Research Discussion Paper – RDP 2022-07 The Term Funding Facility: Has It Encouraged Business Lending?
December 2022
1. Introduction
The Term Funding Facility (TFF) was introduced by the Reserve Bank of Australia (RBA) as part of a policy package in response to the onset of the COVID-19 pandemic. As information around the severity of the new virus emerged, it became clear that restrictions on activity imposed by governments and significant changes in the behaviour of households and businesses would lead to a large fall in GDP. Moreover, as investors responded to new information about the spread of the virus, financial markets experienced a period of dysfunction, raising the possibility that finance would be difficult to access for an extended period, exacerbating the economic downturn.
On 19 March 2020, the RBA introduced a range of measures to lower funding costs and encourage the provision of credit, thereby supporting economic activity: a reduction in the cash rate to 25 basis points; a change in the rate of remuneration of exchange settlement balances to 10 basis points; a target for the 3-year yield on Australian Government Securities (AGS) of around 25 basis points; an increase in the amount and term of repo operations; and the TFF.
The TFF provided authorised deposit-taking institutions (referred to hereafter as ‘banks’) with access to low-cost funding for a term of three years. The facility had three key objectives:
- Support the banking sector to continue to extend credit to households and businesses at a time when wholesale funding markets had been significantly disrupted.
- Lower funding costs for banks and, in turn, reduce borrowing rates for their business and household customers.
- Encourage banks to increase their lending to businesses, particularly small and medium-sized enterprises (SMEs).
Banks were granted an ‘initial allowance’ equivalent to 3 per cent of total credit outstanding, to be drawn by end September 2020. The cost of funding under the TFF was well below the cost of funding in wholesale markets. By providing banks with an assured source of funding at low cost, the TFF achieved the first two objectives above. To address the third objective, the TFF also granted an ‘additional allowance’ to those banks that expanded their business credit relative to a pre-pandemic baseline.
On 1 September 2020, the RBA announced that the deadline for TFF drawdowns would be extended to 30 June 2021 and provided a further supplementary allowance of 2 per cent of credit.
The TFF provided banks with an assured source of funding and lowered their funding costs, both directly and indirectly. The TFF reduced funding costs directly because it had a lower price than alternative sources of funding at the same term. Notably, it provided funding to all eligible lenders at the same rate, and so effectively was more advantageous to lenders with higher costs of funding (lower credit ratings). In addition, the TFF led to lower funding costs through various indirect channels, including by causing the supply of bank bonds to decline, which reduced bond spreads and increased the level of deposits held at banks (Kent 2021b; Black, Jackman and Schwartz 2021).[1]
Furthermore, lower levels of bank bond issuance caused spreads on asset-backed securities (ABS) to decline, as investors sought securities with similar credit risk, thereby benefiting non-bank lenders (Figure 1). Non-bank lenders are finance providers that do not accept deposits, such as finance companies and money market corporations. The reduction in banks' funding costs flowed through to lower interest rates charged to households and businesses, with the largest declines for rates for larger businesses and new fixed-rate housing loans (Figure 2). It is clear that the TFF reduced banks' funding costs and led to lower interest rates throughout the economy. The focus of this paper, however, is on the third objective of the TFF: namely, whether the TFF supported business lending.
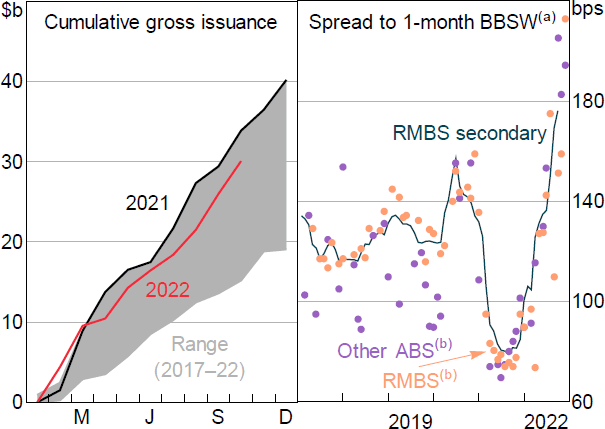
Notes:
(a) AAA-rated notes of prime residential mortgage-backed securities (RMBS) and other ABS, spread to 1-month bank bill swap rates (BBSW).
(b) Face-value weighted monthly average.
Sources: Bloomberg; Intercontinental Exchange, Inc.; KangaNews; RBA
This paper attempts to estimate the causal effect of the TFF on the amount of banks' lending to businesses. The facility may have supported lending by providing banks with an assured source of funding, by reducing their funding costs, and/or by providing an incentive to increase lending so as to increase their additional allowances. We investigate the effect of the TFF on business lending through these three channels. The first set of our regressions aims to identify only the effect of the additional allowance on credit supply to businesses. In subsequent regressions, we aim to capture the broader range of channels, including the effect of the TFF on lending volumes via lowering banks' funding costs and increasing funding certainty. In these latter regressions, we first estimate the overall effect on business lending of the TFF being available to banks, and then estimate the effect on those banks accessing the facility. In all regressions, we use a difference-in-differences approach. The identification strategies rest on assumptions about the relationship between different types of lending in the absence of the TFF or in the absence of the additional allowance feature. We clearly state these assumptions, along with why they may not hold, at the relevant parts of the paper. We also aim to increase the robustness of the results using additional control variables, instrumental variables, and a triple-difference framework. To the extent that these strategies do not fully address the identification challenges, it is possible that our results understate the effect of the TFF on business lending.
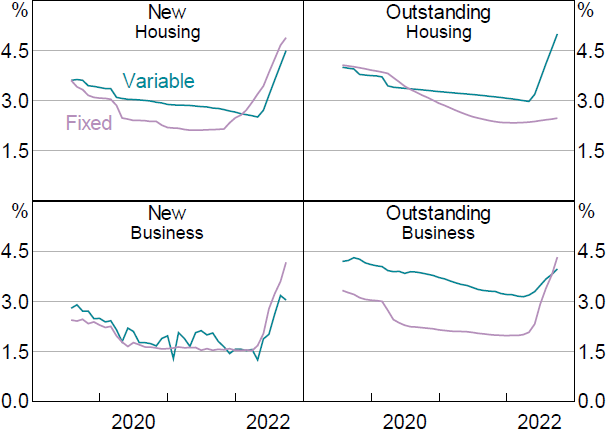
Sources: APRA; RBA
The questions addressed in this paper have important policy implications for central banks. Term funding schemes have been introduced in many countries in the past two decades, with several of these including incentives to encourage specific types of lending, typically to businesses. A wider range of central banks introduced or expanded term funding schemes in response to the COVID-19 pandemic. Most of the existing literature on central bank term funding schemes relates to the European Central Bank's (ECB's) targeted longer-term refinancing operations (TLTROs), which have been found to be generally effective in promoting increased lending to businesses. Literature on other central bank funding schemes remains scarce. This paper seeks to contribute to this literature, building our understanding of term funding schemes and their potential to encourage the provision of credit. This body of evidence, including this paper, is likely to be useful as central banks consider the design of future term funding schemes, should they be pursued.
This paper does not provide a detailed assessment of all aspects of the TFF. As mentioned, other work has described how the facility provided banks with funding certainty and contributed to lower funding costs for banks, which flowed through to lower interest rates for households and businesses (Black, Jackman and Schwartz 2021). So it is important to emphasise that this paper is focused only on the effect of the TFF on the quantity of bank lending to businesses, including SMEs.
This paper is structured as follows. Section 2 describes the TFF in detail, before discussing take-up of the facility and trends in business lending. Section 3 describes the various term funding schemes used by other central banks and the evidence for their effect on lending. Section 4 describes the lender-level data used, while Section 5 explains the identification strategies and challenges. Sections 6, 7 and 8 outline the regressions and results. Section 9 concludes and provides a discussion.
2. Policy Design and Background
2.1 Overview of the TFF
The TFF was available to all authorised deposit-taking institutions (‘banks’) that extend credit. This included more than 130 Australian banks, credit unions and building societies, as well as foreign bank branches and subsidiaries operating in Australia. The facility enabled these institutions to access three-year funding at a fixed rate that was well below their cost of wholesale market funding at the same term. The value of funding available was sizeable, ensuring that the facility had a sizeable influence on participating banks' cost of funds.
The price and amount of funding varied throughout the pandemic. The TFF initially gave banks access to three-year funding at a cost of 0.25 per cent, with:
- an initial allowance equivalent to 3 per cent of each bank's total credit outstanding;[2] banks could access their initial allowance until 30 September 2020.
- an additional allowance, which was initially available until 31 March 2021 to any bank that expanded its business credit, with greater incentives for lending to SMEs. Banks were granted one dollar of additional allowance for every dollar increase in the stock of lending to large businesses, and five dollars of additional allowance for every dollar increase in lending to SMEs. Banks that reported a decline in SME or large business credit received no additional allowance for that category.
The RBA subsequently made a number of adjustments to the TFF in response to changes in economic and financial conditions:
- In September 2020, the TFF was expanded with a new supplementary allowance for each bank equivalent to 2 per cent of its credit outstanding, available to be drawn between 1 October 2020 and 30 June 2021. Also, the period for drawdowns of the additional allowance was extended by three months to 30 June 2021.
- In November 2020, the cost of new funding under the TFF was lowered to 0.1 per cent in line with reductions in the cash rate target and 3-year government bond yield target.
The price of the TFF was set at a low level to ensure it was attractive to banks. Initially set at 25 basis points and then reduced to 10 basis points in November 2020, the TFF was much cheaper than 3-year wholesale funding for major banks throughout the drawdown period (Figure 3). The cost differential was even greater for non-major banks, who typically have higher costs of funding due to lower credit ratings.
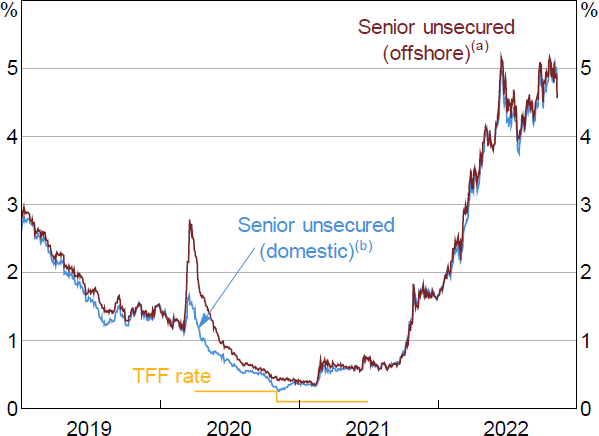
Notes:
(a) Adjusted for cross-currency hedging.
(b) Covered bonds trade at yields on average about 5 basis points below senior unsecured (domestic) bonds since 2019, but this has varied over time. Through 2020 and 2021, this difference fell to 1 basis point on average, but ranged between 14 basis points above and 40 basis points below senior unsecured (domestic) bonds.
Sources: Bloomberg; RBA
To minimise risk to the RBA, banks were required to pledge high-quality collateral to access the TFF, in line with the RBA's collateral policy.[3] The most attractive collateral for banks to use were self-securitisations, which are notes backed by loans already on the banks' balance sheets. By pledging self-securitisations, banks could avoid the need to fund the acquisition of other collateral. Indeed, self-securitisations constituted over 90 per cent of collateral pledged for the TFF by value.
2.2 The additional allowance
The additional allowance, an area of focus for this paper, was designed with the explicit aim of encouraging banks to increase lending to businesses, particularly SMEs. The additional allowance was updated monthly throughout the TFF drawdown period. The final value of the additional allowance was calculated as the sum of:
- One times the dollar increase in large business credit outstanding from the three months ending 31 January 2020 through to the three months ending 30 April 2021 (if there is a decline in large business credit outstanding, then this is zero).
-
The larger of:
- five times the dollar increase in SME credit outstanding from the three months ending 31 January 2020 through to the three months ending 30 April 2021 (if there is a decline in SME credit outstanding, then this is zero).
- five times the dollar increase in SME credit outstanding from 29 February 2020 to the three months ending 30 April 2021 (if there is a decline in SME credit outstanding, then this is zero).
The final value of each bank's additional allowance was determined after the end of April 2021, using the average of the most recent three months of data in the calculations above, and could be drawn until the end of June 2021.[4]
2.3 TFF take-up and business lending through the pandemic
The TFF was announced in March 2020 and was available to access in early April. TFF drawdowns increased only gradually, ramping up in advance of the first deadline of end September 2020. Similarly, drawdowns again increased in the lead-up to the final deadline of end June 2021. By drawing on the TFF close to the deadline dates, banks could push back the time the debt matured.
In aggregate, banks drew down 88 per cent of the total funding available from the facility (Figure 4). Overall, 92 banks accessed the TFF – 41 banks were eligible but did not access the facility, although these lenders accounted for less than 5 per cent of allowances by value.
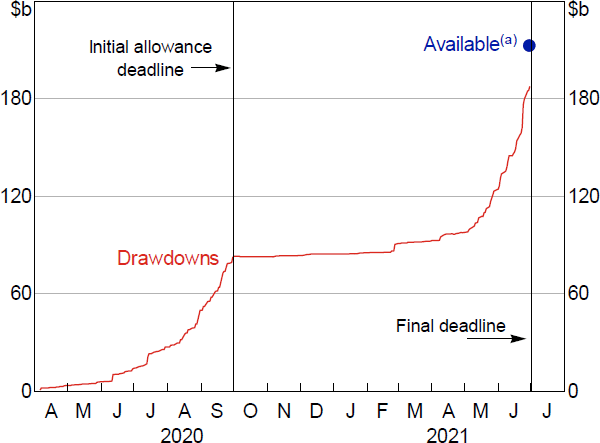
Note: (a) Includes allowances for eligible banks who did not access the facility.
Sources: APRA; RBA
The size of additional allowances varied over time, reflecting changes in each bank's business credit outstanding. Starting in March 2020, businesses turned to banks for access to liquidity (Kohler 2021; Johnson, Lane and McClure 2022). Facing a period of uncertainty and a potential decline in cash flow, some businesses drew down existing lines of credit and some sought higher credit limits as a precaution. These firms generally repaid these facilities within a couple of months. Total lending to businesses was approximately steady for much of the rest of the TFF drawdown period, although aggregate lending to large businesses increased in mid-2021 as economic conditions improved. Meanwhile, aggregate lending to SMEs was little changed. Nevertheless, additional allowances increased over the last few months of the TFF, driven by a number of banks that increased their business lending, particularly to SMEs. Consistent with this, the bulk of additional allowances available in June 2021 were accrued due to increases in SME lending by some banks.
Since SME lending was little changed throughout the pandemic, it is likely those banks that increased their business lending, and hence gained an additional allowance, did so by winning customers from other banks. SME loan refinancing activity was higher in 2020/21 compared with the year prior, despite the stock of SME lending being little changed over the drawdown period. This activity would not necessarily lead to an increase in aggregate business lending, although this refinancing activity is likely to have benefited SMEs, which could have achieved lower interest costs or improved lending terms.
In aggregate, businesses' demand for debt was weak throughout 2020 and early 2021. Business appetite to borrow was likely suppressed by the weakness in economic activity and a heightened level of uncertainty around the outlook (Black, Lane and Nunn 2021). At the same time, businesses received sizeable government support via initiatives such as JobKeeper, which boosted their cash flow, reducing the need for credit. Survey data and liaison with businesses suggested that SMEs, in particular, had little appetite for taking out new loans. In part, this reflected ongoing uncertainty about the economic outlook, with SMEs disproportionately affected by the COVID-19 pandemic as they were more likely to operate in industries harder hit by lockdowns. These factors pose challenges to identifying the effect of the additional allowance on SME lending, as described in Section 5.
As at the end of June 2021, the final value of available additional allowances was around 7 per cent of business credit, or 34 per cent of total TFF allowances (Figure 5). The banks that had access to additional allowances generated $26 billion in new lending to large businesses and $9 billion to SMEs.
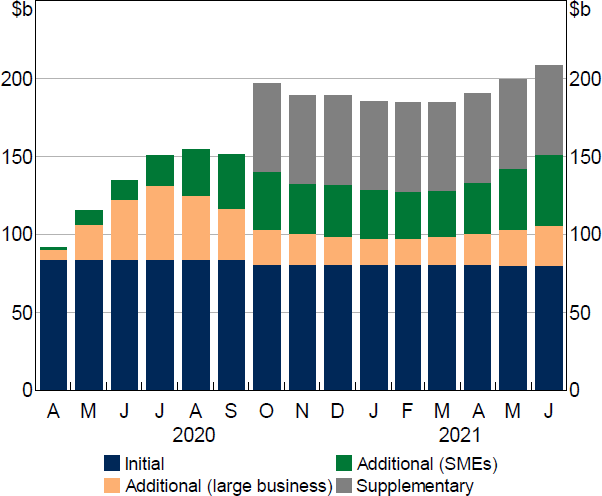
Notes: Total TFF allowances for 133 eligible banks as at the end of each month. In contrast to the initial and supplementary allowances, the additional allowance varied over the life of the TFF, depending on each bank's increase in lending to businesses.
Sources: APRA; RBA
3. The International Context
3.1 Other central bank term funding schemes
A number of other central banks also introduced term funding schemes in response to challenges arising from the outbreak of the COVID-19 pandemic, including the Reserve Bank of New Zealand (RBNZ), the Monetary Authority of Singapore (MAS), and the Sveriges Riksbank.[5] Additionally, some other schemes have been operating for around 10 years or more, including those introduced by the Bank of Japan (BoJ), the ECB and the Bank of England (BoE) as they sought to impart further stimulus at a time when policy rates were near the effective lower bound and the supply of credit had contracted.
Term funding schemes implemented by other central banks have varied in their design. Low-cost funding is a common feature across all term funding schemes, and the price is often tied to a policy rate. The overall price of the scheme may also incorporate additional fees or discounts that vary depending on certain criteria, and, in some cases, the price has been used to incentivise banks to direct lending to particular sectors of the economy. The BoE, ECB and Riksbank introduced schemes in which the cost of funds available to banks has varied depending on their volume of lending to the private sector.
Banks' allowances under the scheme is another parameter that varied across schemes. Similar to the TFF, some schemes offered banks a fixed initial borrowing allowance based on their total credit outstanding, plus an additional allowance that is contingent on their volume of new lending during a particular period. Other schemes offered more targeted credit support by providing an allowance solely dependent on the volume of a particular type of lending, and banks could only draw on the facility by posting these loans as collateral. In contrast, in the Riksbank's onward lending program banks did not have individual allocations. Instead, banks would bid for funds at a weekly auction until all funds were exhausted.
Since the onset of COVID-19, several term funding schemes made favourable lending conditions contingent on the volume of lending to SMEs, similar to the TFF. Such schemes were introduced by the BoE, Banco de México, and the BoJ. Other schemes have directly complemented government loan programs by linking funding allowances to the use of these programs. This included the US Federal Reserve's liquidity facility, which extended credit to facilities that originated loans under the government's Paycheck Protection Program, with the loans to be used as collateral. The RBNZ and the MAS introduced similar programs where loan sizes depended on the volume of lending under a government loan program.
Many term funding schemes required the provision of collateral by the banks to mitigate financial risks to the central bank. Often, collateral requirements were aligned with a central bank's eligible collateral policy for repo funding through open market operations. Some central banks expanded their collateral requirements as part of their wider COVID-19 monetary policy measures, such as the ECB and the BoE. For example, some central banks accepted a wider range of collateral for these facilities than typically accepted for open market operations. This included the TFF which, as discussed above, allowed banks to post self-securitisation notes for funding (avoiding a drain on their liquid assets or the need to borrow to purchase eligible collateral). As noted above, other central banks allowed banks to post certain types of loans as collateral, such as those made through government loan programs or made to select sectors.
