RDP 2007-03: Forecasting with Factors: The Accuracy of Timeliness 3. The Composition of Forecast Models
April 2007
- Download the Paper 296KB
In this study we use a quarterly panel because most of the key Australian macroeconomic time series that one would wish to forecast are available at a quarterly frequency, including the consumer price index (CPI). Further, over the long sample period used in this study, there are relatively few monthly series available for Australia that comprise a representative cross-section of the economy.
Table 1 summarises the composition of our data panel according to the type of economic series. The panel includes 53 series in total and spans the period 1960–2005 (see Appendix A for a full list of the data series). The composition of the data panel is crucial for ensuring that the estimated factors are representative of the aggregate economy. As Gillitzer, Kearns and Richards (2005) discuss, a panel that has a balance of series representing different aspects of the economy is more likely to produce factors that reflect the entire economy. For instance, if the data panel contained a disproportionate number of labour market series, the estimated factors would more closely approximate the state of the labour market than the aggregate economy. The data panel is also constrained by our desire to have a long data sample to ensure that the results are robust to structural change and the state of the economic cycle. Because real-time data are not available for all series in our panel we use final vintage data.
Type of series | Number of series |
---|---|
National accounts | 21 |
Employment | 8 |
Industrial production | 4 |
Building and capital expenditure | 5 |
Internal trade | 1 |
Overseas trade and current account | 5 |
Prices | 5 |
Financial data | 4 |
Total | 53 |
While the data panel contains fewer series than used in similar studies, this need not result in less accurate forecasts. Broader data panels typically include many highly disaggregated series, such as regional series or subcomponents of the series that we have used. These may contain more noise and correlated idiosyncratic dynamics, thereby potentially reducing the information content of the estimated factors (see Boivin and Ng 2006).
The factor models we estimate require the data to be in stationary form, which for most series, such as GDP, we achieve by taking a log-difference, leaving the data in approximate percentage change form. Series such as business surveys and the unemployment rate are already stationary and so require no transformation.
We forecast eight important macroeconomic series representing different aspects of the economy: growth in GDP, non-farm GDP, private final demand, household final consumption expenditure, employment, the number of building approvals, CPI inflation and the unemployment rate.
The publication lag for the data in the panel is shown in Figure 1. Using the release dates for 2006:Q1, it shows the number of series in the panel that have been published at a given time relative to the end of the quarter that those data cover. While the release dates for series in the panel may have changed over the sample, the timing depicted in Figure 1 presents the data availability constraints currently faced by forecasters, and is representative of the order in which series have been released over the long sample period used in this study.
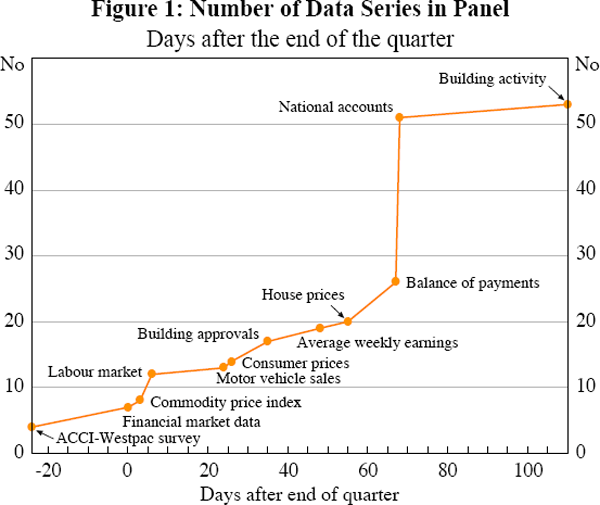
The most timely series in the panel are from the ACCI-Westpac survey of manufacturers, and arrive around 20 days before the end of the relevant quarter. The financial market data in the panel are available within a few days of the end of the quarter, followed about a week later by the labour market data, while many real and nominal series become available between approximately 3–8 weeks after the end of the quarter.[3] Some of the least timely series in the panel are the balance of payments and national accounts data, which are available about 10 weeks after the end of the quarter.
Many similar types of economic series – for example, financial market series or labour market series–are typically released at about the same time. Consequently, prior to the point at which all the series become available, the subset of the data panel with observations for the base quarter will not be representative of the full panel as it will exclude those groups of related series yet to be released. Given the constraints of release dates, this is unavoidable. If national accounts series are not released until 10 weeks after the end of the base quarter, then the data panel used 9 weeks after the end of the quarter cannot include the base quarter's data for any of these series. However, our choice of series ensures that each subset of series that include the base quarter's data is as representative as possible, given data availability. For example, while the group of series with data for the base quarter available 10 days after the end of the quarter will not contain production data, it will have a reasonable balance of survey, financial market and labour market series.
Footnote
Financial market data covering part of the quarter would be available contemporaneously, but to keep the exercise tractable, we only consider the data covering the full quarter. [3]