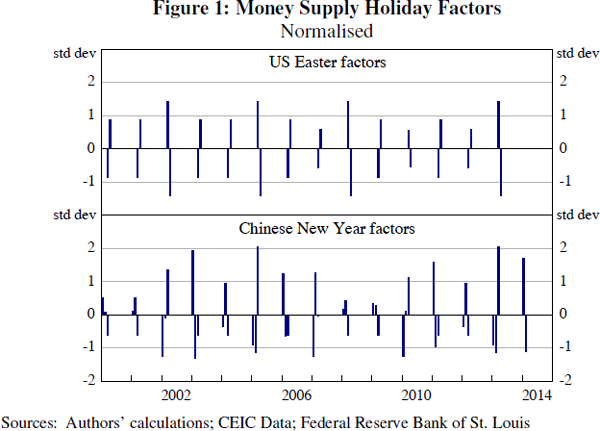
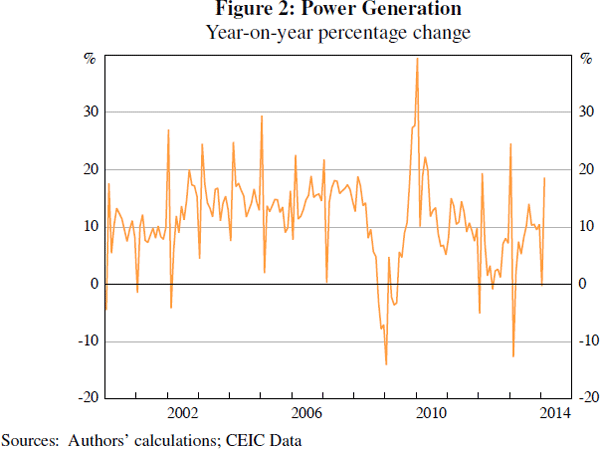
This paper shows that, applied with care and appropriate modifications, seasonal adjustment procedures such as the US Census Bureau's X-12-ARIMA (Findley et al 1998) and the Bank of Spain's SEATS (Gómez and Maravall 1996; Maravall 1999) can assist in the timely interpretation of a range of commonly used Chinese macroeconomic indicators. We do not find that any particular seasonal adjustment procedure is dominant. If suitably adapted to the features of Chinese data, both X-12-ARIMA and SEATS can yield seasonal adjustments that compare favourably to simpler approaches.
To account for the effect of moving holidays on Chinese data within the X-12-ARIMA and SEATS frameworks, we use dummy variable corrections, similar to the approach used by Bell and Hillmer (1983) to address the effects of moving festivals such as Easter. Although the use of dummy variable corrections to account for Chinese New Year is not new (Lin and Liu 2003; Shu and Tsang 2005; PBC 2006), we propose a simple extension. Using an historical documentation of public holidays associated with Chinese New Year, we attempt to optimise the selection of these corrections prior to seasonal adjustment. We conjecture that this allows more precise accounting for seasonal patterns than either an ad hoc choice of these corrections or the alternative method of simply averaging January and February observations prior to adjustment. Our procedure also extends existing approaches by explicitly taking into account the effects of the two main other, shorter lunar holidays, the Dragon Boat festival (duanwujie) in May/June and the Mid-Autumn festival (zhongqiujie) in September/October.
After adjusting for holiday effects and applying the above seasonal adjustment procedures, we assess the quality of our adjusted series using standard methods. In addition, we consider the practical performance of seasonally and holiday adjusted data against two simpler, but widely used, techniques: regression on seasonal dummies, and the calculation of year-on-year growth rates as a ‘rule of thumb’ adjustment for seasonality.
To date, little research has been published on the application of seasonal filters to time series for the People's Republic of China.[1] This paper aims to help fill that gap. Analyses of data from other economies include Lin and Liu (2003), who describe an application of X-12-ARIMA to 10 economic series in Taiwan. Woon (2011) finds that both X-12-ARIMA and SEATS yield ‘acceptable’ seasonal adjustments of Korean time series in the presence of the traditional holidays such as Seollal (lunar New Year's Day) and Chuseok (Korean Thanksgiving Day). Shuja, Lazim and Wah (2007) use a similar approach to adjust Malaysian data in the presence of moving calendar effects arising from traditional holidays including Islamic observances such as Eid-ul Fitr and Eid-ul Adha, the Hindu festival of Deepavali and Chinese New Year.[2]
Studies that use Chinese data use a range of approaches to correct for seasonality and moving holidays. Some studies choose very simple approaches. For instance, Sun's (2013) analysis of monetary policy shocks in China uses unadjusted industrial production data compiled on a year-on-year growth basis, with prior removal of January observations to mitigate Chinese New Year effects. It is also common to correct for seasonality in the context of an econometric model using deterministic dummy variables (e.g. Marquez and Schindler 2007; Garcia-Herrero and Koivu 2009; Zuo and Park 2011; Cheung, Chinn and Qian 2012). However, regression on seasonal dummies can be infeasible in large-scale models (Burman 1980), and is not robust to seasonality that changes over time. Our results suggest that the seasonal pattern in China is likely to be changing over time, which casts doubt on the accuracy of such an approach.
Seasonal adjustment procedures such as X-12-ARIMA have been used in a number of recent studies involving Chinese data.[3] In their factor-augmented VAR study, Fernald, Spiegel and Swanson (2014) use X-12-ARIMA to adjust for seasonality in 29 Chinese economic time series, after removing Chinese New Year effects by averaging January and February observations. Similarly, in their study of the relationship between Chinese and developed economy business cycles, Jia and Sinclair (2013) use quarterly real output data that have been pre-adjusted using X-12-ARIMA. The use of seasonally adjusted Chinese data in current applied work and the growing appetite for adjusted data among Chinese policymakers (revealed by the 2011 decision by the NBS to publish seasonally adjusted figures for key series) provide an additional motivation to investigate the application of seasonal adjustment procedures to Chinese time series.
The paper proceeds as follows. The next section gives an overview of the X-12-ARIMA and SEATS approaches to seasonal adjustment and how they may be adapted in the presence of calendar effects due to Chinese moving holidays. Section 3 describes the data and discusses the results of our seasonal adjustment of 13 selected time series. It compares the results with simpler approaches to smoothing seasonal fluctuations and provides some sensitivity analysis. Section 4 offers brief concluding remarks.
Footnotes
Exceptions includes Shu and Tsang (2005) and PBC (2006). [1]
Liou, Lin and Peng (2012) make use of daily data on monetary aggregates to construct Perng's (1982) bell-shaped holiday variables and feed them into X-12-ARIMA. As we lack comparable daily data for most Chinese economic time series, we are unable to consider this intriguing alternative. [2]
The technical literature is divided on the question of whether pre-adjusted data should be used at all in econometric and applied theoretical models, as it can give rise to bias in estimated parameters (Ghysels 1988; Franses 1996; Saijo 2013). Contributions to this literature advise attention to seasonal unit roots and seasonal cointegration. Several recent applications to Chinese data have followed this advice (e.g. Delatte, Fouquau and Holz 2011; Tang, Selvanathan and Selvanathan 2012; Hererias 2013). [3]