3.2 Literature
This paper contributes to the growing literature studying the effect of term funding schemes implemented around the world and, to the best of our knowledge, is the first empirical study of the effect of the RBA's TFF on bank credit supply in Australia. Few studies to date have examined the effects of central bank term funding schemes throughout the pandemic. In a comprehensive examination of euro area monetary and prudential policies implemented during the pandemic, Altavilla et al (2020) concluded that lending to firms would have been 3 percentage points lower in 2020/21 – 2021/22 in the absence of the ECB's third series of the TLTRO (TLTRO III).[6] Da Silva et al (2021) also found significant positive effects on credit supply from TLTRO III in the euro area. A couple of studies have examined the effects of policies within countries. For example, in a study using Austrian bank-level data, Kwapil and Rieder (2021) found a positive effect of TLTRO III on Austrian banks' loan supply between July and September 2020.
The US Federal Reserve implemented several targeted lending programs in response to the pandemic, including the Main Street Lending Program (MSLP) and the Paycheck Protection Program Liquidity Facility (PPPLF). Minoiu, Zarutskie and Zlate (2021) found that, while overall take-up was limited, bank participation in the MSLP was associated with relatively less tightening of commercial and industrial lending standards than non-participating banks. Additionally, participating banks were more likely to increase business lending. Anbil, Carlson and Styczynski (2021) found that banks that used the PPPLF extended over twice as many paycheck protection program loans compared to non-participating banks.
Notwithstanding the limited literature on central bank term funding schemes during the pandemic, several papers have examined the effect on banks' lending of the first and second series of the ECB's TLTROs, introduced in 2014 and 2016, respectively. The literature generally found that the first two TLTRO series were effective in increasing loan supply or decreasing interest rates for participating institutions relative to non-participating institutions (e.g. Afonso and Sousa-Leite 2020; Esposito, Fantino and Sung 2020; Andreeva and García-Posada 2021; Benetton and Fantino 2021; Laine 2021). Many of these papers employed difference-in-differences approaches to compare the behaviour of participating and non-participating institutions. These studies also generally used instrumental variables to account for endogeneity issues in the self-selection of banks into accessing central bank funding. Some papers examined both the extensive (i.e. participation in the TLTRO relative to non-participation) and intensive margin effects (i.e. the amount of funding), with mixed results as to the relative importance of these effects.
Literature on the effects of other central banks' funding schemes remains sparse.[7] Examining the BoE's Funding for Lending Scheme, Havrylchyk (2016) found no effect of sharpened incentives for lending to SMEs on SME credit growth relative to large businesses. Havrylchyk investigated the effectiveness of the incentive mechanisms in the scheme for banks to expand their supply of lending to SMEs by exploiting the BoE's 2013 amendments to the scheme's rules. When the Funding for Lending Scheme was first implemented in 2012, banks' additional allowances were set such that a £1 increase in net lending would grant a bank £1 of additional allowance. However, from April 2013 to 2014, the BoE amended this allowance so that, for every £1 of net lending to SMEs, banks were granted £10 of additional allowance.
3.2.1 Identification challenges in the literature
Empirical papers assessing the effect of term funding schemes on credit face a few common identification challenges. Firstly, banks could decide whether or not to access central bank funding. The self-selection of banks into receiving funding raises an endogeneity issue as banks with certain characteristics (for example, those who would have increased lending anyway) may be more likely to participate than others. Empirical papers often address this issue through an instrumental variable strategy. Various papers studying the effect of the ECB's TLTROs on credit supply used banks' predetermined borrowing allowances or usage in previous funding series as an instrument for TLTRO uptake (including Andreeva and García-Posada (2021); Benetton and Fantino (2021); Kwapil and Rieder (2021) and Laine (2021)). The rationale typically provided for this instrument is that borrowing allowances are set by the ECB and based on the stock of loans prior to the period being studied. For the US Federal Reserve's facility, Minoiu et al (2021) used proxies of bank familiarity with Federal Reserve procedures and facilities to isolate exogenous variation in participation.
Many of these papers found that this self-selection issue would bias the coefficients in an unclear direction; OLS estimates may be biased upwards if banks that accessed the facility would have increased their lending anyway. In contrast, the coefficients may be biased downward if banks that chose to borrow were experiencing worse unobservable funding problems, and therefore may have granted fewer new loans.
The second key challenge is disentangling the effects of credit supply and credit demand. Studies have commonly dealt with this challenge by adding fixed effects for the borrowing firms. In particular, several papers used the Khwaja and Mian (2008) method, which exploits the fact that many firms simultaneously borrow from several banks (Esposito et al 2020; Benetton and Fantino 2021; Minoiu et al 2021). This allows for a comparison of credit growth across different lenders for the same firm. However, this method relies on access to data covering firms that had loans from multiples lenders. Without access to these types of data, Kwapil and Rieder (2021) utilised a bank-specific covariate which takes into account each bank's lending composition and the effect of the COVID-19 pandemic on each sector. Laine (2021) employed a different approach, using country–time fixed effects and adding housing loans as a control variable.
Our paper contributes to the literature by assessing the effects of the RBA's TFF on the amount of business lending. As noted earlier, empirical papers on the effects of central bank term funding schemes during the pandemic remain limited. Furthermore, while some central bank funding schemes have included additional incentives to promote lending to particular groups such as SMEs, very few papers have examined the effects of these specific incentives. Unlike the bulk of existing literature, we specifically examine whether the additional incentives for SME lending provided in the RBA's TFF (in the form of higher funding allowances) were effective in stimulating increased lending to this sector. Our analysis contributes to our understanding of central bank funding schemes and their potential to encourage increased lending.
4. Data
The analysis in this paper primarily draws on confidential data reported to the Australian Prudential Regulation Authority (APRA) by banks (authorised deposit-taking institutions) and non-banks (finance providers that do not accept deposits, including finance companies and money market corporations). We primarily use data collected under APRA's Economic and Financial Statistics (EFS) collection. The EFS collection has been available since mid-2019, and has provided comprehensive and accurate data on financial institutions and their lending and borrowing activities. In particular, these data are accompanied by comprehensive guidance on data definitions, and are subject to standards for data quality and audit obligations.[8] Most of the lending data used to calculate the TFF's initial, supplementary and additional allowances were based on data from the EFS collection. Further detail on our data is set out in Appendix A.
For our first analysis comparing SME and large business, we draw on monthly EFS data covering business credit by firm size, industry and loan type. These data are reported by banks and non-banks that have more than $2 billion of business credit; this threshold is estimated to capture over 95 per cent of total business credit. We supplement this with data reported directly to the RBA for the purposes of calculating the TFF additional allowance.
For our analysis comparing the effects of the availability and take-up of the TFF, we draw on EFS data covering loans and leases. These data are reported monthly by most banks, as well as non-banks with more than $400 million in total assets. Aside from data reported to APRA through the EFS forms, we use RBA data on banks' self-securitised assets.
5. Identification Strategies, Challenges and Assumptions
5.1 Identification strategies
This paper attempts to estimate the causal effect of the TFF on the amount of banks' lending to businesses. We do so by focusing on three key policy questions. First, we examine whether the incentive in the TFF's additional allowance was effective in stimulating growth in business credit. Second, we investigate whether the availability of the TFF affected a bank's business lending. Finally, we assess whether a lender's take-up of the TFF affected their business lending.
Our analysis is performed in a differences-in-differences set-up, which seeks to estimate the causal effect of an intervention by comparing changes in outcomes between a ‘treatment’ and a ‘control’ group. In our first analysis, where we examine whether the additional allowance feature was effective in stimulating growth in business credit, we exploit the fact that banks received five times the additional allowance for lending to SMEs relative to large businesses, with large businesses acting as the control group in this exercise. Since these regressions focus on the difference between lending to SMEs and large businesses, they are designed to capture only the effect of the incentive provided by the additional allowance. The subsequent analysis captures the broader range of channels, including the additional allowance as well as the effect of the TFF on banks' funding costs and their certainty of funding.[9] We assess the effect of the availability of the TFF on aggregate business lending by examining the difference in business lending growth for banks relative to non-banks. Non-banks are the control group in this exercise as they were not eligible for the TFF. Finally, we aim to estimate the effect of banks accessing the TFF, as distinct from the effect of its availability. To address this question, we use instrumental variables to avoid endogeneity issues.
In our analysis, we face a number of identification challenges, which we address in a variety of ways, including additional control variables, instrumental variables and a triple-difference framework. As discussed above, one identification challenge common with other international literature is disentangling the effects of credit supply and credit demand. Liaison with banks and other data sources indicated that demand for business credit was generally weak from the beginning of the pandemic through to early 2021, reflecting the reluctance of firms to take on credit in an environment of economic uncertainty. In addition, the provision of government support such as JobKeeper provided businesses with substantial liquidity meaning that they had less need to access credit.[10] For our baseline model comparing growth in credit outstanding to SMEs and large businesses, an identification issue would arise if credit outstanding to SMEs would have evolved differently to that of large businesses in the absence of the TFF. For example, SMEs were particularly targeted by various government policy support measures, which may have reduced the need for finance. Larger businesses may have been more willing to borrow, including by drawing on lines of credit for precautionary reasons. While international papers have commonly dealt with this issue by adding fixed effects for the borrowing firms, we are not able to conduct similar analysis as we do not have access to data on individual borrowers.
We use a regression in the spirit of a triple-difference framework to address the potential difference in the effect of COVID-19 on lending to SMEs and larger businesses. This regression assesses the difference between lending to SMEs and large businesses by banks relative to non-banks. Under this model, we assume that if COVID-19 restrictions did have different effects on SME and large business lending, including their demand for debt, then these differences would be common between the business customers of banks and non-banks.
In addition, COVID-19 restrictions had the largest effects on industries such as accommodation, hospitality and tourism, which tend to have a larger share of small firms. Small businesses have generally always found it more difficult to access finance than large businesses and (all else equal), these difficulties might have been expected to worsen during the pandemic (Kent 2021a). We address this issue by using data at the bank and industry level. This allows us to control for the uneven effects of COVID-19 across industries by examining whether the TFF had a differential effect on SME lending compared to large business lending within industries. The triple-difference regression may also address this issue, so long as this difference in industries between small and large firms was common across banks and non-banks.
Closely related to these challenges, data and bank liaison suggest that, in the early months of the COVID-19 pandemic, large businesses drew down on existing lines of credit for precautionary reasons. This resulted in a sharp increase in business credit, which subsequently reversed as these businesses generally repaid these facilities within a couple of months. As these moves in business credit were driven by large businesses, and generally did not have an effect on banks' final additional allowance allocation, these developments may suggest higher large business credit growth by banks than what would otherwise be expected in an ‘ideal’ counterfactual scenario. We attempt to address this issue by using only fixed-term loans, which abstracts from businesses drawing on their existing revolving credit facilities.
In addition to complications related to lending to SMEs and large businesses, other identification challenges are present in the regressions that estimate the effect of the availability of the TFF to banks. In particular, non-banks may not be an ideal control group for banks when comparing business credit growth. A range of factors affect competition between banks and non-banks. While non-banks were not able to access the TFF, they did benefit from the Australian Office of Financial Management's (AOFM's) Structured Finance Support Fund, which improved the certainty of funding and reduced funding costs. In addition, non-banks benefited from less bond issuance by banks, which helped to narrow spreads on ABS. Working in the other direction, non-banks were unable to benefit from the strong pace of growth in deposits during the pandemic. Non-banks' business customers may have also been affected by the pandemic differently to firms that borrow from banks, including because non-banks may lend to a different population of borrowers than banks. It is likely that, in net terms, the effect of the Structured Finance Support Fund and low cost of securitisation issuance would support non-banks' business lending relative to banks' lending. This may suggest higher business credit growth by non-banks than what would otherwise be expected in an ‘ideal’ counterfactual scenario, causing the effects of the TFF on bank lending to be understated.
Another challenge that is common across the international literature is that participation in the TFF was voluntary. It is possible that banks who accessed TFF funding may have increased lending anyway (or the reverse). This identification issue is particularly relevant for our third analysis, which seeks to identify a differential effect between banks that did or did not access the TFF. Similar to international literature, we address this issue through an instrumental variables strategy.
Our first choice of instrument is the availability of self-securitised assets prior to the TFF. A valid instrument should be strongly correlated with a bank's decision to participate in the TFF but have no direct effect on business credit growth during the pandemic (except through its effect on influencing TFF participation). As noted above, banks with self-securitisations could access the TFF at lower cost, since they did not need to fund the acquisition of collateral (or establish processes to create self-securitisations). As such, banks with self-securitised assets were much more likely to access the TFF, suggesting the first condition holds (we test this formally below). The second condition is generally untestable, and this identification strategy will be problematic if the availability of self-securitisations is correlated with banks' business lending through channels other than access to the TFF. As an example, smaller banks and foreign banks are less likely to have self-securitised assets than larger Australian banks. If small or foreign banks' business lending did respond to the pandemic in a different way to that of large Australian banks, this would pose a challenge to this identification strategy.
In our second instrumental variable regression, we use the status of lenders as banks or non-banks as an instrument for whether an institution participated in the TFF. Since only banks were eligible for the scheme, we use this to estimate the effect of accessing the TFF on lending. The effects of accessing the scheme should be larger than the effect of it being available, since not all banks drew on the facility.
Finally, there are some very large growth rates in our sample as some lenders had very low levels of credit in the base period. This is an issue in the analysis comparing SMEs and large businesses, as some SME lending in particular can be quite volatile, suggesting that some smaller banks may drive the results. For our analysis comparing SMEs and large businesses, we address this by dropping three outliers (two banks and one non-bank). We also check our results by weighting the regressions based on the level of each lender's credit outstanding in the base period.
5.2 Parallel trends assumption
A difference-in-differences framework requires a ‘parallel trends assumption’ between the treatment and control groups, such that outcomes in the control group are an appropriate counterfactual for how outcomes in the treatment group would have evolved without the intervention. While we endeavour to create suitable control groups, identification is challenging because these assumptions may be invalidated by factors that are not observable. This is especially pertinent as the introduction of the TFF coincided with significant shocks resulting from the pandemic and other policy interventions.
Although imperfect, one way to examine whether outcomes in our control groups are an appropriate counterfactual is to visually inspect the trends in the lead-up to the TFF. SME and large business loan growth appears to be broadly correlated in the few months immediately prior to the TFF introduction, as measured by the simple average of bank-level growth rates (Figure 6). The pre-TFF trends are also similar for aggregate business credit extended by banks and non-banks (Figure 7). A statistical test of our pre-trends period for SME and large business lending growth, and banks relative to non-banks, supports this assumption.[11]
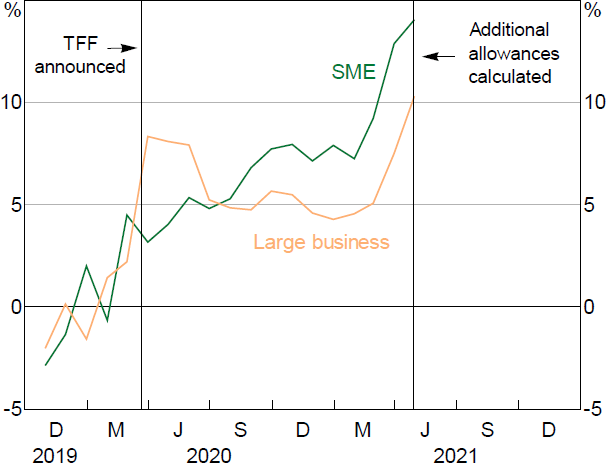
Note: Unweighted average of the cumulative growth in business credit relative to the average of the three months to January 2020 for banks that report data on business lending by business size; excludes two outliers.
Sources: APRA; RBA
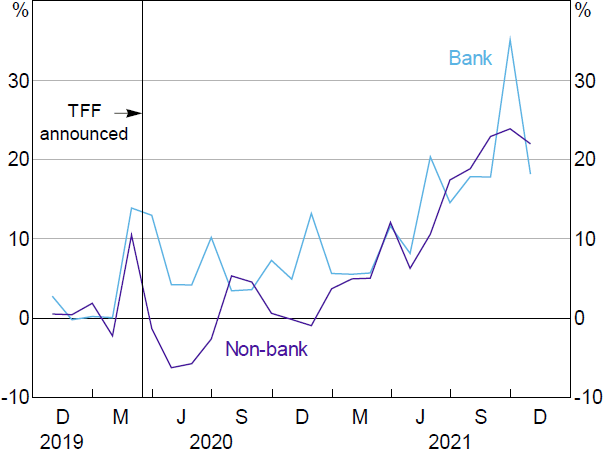
Note: Unweighted average of the cumulative growth in business credit relative to the average of the three months to January 2020 for institutions reporting on ARF 720_1 A/B; excludes institutions that don't have data for all months.
Sources: APRA; RBA
The triple-difference regression considers the differing trends between lending to SMEs and to large businesses, comparing this difference between banks and non-banks. This regression assumes that this difference, SME lending less large business lending, would have evolved similarly for banks and non-banks in the absence of the TFF. However, prior to the pandemic, this differential was increasing for non-banks relative to banks, which could suggest a weakness in this analysis (Figure 8). In part, this could be due to the smaller sample size of non-banks which report outstanding credit by business size.
Our instrumental variable analysis implicitly assumes that total business credit growth would have evolved similarly for banks that had self-securitised assets and those that did not. These data are volatile, although a statistical test fails to reject the null hypothesis that these pre-trends were the same (Figure 9).
In general, the pre-trends period should be interpreted with caution because the EFS data collection only began in mid-2019 and there may have been data quality issues during the first few months of reporting.[12] In addition, we use non-seasonally adjusted data so month-to-month variations may reflect seasonality.
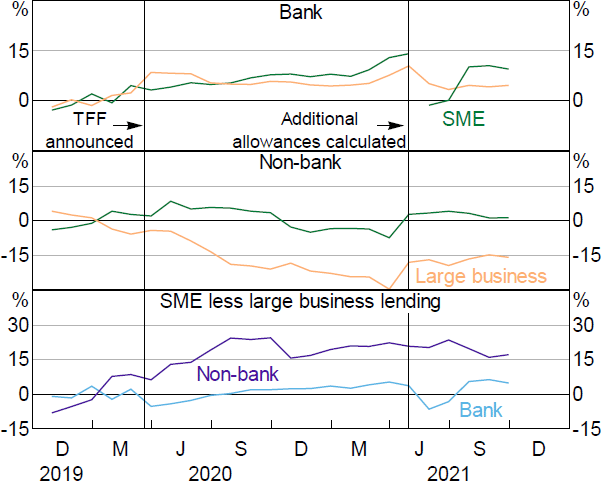
Note: Unweighted average of the cumulative growth in business credit relative to the average of the three months to January 2020 for institutions reporting business lending by business size; excludes three outliers.
Sources: APRA; RBA
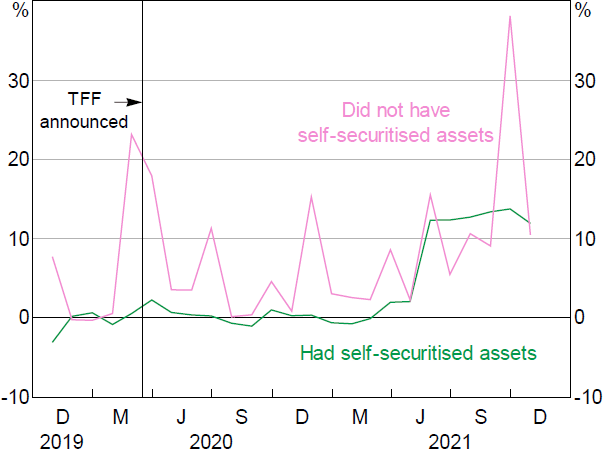
Note: Unweighted average of the cumulative growth in business credit relative to the average of the three months to January 2020 for banks reporting on ARF 720_1 A/B; excludes institutions that don't have data for all months.
Sources: APRA; RBA
6. Was the Additional Allowance Feature Effective?
6.1 Model specification
By exploiting the fact that banks received five times the additional allowance for lending to SMEs relative to large businesses, we examine whether the TFF was effective in stimulating growth in business credit. These regressions aim to identify only the effect of the additional allowance, without reflecting the effects of the TFF on lending via reducing funding costs and increasing funding certainty.
For our analysis examining the effect of the TFF on SME credit growth, we run our baseline specification for each month in the sample:
The dependent variable is the cumulative growth in business credit by bank i to borrower type j in month t relative to a pre-TFF period (average of the three months to January 2020). Borrowerj is a dummy variable equal to 1 for SMEs and 0 for large businesses. The coefficient represents our treatment effect. This approach is approximately equivalent to a panel regression with time-specific coefficients on the borrower type, and fixed effects for the pre- and post-TFF periods.[13]
We run a version of our baseline regression using fixed-term lending as the dependent variable, although this uses a smaller sample of banks. As a ‘placebo test’, we also run a version of the baseline model for small business lending relative to medium business lending.[14]
In order to control for the uneven effects of COVID-19 across industries, we run versions of these models at the bank-industry level. The bank-industry level model specification is similar to the baseline model, with data organised at the bank i and industry k level and the inclusion of a bank × industry fixed effect :
This specification absorbs the effects of any shocks that affect an entire industry (e.g. retail), or a particular institution, or even shocks that affect the lending of a particular institution to a particular industry. As such, this specification is more robust to the potential violations of the parallel trends assumption discussed above.
There are several small institutions in our sample that may be driving our results, particularly those that experienced growth in business credit off a very low base. We address this by removing two outliers (these banks did not access the TFF). As a further check, we weight the regressions for the baseline model and the industry-level model by each institution's level of business credit in the base period. We also run weighted regressions that have been limited to just fixed-term lending.
We explore if the effects of the TFF differed depending on bank characteristics. We add the bank characteristic, Characteristicn , and the characteristic interacted with the SME borrower dummy, one at a time into our baseline specification:
This specification uses a smaller sample size of banks than our baseline regression, given the available data on bank characteristics.
We also take a standard difference-in-difference model and add bank and period fixed effects, and , respectively:
Given the potential issues around the validity of using large business lending as a control group for SME business lending, we run a model in the spirit of a triple-difference regression examining lending to SMEs relative to large businesses and examining lending by banks relative to non-banks. The specification is:
Here, the coefficient of interest is y4. This coefficient reflects the effect of the TFF on the difference between SME and large business lending for banks relative to non-banks. The dependent variable is the cumulative growth in business credit by bank i of institution type h to borrower type j in month t relative to a pre-TFF period (average of the three months to January 2020), where institution_typeh is a dummy variable equal to 1 for banks and 0 for non-banks. This specification uses a larger sample than the specifications above, with non-banks included.
6.2 Results
For most of our models, estimation is between October 2019 and October 2021, with the sample varying depending on the model specification and data used. By running a separate regression for each month in our sample, we are able to estimate a TFF effect for each period rather than constraining the effects to be constant over time. Standard errors are clustered at the institution level. All results tables can be found in Appendix C.
In our baseline model (Equation (1)), we find no statistically significant effects of the TFF on credit growth to SMEs relative to large businesses for all time periods following the introduction of the TFF up until additional allowances were calculated in April 2021 (Table C1).[15] The coefficients for our baseline model represent the difference in cumulative growth, relative to the pre-TFF period, between credit outstanding to SMEs and large businesses. For example, taken at face value, the coefficient for April 2021 suggests that the TFF caused cumulative growth in SME credit to be 3.7 percentage points higher than for large businesses (Figure 10). However, the confidence intervals are wide.
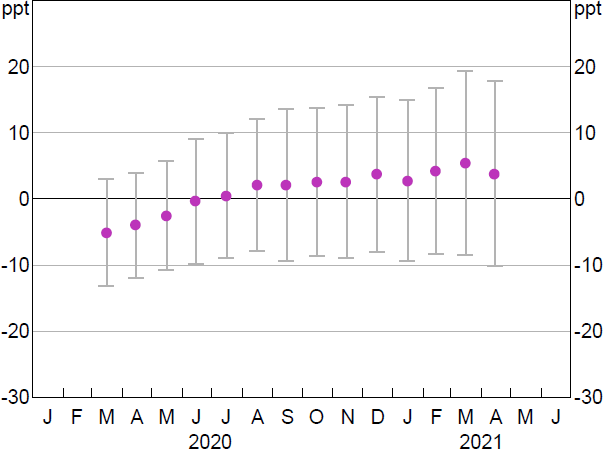
Note: Whiskers show 95 per cent confidence intervals.
Sources: APRA; Authors' calculations; RBA
The coefficients are negative in early 2020, indicating that credit increased for large businesses relative to SMEs. This reflects that large businesses drew down on lines of credit as the pandemic intensified. Limiting the baseline model to fixed-term lending causes the coefficients to be higher in early 2020. However, there are no statistically significant effects of the TFF on fixed-term credit growth to SMEs relative to large businesses for all time periods following the introduction of the TFF (Figure B1; Table C2).
In contrast to what we would expect, our ‘placebo test’ – which is a version of our baseline model for lending to small businesses relative to medium businesses – generates significant and negative coefficients on the small business borrower dummy for the most recent few months in our sample (Figure B2; Table C3). This negative correlation between business size and lending is consistent with the perverse negative coefficient estimates we find in our baseline models; it suggests that lending to large firms is not a good counterfactual for lending to smaller firms during the period being studied.
The industry-level model (which, as discussed above, is intended to control for the uneven effects of COVID-19 by industry; Equation (2)) finds no statistically significant effects for most time periods, although there are some statistically significant, negative coefficients in the earlier months following the TFF introduction (Figure B3; Table C4). This is the opposite sign to what we would expect a priori. Our preferred specification has an interaction between bank and industry dummies, which allows us to examine the difference in SME and large business credit growth for a particular bank within a particular industry. As a crosscheck, we also run variations of the model with only bank-level fixed effects and only industry-level fixed effects (Figures B4 and B5; Tables C5 and C6). The results of these model variations are consistent with our preferred specification, with statistically non-significant effects for most time periods, except the earlier months of the pandemic where large negative significant coefficients are recorded on the SME borrower treatment variable. These were the months in which large businesses drew down on lines of credit for precautionary reasons.
In the regressions that are weighted by each institution's level of business credit in the base period, the coefficients on the SME borrower term are negative and statistically significant in multiple time periods for both the baseline model specification and the industry-level model (Figures B6 and B7; Tables C7 and C8). This is unsurprising given that, in aggregate, SME business credit growth has generally been weaker than large business credit growth, particularly in the early months of the COVID-19 pandemic as large businesses drew down on lines of credit to shore up liquidity positions. For our weighted regressions that have been limited to just fixed-term lending, the coefficients on the SME borrower term are negative and statistically significant in multiple time periods (Figure B8; Table C9).
We also explore whether the effects of the TFF differed depending on bank characteristics (as at December 2019, prior to the TFF; Equation (3)). Some of these regressions have the full sample of up to 50 banks, but three regressions – those using the common equity tier 1 capital ratio, total tier 1 capital ratio and tier 1 leverage ratio – include only 14 banks. For simplicity, we run the analysis for just one time period (April 2021) and add the bank characteristics (the characteristic itself and the characteristic interacted with the SME borrower dummy) one at a time into our baseline specification. These models present statistically insignificant results for almost all model variations (Table C10). However, we find a statistically significant and positive coefficient for the interaction between capital ratios and SME lending. At face value, this suggests that banks with higher capital ratios prior to the TFF experienced stronger cumulative growth in lending to SMEs relative to large businesses in April 2021, though the results should be interpreted with caution given the small sample size of this specification.
Our standard difference-in-difference model with bank and period fixed effects (Equation (4)) generates a negative and not statistically significant coefficient on the interaction term (Table C11). This aligns with our baseline model, which also indicates that there was no statistically significant effect of the TFF credit growth to SMEs relative to large businesses.
In the triple-difference model (Equation (5)), the coefficient on the interaction term reflects the effect of the TFF on banks' lending to SMEs less large businesses, relative to non-banks. We would expect the gap between growth in SME and large business lending to be larger for banks, relative to non-banks. The coefficient on the interaction term is negative and weakly statistically significant, suggesting the opposite result: the difference between SME and large business lending decreased for banks, relative to non-banks (Figure 11; Table C12). However, large and increasing confidence intervals suggest that our estimates are too imprecise to determine whether this trend holds over the whole sample.
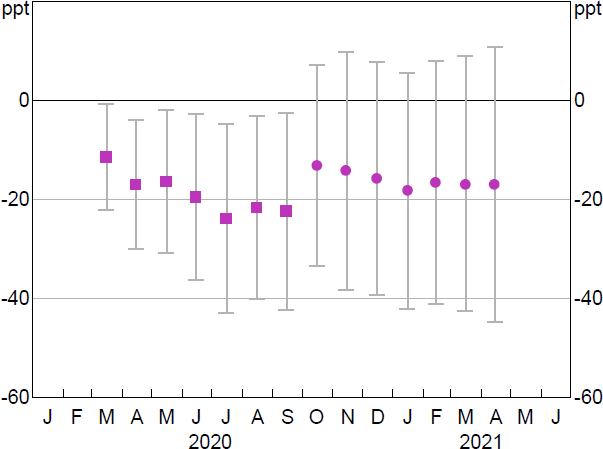
Notes: Whiskers show 95 per cent confidence intervals. The filled square marker indicates a significant result.
Sources: APRA; Authors' calculations; RBA
7. What Was the Effect of the Availability of the TFF on Business Lending?
7.1 Model specification
Our second analysis seeks to identify whether the TFF encouraged business lending by financial institutions that were eligible to access the facility. We compare business lending by banks, which were eligible to access the TFF, relative to non-banks, which were not eligible. This analysis captures the broader range of channels through which the TFF can affect business lending, including the additional allowance incentive captured by the first model, as well as by increasing banks' funding certainty and reducing funding costs. The specification is similar to the first model:
where institution_typeh is a dummy variable equal to 1 if the institution is a bank and 0 if the institution is a non-bank. We also run a version of this regression using fixed-term lending as the dependent variable, although this uses a smaller sample of institutions due to the smaller number of institutions that report outstanding credit by loan type.
Similar to our first set of analysis, we weight the regressions for the baseline and fixed-term model by each institution's level of business credit in the base period.
7.2 Results
For our second question (Equation (6)), we find statistically significant, positive effects on aggregate bank business lending (relative to non-bank lending) for a small number of the 20 post-TFF time periods in our sample (Figure 12; Table C13). These coefficients are generally largest in the early months of the pandemic. In addition, we run regressions for SME and large business credit separately (using data that has the breakdown of business credit by size but a smaller sample of institutions). These models show positive and statistically significant effects on large business credit growth for banks (for most time periods) but insignificant results for SME credit (Figures B9 and B10; Tables C14 and C15).
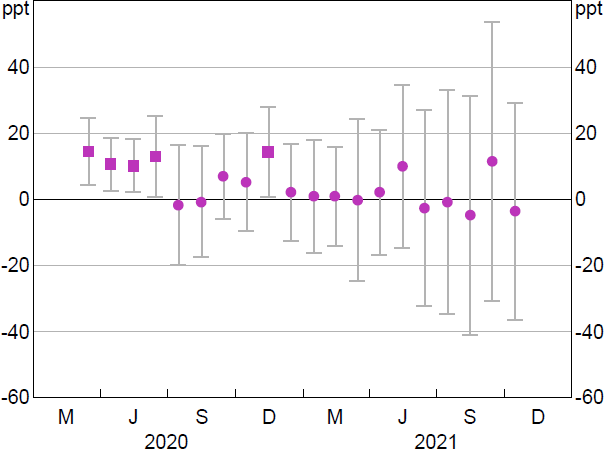
Notes: Whiskers show 95 per cent confidence intervals. The filled square marker indicates a significant result.
Sources: APRA; Authors' calculations; RBA
We also run analysis to compare fixed-term business lending for banks and non-banks. We find no statistically significant effects of the TFF on fixed-term credit growth by banks relative to non-banks for all time periods following the introduction of the TFF (Figure B11; Table C16). Taken together, these results suggest that the significant positive effects on aggregate bank business lending in the early months of the pandemic are driven by larger businesses' precautionary drawdowns on credit lines.
In the regressions that are weighted by each institution's level of business credit in the base period, we find positive and statistically significant coefficients on the bank dummy variable for the months March to July 2020 in the baseline model (Figure B12; Table C17). For the fixed-term lending model, we find no statistically significant results of the TFF on fixed-term credit growth by banks relative to non-banks for most time periods following the introduction of the TFF (Figure B13; Table C18).
8. Did a Lender's Take-up of the TFF Affect Their Business Lending?
8.1 Model specification
Our final set of models investigates whether a lender's growth in business lending was affected by whether or not they accessed funding through the TFF; this is in contrast to the results above, which reflect the availability of the TFF.[16] Similar to the regressions in Section 7, these results capture the full range of channels through which the TFF can affect business lending.
In our first specification, we examine aggregate business credit growth for eligible banks that accessed the TFF compared to banks that did not. These units of analysis are more consistent with international studies, which commonly evaluate outcomes in participating versus non-participating institutions. We begin with a simple OLS specification that is similar to the previous two models:
where accessedTFFi is a binary variable for whether or not a bank accessed the TFF.[17]
However, this gives rise to an endogeneity issue because participation in the TFF was voluntary. As discussed above, we address this through instrumental variables. Firstly, we instrument participation in the TFF with the availability (or adequacy) of self-securitised assets at the beginning of the TFF, using a sample of just banks.[18] The first-stage and instrumental variable specifications are as follows:
where self_secs is either: a binary variable equal to 1 for when a bank has self-securitised assets; or a measure of the adequacy of a bank's self-securitisations (the value of self-securitised assets as a share of the bank's total cash limit).
We also address the endogeneity issue by instrumenting participation in the TFF with a lender's eligibility to access the TFF, using our full sample of bank and non-bank lenders. The first-stage and second-stage specifications are as follows:
where institution_typeh is a binary variable equal to 1 if the institution is a bank and 0 if the institution is a non-bank. This is similar to the instrumental variable regression above using self-securitisations, albeit with a different sample and different set of institutions captured by the estimated treatment effect.
8.2 Results
We start our third analysis by running the basic OLS regression, which compares business credit growth between banks that accessed the TFF and those that did not (Equation (7)). We find no statistically significant effects of accessing the TFF on credit growth (Table C19). The coefficients in this model are volatile around zero, with wide confidence intervals (Figure B14).
However, as discussed above, voluntary participation in the TFF could give rise to an endogeneity issue in this analysis, as it is possible that banks who accessed TFF funding may have increased lending anyway. As such, we address this through instrumental variables. Firstly, we extend the basic OLS regression above by instrumenting participation in the TFF with the availability (or adequacy) of self-securitised assets at the beginning of the TFF. In this model, the first-stage regressions suggest that the availability and/or adequacy of self-securitised assets had a positive and statistically significant effect on whether a bank accessed the TFF, consistent with our expectations (Equation (8)). The t-statistics on the instrument are 5.9 in the model with the binary instrument and 4.3 in the model using self-securitisation adequacy, suggesting the weak instrument test is passed based on the rough rule of thumb of t > 3.16 (Tables C20 and C22).[19] Our second-stage estimates of the effect of the bank accessing the TFF on business lending are generally negative but not statistically significant (Equation (9); Figures 13 and B15; Tables C21 and C23). Our second-stage coefficients also have wide Anderson–Rubin confidence intervals.[20]
As expected, the coefficients in our second-stage regression are generally lower when compared with the coefficients found in our basic OLS version. This is consistent with banks accessing the TFF because they expected to increase lending.
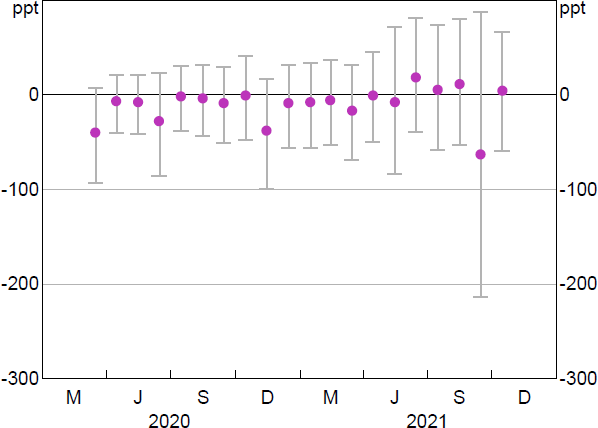
Note: Whiskers show Anderson–Rubin 95 per cent confidence intervals.
Sources: APRA; Authors' calculations; RBA
We repeat the above regressions comparing lending between banks that accessed the TFF and those that did not, but this time using only fixed-term lending (i.e. excluding revolving credit lines). This uses data from the ARF 742_0 A/B form, which has the breakdown of business credit by finance type but a smaller sample of institutions. The first-stage regressions suggest that the availability and/or adequacy of self-securitised assets had a positive and statistically significant effect on whether or not a bank accessed the TFF, consistent with our expectations. The t-statistics on the instrument are 3.1 in the model with the binary instrument and 2.9 in the model using the self-securitisation adequacy, suggesting the instruments may be weak (Tables C24 and C26). Again, we find no statistically significant effect of accessing the TFF on lending for all time periods following the introduction of the TFF (Figures B16 and B17; Tables C25 and C27).
We also instrument participation in the TFF with a lender's eligibility to access the TFF, using our full sample of bank and non-bank lenders. In this model, the first-stage regression (Equation (10)) suggests that, unsurprisingly, eligibility to access the TFF had a positive and statistically significant effect on whether a bank accessed the TFF (Table C28). The t-statistic on the instrument is 17.36, suggesting the weak instrument test is comfortably passed. As expected, our second-stage regression (Equation (11)) finds results similar to the effects of the availability of the scheme but scaled upwards (Figure 14; Table C29). These coefficients are generally positive but are, in most cases, statistically insignificant.
In principle, any difference between these results and those using self-securitisations could reflect differences in samples and differences in the institutions captured by the estimated treatment effect.[21] In any case, the coefficients are statistically insignificant across both results.
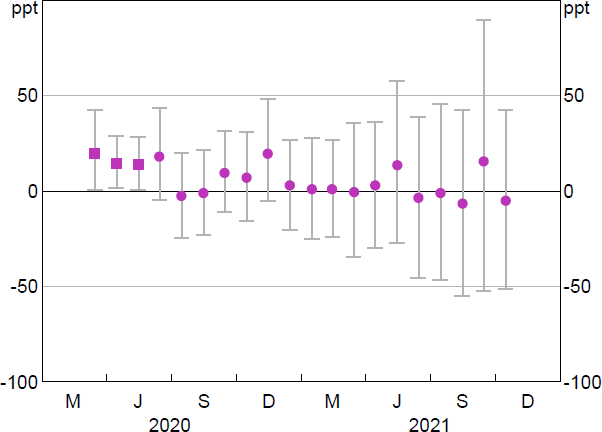
Notes: Whiskers show Anderson–Rubin 95 per cent confidence intervals. The filled square marker indicates a significant result.
Sources: APRA; Authors' calculations; RBA
9. Conclusion
The TFF was introduced in March 2020 as part of a policy package in response to the onset of the COVID-19 pandemic. The facility reduced banks' funding costs and, along with other policies, contributed to lower borrowing rates for households and businesses; two key objectives of the TFF. This paper is focused only on the third objective of the TFF, which was to encourage banks to lend to businesses. Overall, we find no statistically significant effects of the TFF on bank credit growth for SMEs compared to large businesses, and mixed results when comparing aggregate business credit growth for TFF-eligible banks against ineligible non-banks. We also find no statistically significant effects on aggregate business credit growth for eligible banks that accessed the TFF compared to banks that did not draw down. We use a variety of strategies to estimate the effect of the TFF on business lending. Nonetheless, identification challenges remain, reflecting the fact that the TFF was introduced at the same time as a number of other policies and in response to the adverse effect the pandemic would have on the economy, potentially affecting the various lenders and borrowers in different ways.
Our first set of results rely on an assumption that large business credit growth would have been an appropriate counterfactual for SME credit growth in the absence of the TFF, but there are reasons why this assumption may not hold. For example, many SMEs operate in sectors that were more affected by COVID-19 restrictions and may have found it more difficult to access finance after the pandemic. Furthermore, survey data and liaison with businesses suggest that many businesses, particularly SMEs, had little appetite for taking out new loans throughout the pandemic, reflecting ongoing uncertainty about the economic outlook as well as a reduced need for finance due to government support measures and increased cash buffers (Bank and Lewis 2021).[22] These factors may have biased our estimates downwards. While we attempt to address identification issues by using data at the bank and industry level, and by using a triple-difference framework, these strategies may not be sufficient to overcome the identification problems.
In addition, non-banks are not an ideal control in this period. While banks benefited from funding certainty provided by the TFF, non-banks were also being supported by the Structured Finance Support Fund. In addition, non-banks indirectly benefited from the take-up of the TFF, with the decline in bank bond issuance (largely owing to the TFF) contributing to a noticeable decline in spreads on ABS (Kent 2021b). Similarly, the decline in issuance also contributed to a decline in spreads on bank bonds. This benefited banks that did not access the TFF, complicating the comparison of their business lending with those that did draw on the facility.
This paper contributes to the growing literature studying the effect of term funding schemes implemented around the world. It is also, to the best of our knowledge, the first empirical study of the effects of the RBA's TFF on bank credit supply in Australia. Similar to our results, global evidence to date is mixed on the effectiveness of these features to promote lending to particular parts of the economy.
Appendix A: Data Description
Our analysis primarily draws on confidential data reported to APRA by banks and non-banks. We draw mostly on data collected under the EFS collection, which have been available since mid-2019.
For our analysis examining whether the incentive in the TFF's additional allowance was effective, we largely draw on data from EFS form ARF 742_0 A/B, which contains data on business credit stocks, flows and interest rates. This form also contains breakdowns of business credit by firm size and industry (e.g. manufacturing). Banks and non-banks that have $2 billion of business credit or more are required to report under this form; this threshold is estimated to capture over 95 per cent of total business credit.
For our first analysis comparing SME and large business credit growth for banks, we draw on data on outstanding business credit by size from form ARF 742_0 A/B.[23] We supplement this with data reported directly to the RBA for the purposes of calculating the TFF additional allowance by banks who did not report on form ARF 742_0 A/B; 15 banks chose to report SME and large business credit outstanding directly to the RBA from November 2019 until April 2021.[24],[25] These data are adjusted to account for breaks in the series, mostly to account for reclassifications between business sizes. In total, 50 banks reported business lending data by size throughout the drawdown period, although our baseline model in the first analysis excludes 2 banks (outliers) that reported extremely large growth rates for some periods.[26]
Form ARF 742_0 A/B also contains a number of subcomponent breakdowns for business credit outstanding, including lending to businesses in each of 25 different industries. We group these industries into four broad groups (household services, business services, goods production and goods distribution) due to data volatility at the more granular industry level.[27],[28] The industry dataset allows us to run a robustness check on our baseline estimates using data at the bank–industry level. The key rationale for running models at the bank–industry level is to control for uneven effects of the pandemic across industries, based on the notion that businesses within these broad industry groups were similarly affected by COVID-19 restrictions. Unlike our first analysis, the industry-level data are not break adjusted to account for reclassifications between business sizes, owing to a lack of readily available data.
The ARF 742_0 A/B form also includes subcomponent breakdowns for business credit outstanding by loan type. From this, we construct a dataset that is limited to fixed-term loans (defined as loans extended for a fixed period and with a maturity date by which the loan must be repaid). Our rationale for running models with just fixed-term lending is to look through larger businesses drawing down on lines of credit for precautionary reasons; banks would have likely expected these loans to be short term and to not influence the final additional allowance. Indeed, these firms generally repaid these funds within a couple of months.
Data on bank characteristics are sourced from various APRA forms to examine whether the effect of the TFF on business credit growth differed by bank characteristics such as size, liquidity and capital ratios.[29] We use data as at December 2019 (prior to the TFF) to avoid endogeneity issues. Descriptive statistics for a subset of banks are in Table A1; these statistics cover the 50 banks that report the breakdowns of business credit by firm size, although some of these statistics cover a smaller sample of banks due to a different set of reporting thresholds than EFS forms ARF 720_1 and ARF 742_0.
Statistic | Description | No of obs | Mean | Std dev | Min | Percentile | Max | |
---|---|---|---|---|---|---|---|---|
(25) | (75) | |||||||
Total assets ($b) | Total assets(a) | 50 | 73.6 | 194.1 | 0.4 | 2.9 | 25.2 | 800.9 |
Liquidity ratio (%) | Liquid assets/total assets | 50 | 16.1 | 8.6 | 5.2 | 10.2 | 21.9 | 39.8 |
Net interest income ratio (%) | Net interest income/total operating income | 50 | 65.1 | 26.2 | −2.2 | 53.1 | 84.9 | 102.7 |
Funding costs ratio (%) | Interest expenses/interest bearing liabilities | 50 | 0.5 | 0.3 | 0.0 | 0.3 | 0.5 | 2.0 |
Provisions ratio (%) | Collective provisions/total loans and finance leases | 50 | 0.2 | 0.2 | 0.0 | 0.0 | 0.4 | 1.0 |
Common equity tier 1 capital ratio (%) | Common equity tier 1 capital/risk-weighted assets | 14 | 14.5 | 3.0 | 10.7 | 12.4 | 15.7 | 20.7 |
Total tier 1 capital ratio (%) | Tier 1 capital/risk-weighted assets | 14 | 15.1 | 2.4 | 12.9 | 13.3 | 15.7 | 20.7 |
Tier 1 leverage ratio (%) | Tier 1 capital/total assets | 14 | 8.2 | 2.0 | 5.9 | 7.1 | 8.8 | 13.7 |
Note: (a) Excludes intragroup assets for banks that report on ARF 720_0_A/B. Sources: APRA; Authors' calculations; RBA |
For our analysis examining whether the availability or take-up of the TFF affected lending, we use data from EFS form ARF 720_1 A/B, which contains data on loans and leases for a larger set of banks than ARF 742_0 A/B. Form ARF 720_1 A/B includes data on total business credit (outstanding loans and finance leases) to community service organisations, non-financial businesses and financial institutions. This form captures monthly data from most banks, as well as non-banks with more than $400 million in total assets. While this form does not include a breakdown of business credit by firm size, its lower thresholds relative to ARF 742_0 A/B means it has the advantage of a larger number of reporting institutions. We use a balanced panel of 114 banks and 48 non-banks in our analysis.
Form | No of institutions | Description of data available | Sample period |
---|---|---|---|
APRA EFS form ARF 742_0 A/B | 35 banks | Small, medium and large business credit outstanding Industry-level breakdown of business credit by size Finance-type breakdown of business credit by size | October 2019 to October 2021 used in models |
Direct report to RBA | 15 banks | SME and large business credit outstanding | November 2019 and April 2021 |
APRA EFS form ARF 720_1 A/B | 114 banks 48 non-banks | Outstanding loans and finance leases to community service organisations, non-financial businesses and financial institutions | October 2019 to October 2021 used in models |
Sources: APRA; RBA |
Aside from data reported to APRA through the EFS forms, we use RBA data on the availability (or adequacy) of banks' self-securitised assets as the instrumental variable in our analysis of how banks' participation in the TFF affected lending. To avoid endogeneity issues we use data as at May 2020, prior to some banks establishing self-securitisations to facilitate accessing the TFF. Of the 114 banks in our sample, 46 had self-securitised assets established prior to the TFF.
Appendix B: Coefficient Figures
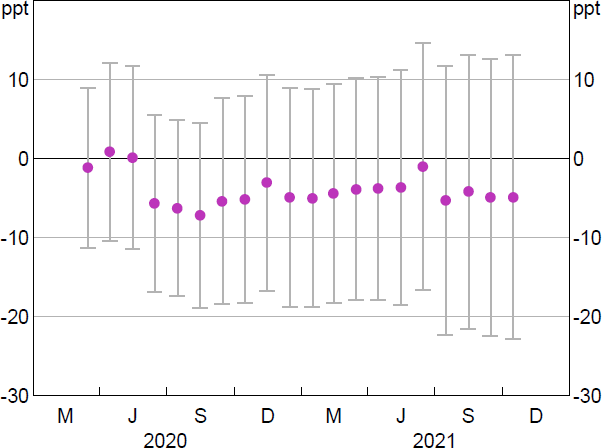
Note: Whiskers show 95 per cent confidence intervals.
Sources: APRA; Authors' calculations; RBA
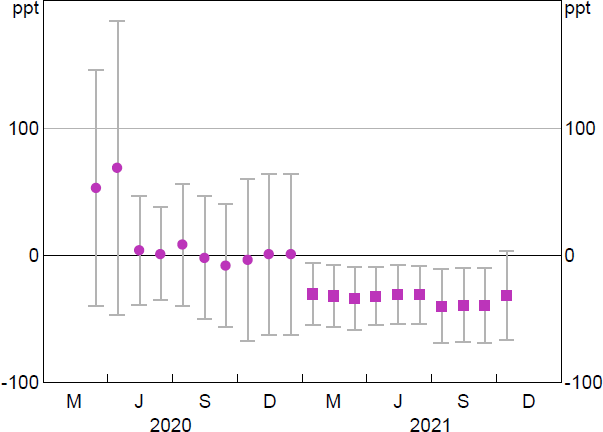
Notes: Whiskers show 95 per cent confidence intervals. The filled square marker indicates a significant result.
Sources: APRA; Authors' calculations; RBA
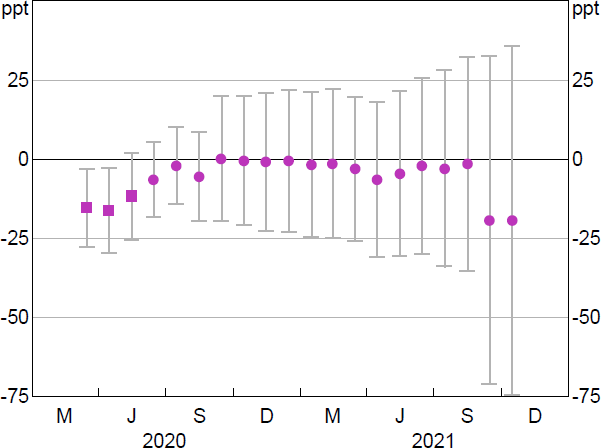
Notes: Whiskers show 95 per cent confidence intervals. The filled square marker indicates a significant result.
Sources: APRA; Authors' calculations; RBA
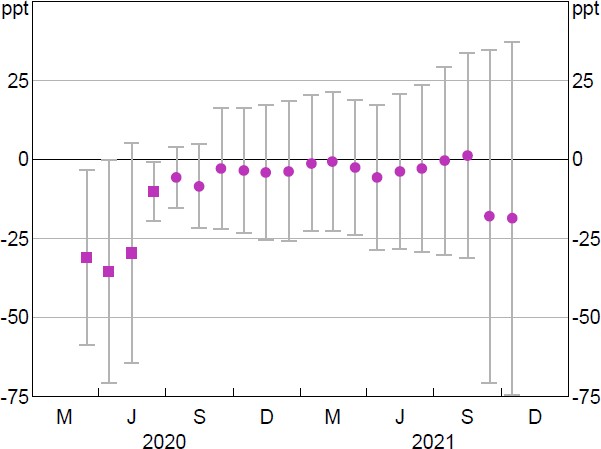
Notes: Whiskers show 95 per cent confidence intervals. The filled square marker indicates a significant result.
Sources: APRA; Authors' calculations; RBA
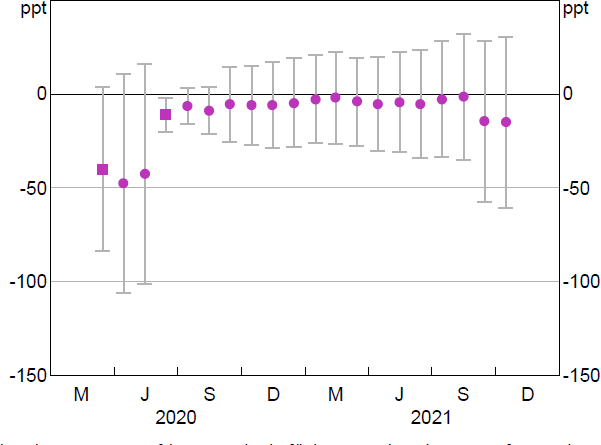
Notes: Whiskers show 95 per cent confidence intervals. The filled square marker indicates a significant result.
Sources: APRA; Authors' calculations; RBA
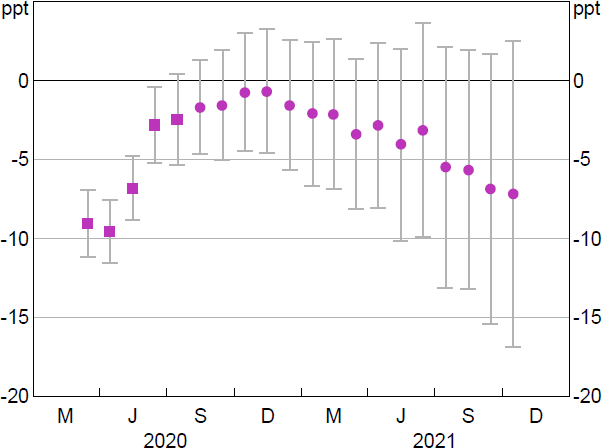
Notes: Weighted by each bank's level of business credit in the base period. Whiskers show 95 per cent confidence intervals. The filled square marker indicates a significant result.
Sources: APRA; Authors' calculations; RBA
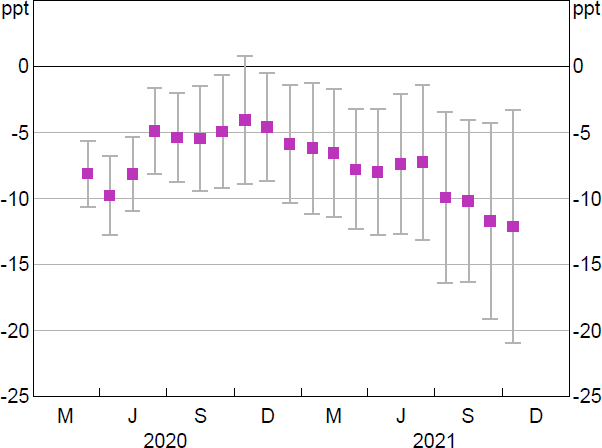
Notes: Weighted by each institution's level of business credit in the base period. Whiskers show 95 per cent confidence intervals. The filled square marker indicates a significant result.
Sources: APRA; Authors' calculations; RBA
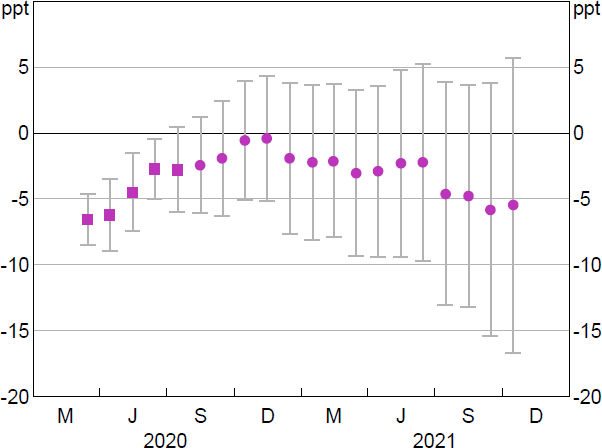
Notes: Weighted by each bank's level of business credit in the base period. Whiskers show 95 per cent confidence intervals. The filled square marker indicates a significant result.
Sources: APRA; Authors' calculations; RBA
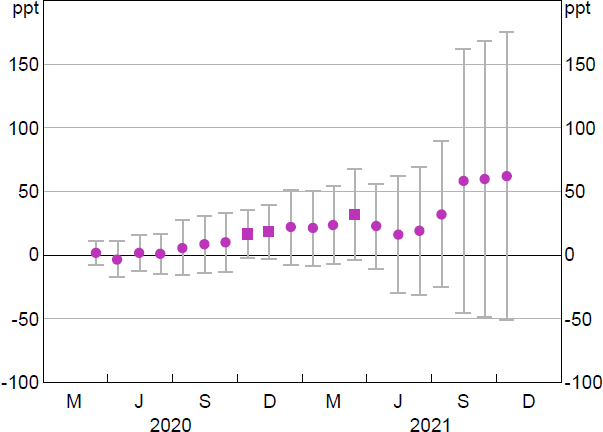
Notes: Whiskers show 95 per cent confidence intervals. The filled square marker indicates a significant result.
Sources: APRA; Authors' calculations; RBA
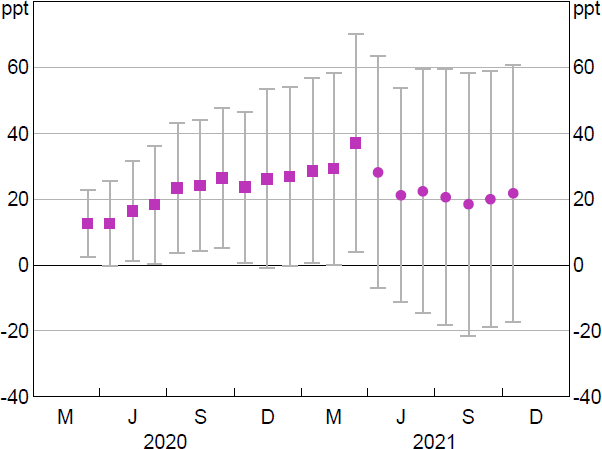
Notes: Whiskers show 95 per cent confidence intervals. The filled square marker indicates a significant result.
Sources: APRA; Authors' calculations; RBA
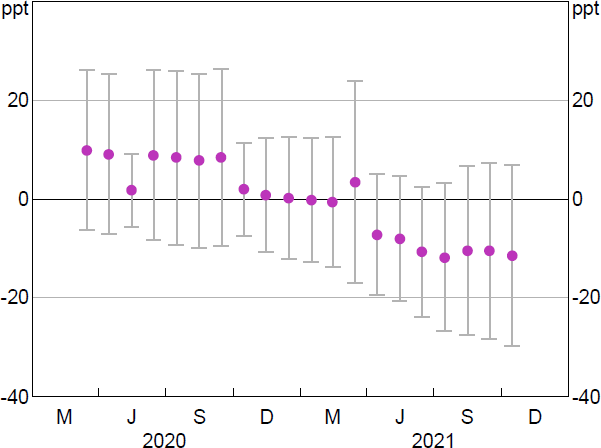
Note: Whiskers show 95 per cent confidence intervals.
Sources: APRA; Authors' calculations; RBA
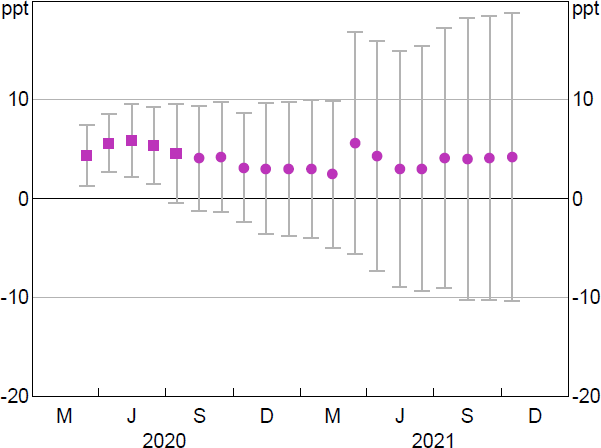
Notes:
Weighted by each institution's level of business credit in the base period. Whiskers show 95 per cent confidence intervals.
The filled square marker indicates a significant result.
Sources: APRA; Authors' calculations; RBA
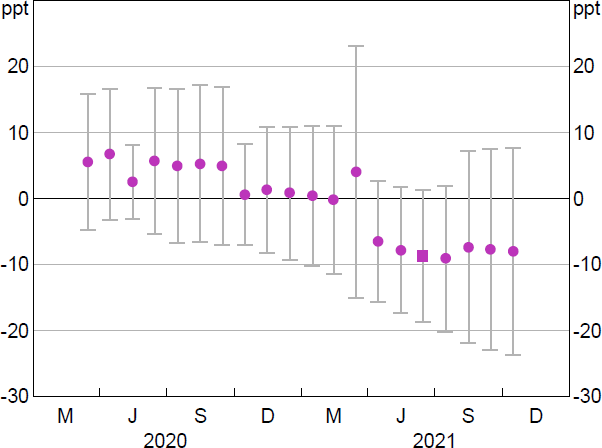
Notes:
Weighted by each institution's level of business credit in the base period. Whiskers show 95 per cent confidence intervals.
The filled square marker indicates a significant result.
Sources: APRA; Authors' calculations; RBA
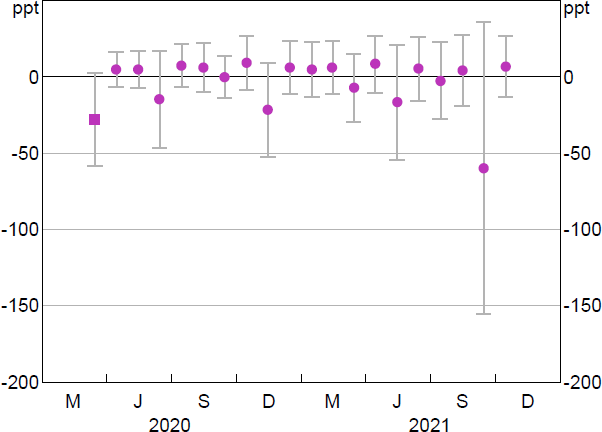
Notes: Whiskers show 95 per cent confidence intervals. The filled square marker indicates a significant result.
Sources: APRA; Authors' calculations; RBA
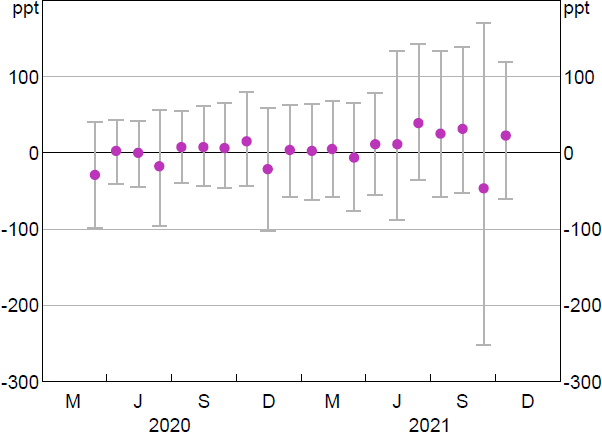
Note: Whiskers show Anderson–Rubin 95 per cent confidence intervals.
Sources: APRA; Authors' calculations; RBA
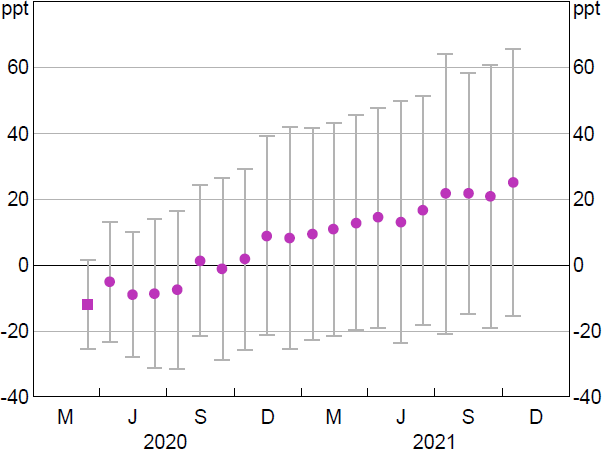
Notes: Whiskers show 95 per cent confidence intervals. The filled square marker indicates a significant result.
Sources: APRA; Authors' calculations; RBA
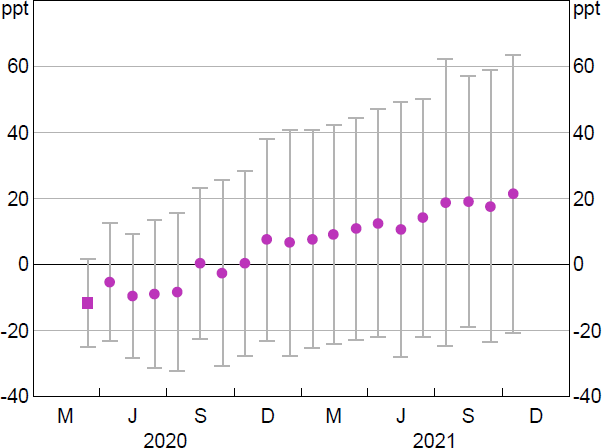
Notes: Whiskers show 95 per cent confidence intervals. The filled square marker indicates a significant result.
Sources: APRA; Authors' calculations; RBA
Appendix C: Full Results
2020 | ||||||||||
---|---|---|---|---|---|---|---|---|---|---|
Mar | Apr | May | Jun | Jul | Aug | Sep | Oct | Nov | Dec | |
SME borrower | −5.170 (4.025) |
−4.057 (3.945) |
−2.575 (4.128) |
−0.425 (4.721) |
0.444 (4.736) |
2.061 (4.961) |
2.063 (5.696) |
2.462 (5.580) |
2.547 (5.729) |
3.620 (5.798) |
Constant | 8.340** (3.152) |
8.095** (3.381) |
7.922** (3.855) |
5.239 (4.080) |
4.847 (4.147) |
4.750 (4.478) |
5.665 (4.709) |
5.486 (5.023) |
4.595 (4.935) |
4.282 (4.926) |
No of obs | 80 | 80 | 80 | 80 | 80 | 80 | 80 | 80 | 80 | 80 |
R2 | 0.013 | 0.008 | 0.003 | 0.000 | 0.000 | 0.001 | 0.001 | 0.001 | 0.001 | 0.003 |
R2 adj | 0.000 | −0.005 | −0.010 | −0.013 | −0.013 | −0.012 | −0.012 | −0.011 | −0.011 | −0.010 |
2021 | ||||||||||
Jan | Feb | Mar | Apr | May(a) | Jun | Jul | Aug | Sep | Oct | |
SME borrower | 2.691 (6.060) |
4.154 (6.207) |
5.371 (6.884) | 3.743 (6.942) |
−6.468 (5.889) |
−3.136 (7.039) |
5.515 (12.028) |
6.470 (12.019) |
4.991 (11.682) |
4.930 (11.741) |
Constant | 4.560 (5.150) |
5.072 (5.256) |
7.508 (5.653) |
10.305 (8.364) |
5.087 (3.996) |
3.219 (4.087) |
4.565 (4.293) |
3.992 (4.101) |
4.442 (4.133) |
4.949 (4.322) |
No of obs | 80 | 79 | 79 | 79 | 57 | 58 | 57 | 58 | 58 | 58 |
R2 | 0.001 | 0.003 | 0.005 | 0.001 | 0.023 | 0.004 | 0.005 | 0.007 | 0.004 | 0.004 |
R2 adj | −0.011 | −0.010 | −0.008 | −0.012 | 0.005 | −0.013 | −0.013 | −0.011 | −0.014 | −0.014 |
Notes: ***, ** and * denote statistical significance at the 1, 5 and 10 per cent levels, respectively. Standard errors are in parentheses. Sources: APRA; Authors' calculations; RBA |
2020 | ||||||||||
---|---|---|---|---|---|---|---|---|---|---|
Mar | Apr | May | Jun | Jul | Aug | Sep | Oct | Nov | Dec | |
SME borrower | −1.231 (4.958) |
0.822 (5.526) |
0.051 (5.675) |
−5.707 (5.513) |
−6.269 (5.451) |
−7.178 (5.737) |
−5.419 (6.372) |
−5.195 (6.428) |
−3.083 (6.701) |
−4.981 (6.809) |
Constant | 4.189** (1.597) |
2.521 (1.707) |
4.023** (1.970) |
5.039* (2.679) |
5.414* (2.727) |
4.750 (2.820) |
4.601 (3.188) |
4.107 (3.144) |
2.408 (3.651) |
3.601 (3.990) |
No of obs | 55 | 55 | 55 | 55 | 55 | 55 | 55 | 55 | 55 | 55 |
R2 | 0.001 | 0.000 | 0.000 | 0.020 | 0.024 | 0.030 | 0.015 | 0.013 | 0.004 | 0.010 |
R2 adj | −0.018 | −0.018 | −0.019 | 0.002 | 0.005 | 0.012 | −0.004 | −0.005 | −0.015 | −0.009 |
2021 | ||||||||||
Jan | Feb | Mar | Apr | May | Jun | Jul | Aug | Sep | Oct | |
SME borrower | −5.059 (6.771) |
−4.439 (6.772) |
−3.880 (6.859) |
−3.785 (6.930) |
−3.744 (7.282) |
−1.015 (7.677) |
−5.340 (8.330) |
−4.250 (8.494) |
−4.978 (8.611) |
−4.946 (8.810) |
Constant | 2.851 (3.802) |
3.135 (3.867) |
3.242 (3.864) |
2.551 (3.929) |
2.854 (3.935) |
1.000 (4.141) |
2.109 (4.588) |
1.583 (4.416) |
2.263 (4.726) |
2.168 (4.875) |
No of obs | 55 | 55 | 55 | 55 | 54 | 55 | 54 | 55 | 55 | 55 |
R2 | 0.011 | 0.009 | 0.007 | 0.006 | 0.006 | 0.000 | 0.009 | 0.006 | 0.007 | 0.007 |
R2 adj | −0.008 | −0.010 | −0.012 | −0.013 | −0.013 | −0.018 | −0.010 | −0.013 | −0.011 | −0.012 |
Notes: ***, ** and * denote statistical significance at the 1, 5 and 10 per cent levels, respectively. Standard errors are in parentheses. Sources: APRA; Authors' calculations; RBA |
2020 | ||||||||||
---|---|---|---|---|---|---|---|---|---|---|
Mar | Apr | May | Jun | Jul | Aug | Sep | Oct | Nov | Dec | |
Small business borrower | 52.948 (45.128) |
68.169 (56.327) |
3.535 (20.852) |
0.768 (17.719) |
7.872 (23.371) |
−2.182 (23.386) |
−8.151 (23.576) |
−4.124 (31.003) |
0.222 (30.739) |
0.476 (30.763) |
Constant | −2.537 (3.887) |
−1.522 (3.417) |
0.705 (3.254) |
−1.128 (3.544) |
−1.733 (3.545) |
−0.948 (4.130) |
0.326 (4.558) |
0.600 (4.551) |
−1.192 (4.572) |
−1.278 (4.610) |
No of obs | 49 | 49 | 49 | 49 | 49 | 49 | 49 | 49 | 49 | 49 |
R2 | 0.034 | 0.037 | 0.001 | 0.000 | 0.003 | 0.000 | 0.003 | 0.000 | 0.000 | 0.000 |
R2 adj | 0.013 | 0.017 | −0.021 | −0.021 | −0.018 | −0.021 | −0.018 | −0.021 | −0.021 | −0.021 |
2021 | ||||||||||
Jan | Feb | Mar | Apr | May | Jun | Jul | Aug | Sep | Oct | |
Small business borrower | −30.814** (12.049) |
−32.353** (11.955) |
−34.220*** (11.958) |
−32.607*** (11.109) |
−31.329*** (11.184) |
−31.273*** (11.058) |
−40.440*** (14.116) |
−39.569** (14.245) |
−39.691*** (14.p234) |
−32.238* (16.977) |
Constant | −2.137 (4.578) |
−0.769 (4.373) |
0.860 (4.600) |
−0.358 (3.986) |
0.940 (4.101) |
2.592 (4.852) |
12.095 (9.885) |
12.612 (10.021) |
11.624 (9.878) |
12.031 (9.923) |
No of obs | 49 | 49 | 49 | 49 | 49 | 49 | 49 | 49 | 49 | 49 |
R2 | 0.145 | 0.161 | 0.176 | 0.169 | 0.156 | 0.145 | 0.140 | 0.129 | 0.133 | 0.072 |
R2 adj | 0.127 | 0.143 | 0.158 | 0.151 | 0.138 | 0.126 | 0.122 | 0.111 | 0.114 | 0.052 |
Notes: ***, ** and * denote statistical significance at the 1, 5 and 10 per cent levels, respectively. Standard errors are in parentheses. Sources: APRA; Authors' calculations; RBA |
2020 | |||||||||||
---|---|---|---|---|---|---|---|---|---|---|---|
Mar | Apr | May | Jun | Jul | Aug | Sep | Oct | Nov | Dec | ||
SME borrower | −15.483** (6.058) |
−16.486** (6.578) |
−11.858* (6.748) |
−6.569 (5.854) |
−2.283 (5.971) |
−5.608 (6.957) |
−0.101 (9.702) |
−0.724 (10.018) |
−1.128 (10.706) |
−0.760 (11.081) |
|
No of obs | 218 | 218 | 218 | 218 | 218 | 218 | 217 | 217 | 217 | 217 | |
R2 | 0.988 | 0.993 | 0.993 | 0.764 | 0.710 | 0.671 | 0.692 | 0.690 | 0.697 | 0.704 | |
R2 adj | 0.969 | 0.983 | 0.982 | 0.406 | 0.269 | 0.171 | 0.227 | 0.222 | 0.238 | 0.258 | |
2021 | |||||||||||
Jan | Feb | Mar | Apr | May | Jun | Jul | Aug | Sep | Oct | ||
SME borrower | −1.839 (11.276) |
−1.552 (11.585) |
−3.349 (11.206) |
−6.608 (12.048) |
−4.705 (12.850) |
−2.204 (13.677) |
−3.105 (15.213) |
−1.793 (16.595) |
−19.452 (25.510) |
−19.582 (27.156) |
|
No of obs | 217 | 217 | 217 | 217 | 213 | 217 | 213 | 217 | 216 | 216 | |
R2 | 0.633 | 0.644 | 0.650 | 0.621 | 0.601 | 0.642 | 0.580 | 0.564 | 0.513 | 0.511 | |
R2 adj | 0.078 | 0.105 | 0.120 | 0.048 | 0.016 | 0.101 | −0.035 | −0.094 | −0.231 | −0.236 | |
Notes: ***, ** and * denote statistical significance at the 1, 5 and 10 per cent levels, respectively. Standard errors are in parentheses. Sources: APRA; Authors' calculations; RBA |
2020 | ||||||||||
---|---|---|---|---|---|---|---|---|---|---|
Mar | Apr | May | Jun | Jul | Aug | Sep | Oct | Nov | Dec | |
SME borrower | −31.037** (13.583) |
−35.546** (17.326) |
−29.595* (17.126) |
−10.181** (4.569) |
−5.800 (4.795) |
−8.596 (6.528) |
−2.778 (9.396) |
−3.518 (9.708) |
−4.163 (10.466) |
−3.766 (10.825) |
No of obs | 218 | 218 | 218 | 218 | 218 | 218 | 217 | 217 | 217 | 217 |
R2 | 0.235 | 0.202 | 0.205 | 0.217 | 0.198 | 0.150 | 0.174 | 0.185 | 0.205 | 0.212 |
R2 adj | 0.098 | 0.059 | 0.063 | 0.077 | 0.054 | −0.002 | 0.026 | 0.038 | 0.062 | 0.070 |
2021 | ||||||||||
Jan | Feb | Mar | Apr | May | Jun | Jul | Aug | Sep | Oct | |
SME borrower | −1.300 (10.574) |
−0.743 (10.848) |
−2.601 (10.495) |
−5.765 (11.278) |
−3.850 (12.073) |
−2.827 (12.979) |
−0.571 (14.604) |
1.070 (15.935) |
−18.126 (25.791) |
−18.699 (27.391) |
No of obs | 217 | 217 | 217 | 217 | 213 | 217 | 213 | 217 | 216 | 216 |
R2 | 0.243 | 0.258 | 0.264 | 0.259 | 0.246 | 0.234 | 0.251 | 0.249 | 0.173 | 0.168 |
R2 adj | 0.107 | 0.124 | 0.131 | 0.125 | 0.112 | 0.096 | 0.118 | 0.113 | 0.023 | 0.017 |
Notes: ***, ** and * denote statistical significance at the 1, 5 and 10 per cent levels, respectively. Standard errors are in parentheses. Sources: APRA; Authors' calculations; RBA |
2020 | |||||||||||
---|---|---|---|---|---|---|---|---|---|---|---|
Mar | Apr | May | Jun | Jul | Aug | Sep | Oct | Nov | Dec | ||
SME borrower | −40.257* (21.489) |
−47.930 (28.749) |
−42.872 (28.738) |
−11.217** (4.389) |
−6.681 (4.738) |
−8.829 (6.158) |
−5.770 (9.902) |
−6.170 (10.278) |
−6.066 (11.215) |
−4.899 (11.654) |
|
No of obs | 218 | 218 | 218 | 218 | 218 | 218 | 217 | 217 | 217 | 217 | |
R2 | 0.025 | 0.021 | 0.019 | 0.041 | 0.041 | 0.050 | 0.047 | 0.045 | 0.035 | 0.032 | |
R2 adj | 0.007 | 0.003 | 0.001 | 0.023 | 0.023 | 0.032 | 0.029 | 0.027 | 0.017 | 0.014 | |
2021 | |||||||||||
Jan | Feb | Mar | Apr | May | Jun | Jul | Aug | Sep | Oct | ||
SME borrower | −2.806 (11.611) |
−2.230 (12.005) |
−4.270 (11.567) |
−5.460 (12.257) |
−4.321 (13.062) |
−5.350 (14.147) |
−3.002 (15.160) |
−1.681 (16.447) |
−14.712 (21.172) |
−15.256 (22.392) |
|
No of obs | 217 | 217 | 217 | 217 | 213 | 217 | 213 | 217 | 216 | 216 | |
R2 | 0.034 | 0.035 | 0.035 | 0.021 | 0.017 | 0.021 | 0.028 | 0.023 | 0.020 | 0.021 | |
R2 adj | 0.016 | 0.017 | 0.017 | 0.002 | −0.002 | 0.003 | 0.009 | 0.004 | 0.001 | 0.002 | |
Notes: ***, ** and * denote statistical significance at the 1, 5 and 10 per cent levels, respectively. Standard errors are in parentheses. Sources: APRA; Authors' calculations; RBA |
2020 | ||||||||||
---|---|---|---|---|---|---|---|---|---|---|
Mar | Apr | May | Jun | Jul | Aug | Sep | Oct | Nov | Dec | |
SME borrower | −9.106*** (1.051) |
−9.599*** (0.976) |
−6.871*** (1.006) |
−2.861** (1.197) |
−2.503* (1.438) |
−1.724 (1.480) |
−1.611 (1.723) |
−0.785 (1.861) |
−0.734 (1.955) |
−1.604 (2.051) |
Constant | 8.882*** (1.204) |
9.189*** (1.111) |
6.832*** (1.154) |
3.929*** (1.021) |
2.400* (1.304) |
1.466 (1.324) |
1.120 (1.424) |
0.268 (1.460) |
−0.281 (1.546) |
0.142 (1.613) |
No of obs | 84 | 84 | 84 | 84 | 84 | 84 | 84 | 84 | 84 | 84 |
R2 | 0.328 | 0.362 | 0.196 | 0.017 | 0.012 | 0.005 | 0.004 | 0.001 | 0.001 | 0.003 |
R2 adj | 0.320 | 0.354 | 0.186 | 0.005 | 0.000 | −0.007 | −0.008 | −0.011 | −0.011 | −0.009 |
2021 | ||||||||||
Jan | Feb | Mar | Apr | May | Jun | Jul | Aug | Sep | Oct | |
SME borrower | −2.154 (2.259) |
−2.176 (2.363) |
−3.449 (2.356) |
−2.864 (2.594) |
−4.108 (2.986) |
−3.197 (3.330) |
−5.541 (3.752) |
−5.687 (3.723) |
−6.896 (4.206) |
−7.232 (4.757) |
Constant | 0.348 (1.800) |
0.607 (1.974) |
2.389 (2.035) |
1.936 (2.268) |
1.822 (2.485) |
2.849 (2.637) |
4.677 (2.864) |
5.053 (3.013) |
6.354** (3.118) |
6.957** (3.129) |
No of obs | 84 | 83 | 83 | 83 | 61 | 62 | 61 | 62 | 62 | 62 |
R2 | 0.005 | 0.005 | 0.008 | 0.007 | 0.018 | 0.009 | 0.027 | 0.026 | 0.037 | 0.031 |
R2 adj | −0.007 | −0.007 | −0.004 | −0.005 | 0.001 | −0.008 | 0.010 | 0.009 | 0.021 | 0.015 |
Notes: Weighted by each bank's level of business credit in the base period. ***, ** and * denote statistical significance at the 1, 5 and 10 per cent levels, respectively. Standard errors are in parentheses. Sources: APRA; Authors' calculations; RBA |
2020 | ||||||||||
---|---|---|---|---|---|---|---|---|---|---|
Mar | Apr | May | Jun | Jul | Aug | Sep | Oct | Nov | Dec | |
SME borrower | −8.149*** (1.225) |
−9.801*** (1.490) |
−8.162*** (1.389) |
−4.908*** (1.610) |
−5.383*** (1.660) |
−5.461*** (1.965) |
−4.946** (2.095) |
−4.086* (2.382) |
−4.610** (2.017) |
−5.880** (2.196) |
No of obs | 228 | 228 | 228 | 228 | 228 | 228 | 227 | 227 | 227 | 227 |
R2 | 0.789 | 0.761 | 0.716 | 0.736 | 0.724 | 0.674 | 0.714 | 0.708 | 0.708 | 0.691 |
R2 adj | 0.455 | 0.384 | 0.268 | 0.318 | 0.289 | 0.158 | 0.266 | 0.251 | 0.251 | 0.205 |
2021 | ||||||||||
Jan | Feb | Mar | Apr | May | Jun | Jul | Aug | Sep | Oct | |
SME borrower | −6.215** (2.443) |
−6.563*** (2.381) |
−7.823*** (2.240) |
−8.026*** (2.367) |
−7.401*** (2.614) |
−7.275** (2.890) |
−9.949*** (3.201) |
−10.231*** (3.018) |
−11.743*** (3.656) |
−12.139*** (4.352) |
No of obs | 227 | 226 | 227 | 227 | 223 | 227 | 223 | 227 | 226 | 226 |
R2 | 0.689 | 0.703 | 0.671 | 0.713 | 0.711 | 0.714 | 0.690 | 0.688 | 0.672 | 0.653 |
R2 adj | 0.201 | 0.232 | 0.156 | 0.263 | 0.270 | 0.264 | 0.217 | 0.200 | 0.152 | 0.102 |
Notes: Weighted by each institution's level of business credit in the base period. ***, ** and * denote statistical significance at the 1, 5 and 10 per cent levels, respectively. Standard errors are in parentheses. Sources: APRA; Authors' calculations; RBA |
2020 | |||||||||||
---|---|---|---|---|---|---|---|---|---|---|---|
Mar | Apr | May | Jun | Jul | Aug | Sep | Oct | Nov | Dec | ||
SME borrower | −6.579*** (0.955) |
−6.247*** (1.349) |
−4.531*** (1.456) |
−2.756** (1.123) |
−2.822* (1.579) |
−2.462 (1.777) |
−1.958 (2.143) |
−0.613 (2.213) |
−0.468 (2.332) |
−1.986 (2.802) |
|
Constant | 6.416*** (0.783) |
6.061*** (1.243) |
5.048*** (1.328) |
4.306*** (0.930) |
3.606** (1.490) |
3.058* (1.642) |
2.318 (1.932) |
1.116 (2.023) |
0.765 (2.157) |
2.042 (2.731) |
|
No of obs | 55 | 55 | 55 | 55 | 55 | 55 | 55 | 55 | 55 | 55 | |
R2 | 0.408 | 0.267 | 0.156 | 0.060 | 0.050 | 0.030 | 0.015 | 0.001 | 0.001 | 0.009 | |
R2 adj | 0.397 | 0.253 | 0.140 | 0.042 | 0.032 | 0.012 | −0.004 | −0.017 | −0.018 | −0.009 | |
2021 | |||||||||||
Jan | Feb | Mar | Apr | May | Jun | Jul | Aug | Sep | Oct | ||
SME borrower | −2.286 (2.881) |
−2.143 (2.843) |
−3.089 (3.090) |
−2.944 (3.171) |
−2.332 (3.465) |
−2.246 (3.670) |
−4.628 (4.149) |
−4.790 (4.138) |
−5.838 (4.715) |
−5.512 (5.492) |
|
Constant | 2.020 (2.806) |
1.987 (2.813) |
3.184 (3.067) |
3.094 (3.244) |
2.691 (3.460) |
4.184 (3.570) |
6.176 (3.804) |
6.719 (4.043) |
7.913* (4.431) |
8.013 (4.884) |
|
No of obs | 55 | 55 | 55 | 55 | 54 | 55 | 54 | 55 | 55 | 55 | |
R2 | 0.012 | 0.011 | 0.020 | 0.017 | 0.011 | 0.009 | 0.034 | 0.034 | 0.043 | 0.035 | |
R2 adj | −0.006 | −0.008 | 0.002 | −0.001 | −0.008 | −0.010 | 0.015 | 0.015 | 0.025 | 0.017 | |
Notes: Weighted by each bank's level of business credit in the base period. ***, ** and * denote statistical significance at the 1, 5 and 10 per cent levels, respectively. Standard errors are in parentheses. Sources: APRA; Authors' calculations; RBA |
(1) | (2) | (3) | (4) | (5) | (6) | (7) | (8) | |
---|---|---|---|---|---|---|---|---|
SME borrower | 78.036 (100.926) |
13.723* (8.188) |
12.408 (12.816) |
3.267 (17.589) |
17.363 (12.500) |
−120.931** (47.154) |
−152.743* (73.749) |
20.895 (70.553) |
Log total assets | −5.695 (6.050) |
|||||||
SME borrower x log total assets | −3.191 (4.069) |
|||||||
Provisions ratio | 42.523* (24.838) |
|||||||
SME borrower x provisions ratio | −35.384** (15.411) |
|||||||
Liquidity ratio | 1.242 (1.265) |
|||||||
SME borrower x liquidity ratio | −0.457 (0.752) |
|||||||
Net interest income ratio | 0.192 (0.413) |
|||||||
SME borrower x net interest income ratio | 0.011 (0.265) |
|||||||
Funding costs ratio | 30.394 (19.747) |
|||||||
SME borrower x funding costs ratio | −25.885 (17.952) |
|||||||
Common equity tier 1 capital ratio | −7.720*** (1.978) |
|||||||
SME borrower x common equity tier 1 capital ratio | 10.734** (3.913) |
|||||||
Total tier 1 capital ratio | −9.347*** (2.273) |
|||||||
SME borrower x total tier 1 capital ratio | 12.190** (5.096) |
|||||||
Leverage to total tier 1 capital | −1.423 (5.906) |
|||||||
SME borrower x leverage to total tier 1 capital | 0.485 (6.913) |
|||||||
Constant | 143.225 (149.838) |
−1.529 (6.999) |
−11.015 (15.571) |
−3.279 (21.351) |
−5.479 (11.012) |
89.750*** (21.638) |
122.042*** (35.462) |
−1.814 (59.743) |
No of obs | 78 | 78 | 78 | 78 | 78 | 21 | 21 | 21 |
R2 | 0.080 | 0.029 | 0.040 | 0.012 | 0.019 | 0.319 | 0.289 | 0.124 |
R2 adj | 0.043 | −0.010 | 0.002 | −0.028 | −0.020 | 0.199 | 0.163 | −0.031 |
Notes: ***, ** and * denote statistical significance at the 1, 5 and 10 per cent levels, respectively. Standard errors are in parentheses. Sources: APRA; Authors' calculations; RBA |
(1) | |
---|---|
SME borrower x TFF | −0.118 (0.087) |
No of obs | 1,863 |
R2 | 0.716 |
R2 adj | 0.704 |
Fixed effects: bank | |
Fixed effects: period | |
Note: Standard errors are in parentheses. Sources: APRA; Authors' calculations; RBA |
2020 | |||||||||||
---|---|---|---|---|---|---|---|---|---|---|---|
Mar | Apr | May | Jun | Jul | Aug | Sep | Oct | Nov | Dec | ||
SME borrower | 6.258* (3.476) |
12.971** (5.169) |
13.858** (5.875) |
19.144*** (6.865) |
24.368*** (8.278) |
23.737*** (7.804) |
24.477*** (8.113) |
15.692* (8.431) |
16.828 (10.535) |
19.408* (10.209) |
|
Bank | 12.680** (5.097) |
12.776* (6.416) |
16.865** (7.559) |
18.772** (8.978) |
23.911** (9.881) |
24.501** (9.957) |
26.802** (10.589) |
24.033** (11.425) |
26.573* (13.561) |
27.253** (13.564) |
|
SMEborrower x bank | −11.522** (5.341) |
−17.121** (6.520) |
−16.530** (7.199) |
−19.663** (8.352) |
−24.016** (9.554) |
−21.761** (9.265) |
−22.498** (9.934) |
−13.313 (10.130) |
−14.372 (12.013) |
−15.876 (11.762) |
|
Constant | −4.246 (3.986) |
−4.588 (5.438) |
−8.846 (6.483) |
−13.438* (7.980) |
−18.973** (8.954) |
−19.666** (8.879) |
−21.053** (9.469) |
−18.464* (10.247) |
−21.887* (12.616) |
−22.883* (12.624) |
|
No of obs | 99 | 99 | 99 | 99 | 99 | 99 | 99 | 99 | 97 | 97 | |
R2 | 0.031 | 0.026 | 0.034 | 0.038 | 0.055 | 0.053 | 0.059 | 0.053 | 0.054 | 0.054 | |
R2 adj | 0.001 | −0.005 | 0.003 | 0.008 | 0.025 | 0.023 | 0.029 | 0.023 | 0.023 | 0.023 | |
2021 | |||||||||||
Jan | Feb | Mar | Apr | May | Jun | Jul | Aug | Sep | Oct | ||
SME borrower | 20.970** (10.216) |
20.763* (10.529) |
22.302** (10.820) |
20.811* (11.946) |
20.259 (12.108) |
23.533 (14.313) |
19.820 (13.637) |
16.020 (14.256) |
17.156 (13.541) |
18.480 (13.509) |
|
Bank | 28.993** (14.025) |
29.559** (14.541) |
37.323** (16.586) |
28.486 (17.602) |
22.051 (16.208) |
22.798 (18.453) |
21.207 (19.315) |
18.956 (19.824) |
20.527 (19.416) |
22.170 (19.462) |
|
SMEborrower x bank | −18.363 (11.900) |
−16.688 (12.244) |
−17.010 (12.850) |
−17.142 (13.841) |
−26.727* (13.486) |
−26.760 (15.974) |
−14.305 (18.245) |
−9.666 (18.715) |
−12.290 (17.953) |
−13.670 (17.967) |
|
Constant | −24.349* (13.031) |
−24.409* (13.545) |
−29.736* (15.579) |
−18.107 (15.455) |
−16.964 (15.699) |
−19.488 (17.990) |
−16.642 (18.823) |
−14.847 (19.387) |
−15.961 (18.961) |
−17.102 (18.965) |
|
No of obs | 97 | 96 | 96 | 94 | 72 | 73 | 72 | 73 | 73 | 73 | |
R2 | 0.054 | 0.058 | 0.078 | 0.028 | 0.063 | 0.055 | 0.033 | 0.030 | 0.030 | 0.033 | |
R2 adj | 0.023 | 0.028 | 0.048 | −0.004 | 0.022 | 0.014 | −0.010 | −0.012 | −0.012 | −0.009 | |
Notes: ***, ** and * denote statistical significance at the 1, 5 and 10 per cent levels, respectively. Standard errors are in parentheses. Sources: APRA; Authors' calculations; RBA |
2020 | ||||||||||
---|---|---|---|---|---|---|---|---|---|---|
Mar | Apr | May | Jun | Jul | Aug | Sep | Oct | Nov | Dec | |
Bank | 14.282*** (5.140) |
10.478** (4.072) |
9.938** (4.065) |
12.825** (6.197) |
−1.875 (9.185) |
−0.956 (8.542) |
6.679 (6.473) |
5.086 (7.545) |
14.151** (6.955) |
1.930 (7.447) |
Constant | −1.324 (2.435) |
−6.279** (2.903) |
−5.771** (2.815) |
−2.660 (3.218) |
5.305 (8.328) |
4.530 (7.443) |
0.589 (4.604) |
−0.190 (5.720) |
−0.971 (3.816) |
3.680 (5.465) |
No of obs | 162 | 162 | 162 | 162 | 162 | 162 | 162 | 162 | 162 | 162 |
R2 | 0.024 | 0.029 | 0.025 | 0.014 | 0.000 | 0.000 | 0.005 | 0.002 | 0.014 | 0.000 |
R2 adj | 0.018 | 0.023 | 0.019 | 0.008 | −0.006 | −0.006 | −0.001 | −0.004 | 0.008 | −0.006 |
2021 | ||||||||||
Jan | Feb | Mar | Apr | May | Jun | Jul | Aug | Sep | Oct | |
Bank | 0.589 (8.639) |
0.660 (7.604) |
−0.472 (12.406) |
1.888 (9.626) |
9.760 (12.470) |
−2.876 (15.083) |
−1.003 (17.154) |
−5.111 (18.286) |
11.234 (21.462) |
−3.816 (16.638) |
Constant | 4.929 (6.628) |
5.014 (5.188) |
12.070 (10.216) |
6.261 (6.294) |
10.573 (8.412) |
17.416 (12.497) |
18.836 (14.621) |
22.898 (15.673) |
23.876 (15.064) |
21.975 (13.673) |
No of obs | 162 | 162 | 162 | 162 | 162 | 162 | 162 | 162 | 162 | 162 |
R2 | 0.000 | 0.000 | 0.000 | 0.000 | 0.003 | 0.000 | 0.000 | 0.001 | 0.001 | 0.000 |
R2 adj | −0.006 | −0.006 | −0.006 | −0.006 | −0.004 | −0.006 | −0.006 | −0.006 | −0.005 | −0.006 |
Notes: ***, ** and * denote statistical significance at the 1, 5 and 10 per cent levels, respectively. Standard errors are in parentheses. Sources: APRA; Authors' calculations; RBA |
2020 | ||||||||||
---|---|---|---|---|---|---|---|---|---|---|
Mar | Apr | May | Jun | Jul | Aug | Sep | Oct | Nov | Dec | |
Bank | 1.257 (4.747) |
−3.733 (6.962) |
1.385 (7.120) |
0.453 (7.765) |
5.363 (10.781) |
8.001 (11.200) |
9.723 (11.590) |
16.144* (9.423) |
17.947* (10.487) |
21.314 (14.593) |
Constant | 2.012 (2.567) |
8.382 (5.679) |
5.011 (5.800) |
5.706 (6.253) |
5.396 (7.880) |
4.071 (8.115) |
3.424 (8.423) |
−2.772 (4.908) |
−5.059 (6.428) |
−3.475 (8.189) |
No of obs | 52 | 52 | 52 | 52 | 52 | 52 | 52 | 52 | 51 | 51 |
R2 | 0.001 | 0.004 | 0.001 | 0.000 | 0.003 | 0.005 | 0.007 | 0.021 | 0.022 | 0.015 |
R2 adj | −0.019 | −0.016 | −0.019 | −0.020 | −0.017 | −0.015 | −0.013 | 0.001 | 0.002 | −0.005 |
2021 | ||||||||||
Jan | Feb | Mar | Apr | May | Jun | Jul | Aug | Sep | Oct | |
Bank | 20.574 (14.676) |
23.276 (15.249) |
31.366* (17.922) |
22.186 (16.662) |
15.806 (22.716) |
18.613 (24.801) |
31.556 (28.305) |
57.965 (51.156) |
59.414 (53.571) |
61.806 (55.666) |
Constant | −3.379 (8.272) |
−3.646 (8.468) |
−7.434 (11.731) |
2.704 (8.378) |
3.295 (8.517) |
4.045 (8.570) |
3.178 (9.128) |
1.172 (9.984) |
1.195 (10.019) |
1.378 (10.081) |
No of obs | 51 | 50 | 50 | 49 | 36 | 36 | 36 | 36 | 36 | 36 |
R2 | 0.014 | 0.017 | 0.026 | 0.011 | 0.005 | 0.006 | 0.013 | 0.013 | 0.012 | 0.012 |
R2 adj | −0.006 | −0.004 | 0.006 | −0.010 | −0.024 | −0.023 | −0.016 | −0.016 | −0.017 | −0.017 |
Notes: ***, ** and * denote statistical significance at the 1, 5 and 10 per cent levels, respectively. Standard errors are in parentheses. Sources: APRA; Authors' calculations; RBA |
2020 | ||||||||||
---|---|---|---|---|---|---|---|---|---|---|
Mar | Apr | May | Jun | Jul | Aug | Sep | Oct | Nov | Dec | |
Bank | 11.282** (4.908) |
15.906** (6.657) |
19.013** (7.403) |
20.379** (8.456) |
24.704*** (9.073) |
25.299*** (9.143) |
27.405*** (9.680) |
24.940** (10.504) |
27.524** (12.217) |
28.269** (12.200) |
Constant | −2.778 (3.801) |
−7.927 (5.787) |
−11.747* (6.381) |
−15.968** (7.472) |
−20.855** (8.131) |
−21.414** (8.038) |
−22.497** (8.497) |
−20.449** (9.270) |
−24.071** (11.189) |
−24.998** (11.184) |
No of obs | 51 | 51 | 51 | 51 | 51 | 51 | 51 | 51 | 50 | 50 |
R2 | 0.051 | 0.082 | 0.090 | 0.091 | 0.122 | 0.113 | 0.117 | 0.088 | 0.095 | 0.101 |
R2 adj | 0.032 | 0.063 | 0.072 | 0.072 | 0.104 | 0.095 | 0.099 | 0.069 | 0.076 | 0.082 |
2021 | ||||||||||
Jan | Feb | Mar | Apr | May | Jun | Jul | Aug | Sep | Oct | |
Bank | 29.730** (12.583) |
30.421** (12.976) |
37.235** (14.672) |
28.251* (15.470) |
21.555 (13.914) |
21.921 (15.800) |
20.001 (16.537) |
17.421 (16.953) |
18.356 (16.630) |
19.844 (16.692) |
Constant | −26.305** (11.508) |
−26.069** (11.905) |
−30.578** (13.595) |
−18.815 (13.203) |
−17.711 (13.364) |
−19.943 (15.298) |
−16.921 (16.003) |
−14.652 (16.481) |
−15.022 (16.142) |
−15.764 (16.171) |
No of obs | 50 | 50 | 50 | 49 | 40 | 41 | 40 | 41 | 41 | 41 |
R2 | 0.102 | 0.104 | 0.129 | 0.039 | 0.101 | 0.090 | 0.072 | 0.055 | 0.062 | 0.069 |
R2 adj | 0.084 | 0.085 | 0.111 | 0.019 | 0.078 | 0.067 | 0.047 | 0.031 | 0.038 | 0.045 |
Notes: ***, ** and * denote statistical significance at the 1, 5 and 10 per cent levels, respectively. Standard errors are in parentheses. Sources: APRA; Authors' calculations; RBA |
2020 | ||||||||||
---|---|---|---|---|---|---|---|---|---|---|
Mar | Apr | May | Jun | Jul | Aug | Sep | Oct | Nov | Dec | |
Bank | 9.780 (8.031) |
8.988 (8.053) |
1.664 (3.673) |
8.805 (8.505) |
8.251 (8.709) |
7.707 (8.739) |
8.356 (8.877) |
1.833 (4.700) |
0.741 (5.708) |
0.181 (6.124) |
Constant | −5.442 (7.904) |
−6.291 (7.823) |
1.843 (3.078) |
−5.184 (8.207) |
−5.094 (8.387) |
−5.808 (8.444) |
−6.116 (8.436) |
0.031 (3.801) |
−0.483 (4.828) |
0.524 (5.108) |
No of obs | 45 | 45 | 45 | 45 | 45 | 45 | 45 | 45 | 44 | 44 |
R2 | 0.068 | 0.053 | 0.005 | 0.043 | 0.036 | 0.032 | 0.033 | 0.003 | 0.000 | 0.000 |
R2 adj | 0.047 | 0.031 | −0.018 | 0.021 | 0.014 | 0.010 | 0.010 | −0.020 | −0.023 | −0.024 |
2021 | ||||||||||
Jan | Feb | Mar | Apr | May | Jun | Jul | Aug | Sep | Oct | |
Bank | −0.323 (6.257) |
−0.696 (6.562) |
3.343 (10.124) |
−7.327 (6.093) |
−8.132 (6.261) |
−10.807 (6.556) |
−11.888 (7.422) |
−10.513 (8.481) |
−10.652 (8.836) |
−11.501 (9.081) |
Constant | 0.623 (5.346) |
0.916 (5.701) |
−2.612 (9.556) |
7.465 (5.020) |
8.403 (5.267) |
10.641* (5.540) |
11.597* (6.346) |
10.332 (7.589) |
10.925 (7.861) |
11.556 (8.114) |
No of obs | 44 | 44 | 44 | 43 | 42 | 43 | 42 | 43 | 43 | 43 |
R2 | 0.000 | 0.000 | 0.004 | 0.029 | 0.038 | 0.058 | 0.059 | 0.042 | 0.039 | 0.043 |
R2 adj | −0.024 | −0.024 | −0.020 | 0.006 | 0.014 | 0.035 | 0.036 | 0.019 | 0.015 | 0.020 |
Notes: ***, ** and * denote statistical significance at the 1, 5 and 10 per cent levels, respectively. Standard errors are in parentheses. Sources: APRA; Authors' calculations; RBA |
2020 | ||||||||||
---|---|---|---|---|---|---|---|---|---|---|
Mar | Apr | May | Jun | Jul | Aug | Sep | Oct | Nov | Dec | |
Bank | 4.332*** (1.544) |
5.564*** (1.483) |
5.859*** (1.871) |
5.337*** (1.954) |
4.522* (2.539) |
4.018 (2.688) |
4.170 (2.823) |
3.100 (2.811) |
2.998 (3.349) |
2.945 (3.426) |
Constant | 1.009 (1.245) |
−0.092 (1.248) |
−1.518 (1.702) |
−2.061 (1.829) |
−2.577* (2.397) |
−2.640 (2.540) |
−2.854 (2.648) |
−2.246 (2.606) |
−2.546 (3.166) |
−2.422 (3.220) |
No of obs | 162 | 162 | 162 | 162 | 162 | 162 | 162 | 162 | 162 | 162 |
R2 | 0.022 | 0.044 | 0.047 | 0.026 | 0.014 | 0.010 | 0.010 | 0.005 | 0.004 | 0.004 |
R2 adj | 0.016 | 0.038 | 0.041 | 0.020 | 0.008 | 0.004 | 0.004 | −0.001 | −0.002 | −0.003 |
2021 | ||||||||||
Jan | Feb | Mar | Apr | May | Jun | Jul | Aug | Sep | Oct | |
Bank | 2.962 (3.550) |
2.408 (3.787) |
5.603 (5.684) |
4.302 (5.902) |
2.953 (6.063) |
3.006 (6.296) |
4.106 (6.664) |
3.988 (7.212) |
4.090 (7.261) |
4.194 (7.380) |
Constant | −2.475 (3.294) |
−1.632 (3.485) |
−3.488 (5.448) |
−2.378 (5.632) |
−0.961 (5.775) |
0.564 (5.965) |
0.394 (6.347) |
0.904 (6.882) |
1.713 (6.922) |
2.107 (7.050) |
No of obs | 162 | 162 | 162 | 162 | 162 | 162 | 162 | 162 | 162 | 162 |
R2 | 0.003 | 0.002 | 0.007 | 0.004 | 0.002 | 0.001 | 0.002 | 0.002 | 0.002 | 0.002 |
R2 adj | −0.003 | −0.004 | 0.001 | −0.002 | −0.005 | −0.005 | −0.004 | −0.004 | −0.004 | −0.004 |
Notes: Weighted by each institution's level of business credit in the base period. ***, ** and * denote statistical significance at the 1, 5 and 10 per cent levels, respectively. Standard errors are in parentheses. Sources: APRA; Authors' calculations; RBA |
2020 | ||||||||||
---|---|---|---|---|---|---|---|---|---|---|
Mar | Apr | May | Jun | Jul | Aug | Sep | Oct | Nov | Dec | |
Bank | 5.941 (5.091) |
6.627 (4.933) |
2.418 (2.809) |
5.622 (5.518) |
4.871 (5.780) |
5.191 (5.914) |
4.941 (5.945) |
0.584 (3.812) |
1.254 (4.754) |
0.752 (5.004) |
Constant | −2.150 (5.078) |
−3.486 (4.903) |
0.511 (2.746) |
−2.604 (5.498) |
−2.580 (5.729) |
−3.280 (5.843) |
−3.535 (5.842) |
0.250 (3.617) |
−0.703 (4.574) |
0.366 (4.731) |
No of obs | 45 | 45 | 45 | 45 | 45 | 45 | 45 | 45 | 44 | 44 |
R2 | 0.045 | 0.059 | 0.018 | 0.034 | 0.022 | 0.024 | 0.017 | 0.000 | 0.001 | 0.000 |
R2 adj | 0.023 | 0.037 | −0.005 | 0.011 | 0.000 | 0.001 | −0.005 | −0.023 | −0.023 | −0.023 |
2021 | ||||||||||
Jan | Feb | Mar | Apr | May | Jun | Jul | Aug | Sep | Oct | |
Bank | 0.333 (5.246) |
−0.265 (5.574) |
3.928 (9.481) |
−6.600 (4.752) |
−7.874 (4.766) |
−8.804* (4.952) |
−9.196 (5.478) |
−7.435 (7.197) |
−7.763 (7.554) |
−8.078 (7.797) |
Constant | 0.622 (4.952) |
1.255 (5.272) |
−2.185 (9.267) |
8.321** (4.039) |
9.476** (4.193) |
11.941*** (4.331) |
13.206** (4.931) |
11.918* (6.708) |
12.951* (7.010) |
13.518* (7.194) |
No of obs | 44 | 44 | 44 | 43 | 42 | 43 | 42 | 43 | 43 | 43 |
R2 | 0.000 | 0.000 | 0.006 | 0.024 | 0.035 | 0.038 | 0.041 | 0.023 | 0.022 | 0.022 |
R2 adj | −0.024 | −0.024 | −0.017 | 0.000 | 0.011 | 0.014 | 0.018 | −0.001 | −0.002 | −0.002 |
Notes: Weighted by each institution's level of business credit in the base period. ***, ** and * denote statistical significance at the 1, 5 and 10 per cent levels, respectively. Standard errors are in parentheses. Sources: APRA; Authors' calculations; RBA |
2020 | ||||||||||||||||||
---|---|---|---|---|---|---|---|---|---|---|---|---|---|---|---|---|---|---|
Mar | Apr | May | Jun | Jul | Aug | Sep | Oct | Nov | Dec | |||||||||
Accessed TFF | −28.358* (15.274) |
4.467 (5.855) |
4.191 (6.135) |
−15.265 (16.060) |
7.140 (7.068) |
5.845 (8.082) |
−0.730 (6.917) |
8.755 (8.910) |
−22.048 (15.489) |
5.642 (8.706) |
||||||||
Constant | 32.773** (14.987) |
−0.960 (4.961) |
−0.893 (5.269) |
18.230 (15.655) |
−5.522 (6.216) |
−4.563 (7.206) |
3.674 (5.395) |
−5.935 (7.674) |
25.700* (14.795) |
−2.661 (7.516) |
||||||||
No of obs | 111 | 111 | 111 | 111 | 111 | 111 | 111 | 111 | 111 | 111 | ||||||||
R2 | 0.068 | 0.005 | 0.004 | 0.017 | 0.010 | 0.005 | 0.000 | 0.009 | 0.032 | 0.004 | ||||||||
R2 adj | 0.059 | −0.004 | −0.005 | 0.008 | 0.001 | −0.004 | −0.009 | 0.000 | 0.023 | −0.005 | ||||||||
2021 | ||||||||||||||||||
Jan | Feb | Mar | Apr | May | Jun | Jul | Aug | Sep | Oct | |||||||||
Accessed TFF | 4.625 (9.100) |
5.616 (8.672) |
−7.653 (11.373) |
7.921 (9.480) |
−17.036 (19.010) |
4.885 (10.517) |
−3.019 (12.736) |
3.828 (11.669) |
−60.206 (48.357) |
6.370 (9.966) |
||||||||
Constant | −2.243 (7.888) |
−2.869 (7.325) |
11.627 (10.304) |
−3.721 (8.107) |
26.961 (17.702) |
4.616 (8.214) |
13.725 (10.682) |
7.955 (9.170) |
73.239 (47.790) |
6.302 (6.997) |
||||||||
No of obs | 111 | 111 | 111 | 111 | 111 | 111 | 111 | 111 | 111 | 111 | ||||||||
R2 | 0.002 | 0.004 | 0.005 | 0.006 | 0.011 | 0.001 | 0.000 | 0.001 | 0.034 | 0.002 | ||||||||
R2 adj | −0.007 | −0.006 | −0.004 | −0.003 | 0.002 | −0.008 | −0.009 | −0.008 | 0.026 | −0.007 | ||||||||
Notes: ***, ** and * denote statistical significance at the 1, 5 and 10 per cent levels, respectively. Standard errors are in parentheses. Sources: APRA; Authors' calculations; RBA |
2020 | ||||||||||
---|---|---|---|---|---|---|---|---|---|---|
Mar | Apr | May | Jun | Jul | Aug | Sep | Oct | Nov | Dec | |
Self-securitisation | 0.387*** (0.065) |
0.387*** (0.065) |
0.387*** (0.065) |
0.387*** (0.065) |
0.387*** (0.065) |
0.387*** (0.065) |
0.387*** (0.065) |
0.387*** (0.065) |
0.387*** (0.065) |
0.387*** (0.065) |
Constant | 0.591*** (0.061) |
0.591*** (0.061) |
0.591*** (0.061) |
0.591*** (0.061) |
0.591*** (0.061) |
0.591*** (0.061) |
0.591*** (0.061) |
0.591*** (0.061) |
0.591*** (0.061) |
0.591*** (0.061) |
No of obs | 111 | 111 | 111 | 111 | 111 | 111 | 111 | 111 | 111 | 111 |
R2 | 0.055 | −0.030 | −0.032 | 0.004 | −0.007 | −0.009 | −0.010 | −0.003 | 0.014 | −0.023 |
R2 adj | 0.047 | −0.039 | −0.041 | −0.005 | −0.016 | −0.018 | −0.019 | −0.012 | 0.005 | −0.033 |
2021 | ||||||||||
Jan | Feb | Mar | Apr | May | Jun | Jul | Aug | Sep | Oct | |
Self-securitisation | 0.387*** (0.065) |
0.387*** (0.065) |
0.387*** (0.065) |
0.387*** (0.065) |
0.387*** (0.065) |
0.387*** (0.065) |
0.387*** (0.065) |
0.387*** (0.065) |
0.387*** (0.065) |
0.387*** (0.065) |
Constant | 0.591*** (0.061) |
0.591*** (0.061) |
0.591*** (0.061) |
0.591*** (0.061) |
0.591*** (0.061) |
0.591*** (0.061) |
0.591*** (0.061) |
0.591*** (0.061) |
0.591*** (0.061) |
0.591*** (0.061) |
No of obs | 111 | 111 | 111 | 111 | 111 | 111 | 111 | 111 | 111 | 111 |
R2 | −0.017 | −0.012 | −0.003 | −0.001 | 0.008 | −0.009 | −0.003 | −0.002 | 0.034 | 0.002 |
R2 adj | −0.026 | −0.021 | −0.012 | −0.010 | −0.001 | −0.018 | −0.012 | −0.011 | 0.026 | −0.007 |
Notes: ***, ** and * denote statistical significance at the 1, 5 and 10 per cent levels, respectively. Standard errors are in parentheses. Sources: APRA; Authors' calculations; RBA |
2020 | ||||||||||
---|---|---|---|---|---|---|---|---|---|---|
Mar | Apr | May | Jun | Jul | Aug | Sep | Oct | Nov | Dec | |
Accessed TFF (fitted) | −40.491* (21.424) |
−7.406 (15.221) |
−8.117 (15.373) |
−28.581 (23.735) |
−2.165 (16.219) |
−3.706 (17.851) |
−9.249 (20.175) |
−1.470 (21.981) |
−38.532 (26.217) |
−9.455 (20.982) |
Constant | 41.846** (18.280) |
7.918 (10.652) |
8.311 (10.946) |
28.187 (19.858) |
1.436 (12.031) |
2.579 (13.338) |
10.044 (13.945) |
1.711 (15.613) |
38.025* (20.925) |
8.627 (15.443) |
No of obs | 111 | 111 | 111 | 111 | 111 | 111 | 111 | 111 | 111 | 111 |
R2 | 0.055 | −0.030 | −0.032 | 0.004 | −0.007 | −0.009 | −0.010 | −0.003 | 0.014 | −0.023 |
R2 adj | 0.047 | −0.039 | −0.041 | −0.005 | −0.016 | −0.018 | −0.019 | −0.012 | 0.005 | −0.033 |
2021 | ||||||||||
Jan | Feb | Mar | Apr | May | Jun | Jul | Aug | Sep | Oct | |
Accessed TFF (fitted) | −8.530 (21.713) |
−6.195 (21.783) |
−17.131 (23.682) |
−0.628 (22.935) |
−8.251 (36.674) |
17.750 (30.313) |
5.394 (32.512) |
11.129 (33.023) |
−62.898 (61.501) |
3.757 (31.062) |
Constant | 7.594 (15.938) |
5.962 (15.877) |
18.715 (18.053) |
2.672 (16.977) |
20.392 (27.675) |
−5.003 (21.248) |
7.435 (23.373) |
2.495 (23.380) |
75.252 (55.635) |
8.256 (22.333) |
No of obs | 111 | 111 | 111 | 111 | 111 | 111 | 111 | 111 | 111 | 111 |
R2 | −0.017 | −0.012 | −0.003 | −0.001 | 0.008 | −0.009 | −0.003 | −0.002 | 0.034 | 0.002 |
R2 adj | −0.026 | −0.021 | −0.012 | −0.010 | −0.001 | −0.018 | −0.012 | −0.011 | 0.026 | −0.007 |
Notes: ***, ** and * denote statistical significance at the 1, 5 and 10 per cent levels, respectively. Standard errors are in parentheses. Sources: APRA; Authors' calculations; RBA |
2020 | ||||||||||
---|---|---|---|---|---|---|---|---|---|---|
Mar | Apr | May | Jun | Jul | Aug | Sep | Oct | Nov | Dec | |
Self-securitisation adequacy | 0.001*** (0.000) |
0.001*** (0.000) |
0.001*** (0.000) |
0.001*** (0.000) |
0.001*** (0.000) |
0.001*** (0.000) |
0.001*** (0.000) |
0.001*** (0.000) |
0.001*** (0.000) |
0.001*** (0.000) |
Constant | 0.651*** (0.054) |
0.651*** (0.054) |
0.651*** (0.054) |
0.651*** (0.054) |
0.651*** (0.054) |
0.651*** (0.054) |
0.651*** (0.054) |
0.651*** (0.054) |
0.651*** (0.054) |
0.651*** (0.054) |
No of obs | 111 | 111 | 111 | 111 | 111 | 111 | 111 | 111 | 111 | 111 |
R2 | 0.068 | 0.003 | 0.000 | 0.017 | 0.010 | 0.005 | −0.006 | 0.004 | 0.032 | 0.003 |
R2 adj | 0.059 | −0.006 | −0.010 | 0.008 | 0.001 | −0.004 | −0.016 | −0.005 | 0.023 | −0.006 |
2021 | ||||||||||
Jan | Feb | Mar | Apr | May | Jun | Jul | Aug | Sep | Oct | |
Self-securitisation adequacy | 0.001*** (0.000) |
0.001*** (0.000) |
0.001*** (0.000) |
0.001*** (0.000) |
0.001*** (0.000) |
0.001*** (0.000) |
0.001*** (0.000) |
0.001*** (0.000) |
0.001*** (0.000) |
0.001*** (0.000) |
Constant | 0.651*** (0.054) |
0.651*** (0.054) |
0.651*** (0.054) |
0.651*** (0.054) |
0.651*** (0.054) |
0.651*** (0.054) |
0.651*** (0.054) |
0.651*** (0.054) |
0.651*** (0.054) |
0.651*** (0.054) |
No of obs | 111 | 111 | 111 | 111 | 111 | 111 | 111 | 111 | 111 | 111 |
R2 | 0.002 | 0.003 | 0.005 | 0.006 | −0.017 | −0.067 | −0.039 | −0.036 | 0.033 | −0.011 |
R2 adj | −0.007 | −0.006 | −0.004 | −0.004 | −0.027 | −0.077 | −0.048 | −0.045 | 0.024 | −0.020 |
Notes: ***, ** and * denote statistical significance at the 1, 5 and 10 per cent levels, respectively. Standard errors are in parentheses. Sources: APRA; Authors' calculations; RBA |
2020 | ||||||||||
---|---|---|---|---|---|---|---|---|---|---|
Mar | Apr | May | Jun | Jul | Aug | Sep | Oct | Nov | Dec | |
Accessed TFF (fitted) | −29.084 (33.799) |
1.830 (30.192) |
−0.227 (29.946) |
−18.458 (38.641) |
7.332 (29.346) |
7.720 (30.365) |
6.023 (37.529) |
14.948 (40.095) |
−21.898 (42.401) |
2.811 (34.362) |
Constant | 33.316 (25.912) |
1.012 (21.104) |
2.410 (21.040) |
20.617 (29.205) |
−5.665 (20.935) |
−5.965 (21.777) |
−1.376 (26.184) |
−10.565 (28.206) |
25.588 (31.376) |
−0.544 (24.509) |
No of obs | 111 | 111 | 111 | 111 | 111 | 111 | 111 | 111 | 111 | 111 |
R2 | 0.068 | 0.003 | 0.000 | 0.017 | 0.010 | 0.005 | −0.006 | 0.004 | 0.032 | 0.003 |
R2 adj | 0.059 | −0.006 | −0.010 | 0.008 | 0.001 | −0.004 | −0.016 | −0.005 | 0.023 | −0.006 |
2021 | ||||||||||
Jan | Feb | Mar | Apr | May | Jun | Jul | Aug | Sep | Oct | |
Accessed TFF (fitted) | 2.071 (34.753) |
5.080 (34.162) |
−6.418 (35.079) |
10.321 (34.240) |
10.536 (51.628) |
38.490 (45.925) |
24.805 (48.804) |
30.729 (49.448) |
−46.800 (72.725) |
21.815 (47.162) |
Constant | −0.333 (24.799) |
−2.468 (24.342) |
10.704 (25.650) |
−5.515 (24.662) |
6.344 (37.838) |
−20.512 (32.468) |
−7.080 (34.800) |
−12.161 (34.981) |
63.215 (62.267) |
−5.247 (33.529) |
No of obs | 111 | 111 | 111 | 111 | 111 | 111 | 111 | 111 | 111 | 111 |
R2 | 0.002 | 0.003 | 0.005 | 0.006 | −0.017 | −0.067 | −0.039 | −0.036 | 0.033 | −0.011 |
R2 adj | −0.007 | −0.006 | −0.004 | −0.004 | −0.027 | −0.077 | −0.048 | −0.045 | 0.024 | −0.020 |
Notes: ***, ** and * denote statistical significance at the 1, 5 and 10 per cent levels, respectively. Standard errors are in parentheses. Sources: APRA; Authors' calculations; RBA |
2020 | ||||||||||
---|---|---|---|---|---|---|---|---|---|---|
Mar | Apr | May | Jun | Jul | Aug | Sep | Oct | Nov | Dec | |
Self-securitisation dummy | 0.304*** (0.099) |
0.304*** (0.099) |
0.304*** (0.099) |
0.304*** (0.099) |
0.304*** (0.099) |
0.304*** (0.099) |
0.304*** (0.099) |
0.304*** (0.099) |
0.304*** (0.099) |
0.304*** (0.099) |
Constant | 0.696*** (0.099) |
0.696*** (0.099) |
0.696*** (0.099) |
0.696*** (0.099) |
0.696*** (0.099) |
0.696*** (0.099) |
0.696*** (0.099) |
0.696*** (0.099) |
0.696*** (0.099) |
0.696*** (0.099) |
No of obs | 32 | 32 | 32 | 32 | 32 | 32 | 32 | 32 | 32 | 32 |
R2 | 0.072 | 0.091 | 0.057 | −0.046 | −0.033 | 0.002 | −0.011 | 0.010 | 0.014 | 0.013 |
R2 adj | 0.041 | 0.061 | 0.026 | −0.081 | −0.068 | −0.031 | −0.045 | −0.023 | −0.019 | −0.020 |
2021 | ||||||||||
Jan | Feb | Mar | Apr | May | Jun | Jul | Aug | Sep | Oct | |
Self-securitisation dummy | 0.304*** (0.099) |
0.304*** (0.099) |
0.304*** (0.099) |
0.304*** (0.099) |
0.273*** (0.098) |
0.304*** (0.099) |
0.273*** (0.098) |
0.304*** (0.099) |
0.304*** (0.099) |
0.304*** (0.099) |
Constant | 0.696*** (0.099) |
0.696*** (0.099) |
0.696*** (0.099) |
0.696*** (0.099) |
0.727*** (0.098) |
0.696*** (0.099) |
0.727*** (0.098) |
0.696*** (0.099) |
0.696*** (0.099) |
0.696*** (0.099) |
No of obs | 32 | 32 | 32 | 32 | 31 | 32 | 31 | 32 | 32 | 32 |
R2 | 0.046 | 0.049 | 0.077 | 0.075 | 0.094 | 0.102 | 0.106 | 0.100 | 0.051 | 0.032 |
R2 adj | 0.014 | 0.018 | 0.046 | 0.045 | 0.063 | 0.073 | 0.076 | 0.070 | 0.019 | 0.000 |
Notes: ***, ** and * denote statistical significance at the 1, 5 and 10 per cent levels, respectively. Standard errors are in parentheses. Sources: APRA; Authors' calculations; RBA |
2020 | ||||||||||
---|---|---|---|---|---|---|---|---|---|---|
Mar | Apr | May | Jun | Jul | Aug | Sep | Oct | Nov | Dec | |
Accessed TFF (fitted) | −12.044* (6.586) |
−5.219 (8.937) |
−9.032 (9.289) |
−8.774 (11.078) |
−7.687 (11.720) |
1.202 (11.242) |
−1.313 (13.505) |
1.592 (13.438) |
8.749 (14.794) |
8.161 (16.537) |
Constant | 13.747** (6.431) |
6.774 (8.625) |
10.563 (9.101) |
10.475 (10.759) |
9.162 (11.294) |
0.960 (10.727) |
3.266 (13.100) |
0.620 (12.989) |
−6.576 (14.286) |
−5.671 (15.904) |
No of obs | 32 | 32 | 32 | 32 | 32 | 32 | 32 | 32 | 32 | 32 |
R2 | 0.072 | 0.091 | 0.057 | −0.046 | −0.033 | 0.002 | −0.011 | 0.010 | 0.014 | 0.013 |
R2 adj | 0.041 | 0.061 | 0.026 | −0.081 | −0.068 | −0.031 | −0.045 | −0.023 | −0.019 | −0.020 |
2021 | ||||||||||
Jan | Feb | Mar | Apr | May | Jun | Jul | Aug | Sep | Oct | |
Accessed TFF (fitted) | 9.278 (15.841) |
10.785 (15.848) |
12.732 (16.000) |
14.255 (16.366) |
12.930 (18.009) |
16.388 (17.037) |
21.508 (20.815) |
21.571 (17.996) |
20.637 (19.556) |
24.918 (19.821) |
Constant | −6.948 (15.126) |
−8.206 (15.111) |
−9.215 (15.289) |
−10.998 (15.729) |
−10.157 (17.334) |
−12.969 (16.005) |
−17.636 (19.789) |
−17.033 (17.136) |
−15.849 (18.697) |
−19.412 (19.021) |
No of obs | 32 | 32 | 32 | 32 | 31 | 32 | 31 | 32 | 32 | 32 |
R2 | 0.046 | 0.049 | 0.077 | 0.075 | 0.094 | 0.102 | 0.106 | 0.100 | 0.051 | 0.032 |
R2 adj | 0.014 | 0.018 | 0.046 | 0.045 | 0.063 | 0.073 | 0.076 | 0.070 | 0.019 | 0.000 |
Notes: ***, ** and * denote statistical significance at the 1, 5 and 10 per cent levels, respectively. Standard errors are in parentheses. Sources: APRA; Authors' calculations; RBA |
2020 | ||||||||||
---|---|---|---|---|---|---|---|---|---|---|
Mar | Apr | May | Jun | Jul | Aug | Sep | Oct | Nov | Dec | |
Self-securitisation adequacy | 0.002*** (0.001) |
0.002*** (0.001) |
0.002*** (0.001) |
0.002*** (0.001) |
0.002*** (0.001) |
0.002*** (0.001) |
0.002*** (0.001) |
0.002*** (0.001) |
0.002*** (0.001) |
0.002*** (0.001) |
Constant | 0.701*** (0.097) |
0.701*** (0.097) |
0.701*** (0.097) |
0.701*** (0.097) |
0.701*** (0.097) |
0.701*** (0.097) |
0.701*** (0.097) |
0.701*** (0.097) |
0.701*** (0.097) |
0.701*** (0.097) |
No of obs | 32 | 32 | 32 | 32 | 32 | 32 | 32 | 32 | 32 | 32 |
R2 | 0.077 | 0.093 | 0.053 | −0.051 | −0.044 | 0.001 | −0.027 | 0.002 | 0.018 | 0.015 |
R2 adj | 0.046 | 0.063 | 0.021 | −0.086 | −0.078 | −0.033 | −0.061 | −0.031 | −0.015 | −0.017 |
2021 | ||||||||||
Jan | Feb | Mar | Apr | May | Jun | Jul | Aug | Sep | Oct | |
Self-securitisation adequacy | 0.002*** (0.001) |
0.002*** (0.001) |
0.002*** (0.001) |
0.002*** (0.001) |
0.002*** (0.001) |
0.002*** (0.001) |
0.002*** (0.001) |
0.002*** (0.001) |
0.002*** (0.001) |
0.002*** (0.001) |
Constant | 0.701*** (0.097) |
0.701*** (0.097) |
0.701*** (0.097) |
0.701*** (0.097) |
0.733*** (0.096) |
0.701*** (0.097) |
0.733*** (0.096) |
0.701*** (0.097) |
0.701*** (0.097) |
0.701*** (0.097) |
No of obs | 32 | 32 | 32 | 32 | 31 | 32 | 31 | 32 | 32 | 32 |
R2 | 0.044 | 0.050 | 0.076 | 0.076 | 0.088 | 0.103 | 0.111 | 0.107 | 0.062 | 0.054 |
R2 adj | 0.012 | 0.018 | 0.045 | 0.046 | 0.057 | 0.073 | 0.081 | 0.077 | 0.031 | 0.023 |
Notes: ***, ** and * denote statistical significance at the 1, 5 and 10 per cent levels, respectively. Standard errors are in parentheses. Sources: APRA; Authors' calculations; RBA |
2020 | ||||||||||
---|---|---|---|---|---|---|---|---|---|---|
Mar | Apr | May | Jun | Jul | Aug | Sep | Oct | Nov | Dec | |
Accessed TFF (fitted) | −11.877* (6.604) |
−5.455 (8.852) |
−9.690 (9.254) |
−9.119 (11.053) |
−8.476 (11.782) |
0.196 (11.284) |
−2.758 (13.804) |
0.248 (13.750) |
7.402 (15.030) |
6.488 (16.838) |
Constant | 13.617* (6.437) |
6.959 (8.574) |
11.076 (9.093) |
10.745 (10.757) |
9.778 (11.355) |
1.746 (10.759) |
4.395 (13.317) |
1.670 (13.196) |
−5.524 (14.415) |
−4.364 (16.074) |
No of obs | 32 | 32 | 32 | 32 | 32 | 32 | 32 | 32 | 32 | 32 |
R2 | 0.077 | 0.093 | 0.053 | −0.051 | −0.044 | 0.001 | −0.027 | 0.002 | 0.018 | 0.015 |
R2 adj | 0.046 | 0.063 | 0.021 | −0.086 | −0.078 | −0.033 | −0.061 | −0.031 | −0.015 | −0.017 |
2021 | ||||||||||
Jan | Feb | Mar | Apr | May | Jun | Jul | Aug | Sep | Oct | |
Accessed TFF (fitted) | 7.556 (16.231) |
8.944 (16.257) |
10.727 (16.519) |
12.437 (16.963) |
10.500 (18.939) |
14.073 (17.710) |
18.625 (21.350) |
18.975 (18.652) |
17.567 (20.212) |
21.208 (20.269) |
Constant | −5.603 (15.365) |
−6.767 (15.356) |
−7.649 (15.604) |
−9.578 (16.078) |
−8.197 (17.944) |
−11.160 (16.410) |
−15.311 (20.114) |
−15.005 (17.498) |
−13.451 (19.042) |
−16.513 (19.452) |
No of obs | 32 | 32 | 32 | 32 | 31 | 32 | 31 | 32 | 32 | 32 |
R2 | 0.044 | 0.050 | 0.076 | 0.076 | 0.088 | 0.103 | 0.111 | 0.107 | 0.062 | 0.054 |
R2 adj | 0.012 | 0.018 | 0.045 | 0.046 | 0.057 | 0.073 | 0.081 | 0.077 | 0.031 | 0.023 |
Notes: ***, ** and * denote statistical significance at the 1, 5 and 10 per cent levels, respectively. Standard errors are in parentheses. APRA; Authors' calculations; RBA |
2020 | ||||||||||
---|---|---|---|---|---|---|---|---|---|---|
Mar | Apr | May | Jun | Jul | Aug | Sep | Oct | Nov | Dec | |
Bank | 0.728*** (0.042) |
0.728*** (0.042) |
0.728*** (0.042) |
0.728*** (0.042) |
0.728*** (0.042) |
0.728*** (0.042) |
0.728*** (0.042) |
0.728*** (0.042) |
0.728*** (0.042) |
0.728*** (0.042) |
Constant | 0.000 (0.000) |
0.000 (0.000) |
0.000 (0.000) |
0.000 (0.000) |
0.000 (0.000) |
0.000 (0.000) |
0.000 (0.000) |
0.000 (0.000) |
0.000 (0.000) |
0.000 (0.000) |
No of obs | 162 | 162 | 162 | 162 | 162 | 162 | 162 | 162 | 162 | 162 |
R2 | −0.104 | −0.021 | −0.022 | −0.058 | 0.002 | 0.001 | −0.022 | −0.007 | −0.069 | −0.003 |
R2 adj | −0.111 | −0.027 | −0.028 | −0.064 | −0.004 | −0.005 | −0.029 | −0.013 | −0.075 | −0.009 |
2021 | ||||||||||
Jan | Feb | Mar | Apr | May | Jun | Jul | Aug | Sep | Oct | |
Bank | 0.728*** (0.042) |
0.728*** (0.042) |
0.728*** (0.042) |
0.728*** (0.042) |
0.728*** (0.042) |
0.728*** (0.042) |
0.728*** (0.042) |
0.728*** (0.042) |
0.728*** (0.042) |
0.728*** (0.042) |
Constant | 0.000 (0.000) |
0.000 (0.000) |
0.000 (0.000) |
0.000 (0.000) |
0.000 (0.000) |
0.000 (0.000) |
0.000 (0.000) |
0.000 (0.000) |
0.000 (0.000) |
0.000 (0.000) |
No of obs | 162 | 162 | 162 | 162 | 162 | 162 | 162 | 162 | 162 | 162 |
R2 | −0.001 | −0.001 | 0.001 | −0.002 | −0.019 | 0.003 | 0.001 | 0.004 | −0.016 | 0.003 |
R2 adj | −0.007 | −0.007 | −0.005 | −0.009 | −0.026 | −0.004 | −0.005 | −0.002 | −0.023 | −0.003 |
Notes: ***, ** and * denote statistical significance at the 1, 5 and 10 per cent levels, respectively. Standard errors are in parentheses. Sources: APRA; Authors' calculations; RBA |
2020 | ||||||||||
---|---|---|---|---|---|---|---|---|---|---|
Mar | Apr | May | Jun | Jul | Aug | Sep | Oct | Nov | Dec | |
Accessed TFF (fitted) | 19.616*** (7.431) |
14.392** (5.675) |
13.650** (5.664) |
17.615** (8.751) |
−2.576 (12.612) |
−1.313 (11.730) |
9.173 (8.960) |
6.986 (10.387) |
19.436* (9.850) |
2.651 (10.238) |
Constant | −1.324 (2.435) |
−6.279** (2.903) |
−5.771** (2.815) |
−2.660 (3.218) |
5.305 (8.328) |
4.530 (7.443) |
0.589 (4.604) |
−0.190 (5.720) |
−0.971 (3.816) |
3.680 (5.465) |
No of obs | 162 | 162 | 162 | 162 | 162 | 162 | 162 | 162 | 162 | 162 |
R2 | −0.104 | −0.021 | −0.022 | −0.058 | 0.002 | 0.001 | −0.022 | −0.007 | −0.069 | −0.003 |
R2 adj | −0.111 | −0.027 | −0.028 | −0.064 | −0.004 | −0.005 | −0.029 | −0.013 | −0.075 | −0.009 |
2021 | ||||||||||
Jan | Feb | Mar | Apr | May | Jun | Jul | Aug | Sep | Oct | |
Accessed TFF (fitted) | 0.809 (11.868) |
0.906 (10.447) |
−0.648 (17.035) |
2.593 (13.232) |
13.405 (17.244) |
−3.951 (20.705) |
−1.378 (23.556) |
−7.019 (25.098) |
15.430 (29.631) |
−5.241 (22.838) |
Constant | 4.929 (6.628) |
5.014 (5.188) |
12.070 (10.216) |
6.261 (6.294) |
10.573 (8.412) |
17.416 (12.497) |
18.836 (14.621) |
22.898 (15.673) |
23.876 (15.064) |
21.975 (13.673) |
No of obs | 162 | 162 | 162 | 162 | 162 | 162 | 162 | 162 | 162 | 162 |
R2 | −0.001 | −0.001 | 0.001 | −0.002 | −0.019 | 0.003 | 0.001 | 0.004 | −0.016 | 0.003 |
R2 adj | −0.007 | −0.007 | −0.005 | −0.009 | −0.026 | −0.004 | −0.005 | −0.002 | −0.023 | −0.003 |
Notes: ***, ** and * denote statistical significance at the 1, 5 and 10 per cent levels, respectively. Standard errors are in parentheses. Sources: APRA; Authors' calculations; RBA |
References
Afonso A and J Sousa-Leite (2020), ‘The Transmission of Unconventional Monetary Policy to Bank Credit Supply: Evidence from the TLTRO’, The Manchester School, 88(S1), pp 151–171.
Altavilla C, F Barbiero, M Boucinha and L Burlon (2020), ‘The Great Lockdown: Pandemic Response Policies and Bank Lending Conditions’, European Central Bank Working Paper Series No 2465.
Anbil S, M Carlson and M-F Styczynski (2021), ‘The Effect of the PPPLF on PPP Lending by Commercial Banks’, Board of Governors of the Federal Reserve System Finance and Economics Discussion Paper Series No 2021-030.
Anderson TW and H Rubin (1949), ‘Estimation of the Parameters of a Single Equation in a Complete System of Stochastic Equations’, The Annals of Mathematical Statistics, 20(1), pp 46–63.
Andreeva DC and M García-Posada (2021), ‘The Impact of the ECB's Targeted Long-Term Refinancing Operations on Banks’ Lending Policies: The Role of Competition', Journal of Banking & finance, 122, Article 105992.
Bank J and M Lewis (2021), ‘Australia's Economic Recovery and Access to Small Business Finance’, RBA Bulletin, March.
Benetton M and D Fantino (2021), ‘Targeted Monetary Policy and Bank Lending Behavior’, Journal of Financial Economics, 142(1), pp 404–429.
Berger D, N Turner and E Zwick (2020), ‘Stimulating Housing Markets’, Journal of Finance, 75(1), pp 277–321
Black S, B Jackman and C Schwartz (2021), ‘An Assessment of the Term Funding Facility’, RBA Bulletin, September.
Black S, K Lane and L Nunn (2021), ‘Small Business Finance and COVID-19 Outbreaks’, RBA Bulletin, September.
Cantú C, P Cavallino, F De Fiore and J Yetman (2021), ‘A Global Database on Central Banks’ Monetary Responses to Covid-19', BIS Working Paper No 934.
Churm R, M Joyce, G Kapetanios and K Theodoridis (2021), ‘Unconventional Monetary Policies and the Macroeconomy: The Impact of the UK's QE2 and Funding for Lending Scheme’, The Quarterly Review of Economics and Finance, 80, pp 721–736.
Da Silva E, V Grossmann-Wirth, B Nguyen and M Vari (2021), ‘Paying Banks to Lend? Evidence from the Eurosystem's TLTRO and the Euro Area Credit Registry’, Banque de France Working Paper No 848.
Doherty E, B Jackman and E Perry (2018), ‘Money in the Australian Economy’, RBA Bulletin, September.
Esposito L, D Fantino and Y Sung (2020), ‘The Impact of TLTRO2 on the Italian Credit Market: Some Econometric Evidence’, Banca D'Italia Temi di Discussione No 1264.
Havrylchyk O (2016), ‘Incentivising Lending to SMEs with the Funding for Lending Scheme: Some Evidence from Bank-Level Data in the United Kingdom’, OECD Economics Department Working Papers No 1365.
Johnson C, K Lane and N McClure (2022), ‘Australian Securities Markets through the COVID-19 Pandemic’, RBA Bulletin, March.
Kent C (2018), ‘Money – Born of Credit?’, Remarks at the Reserve Bank's Topical Talks Event for Educators, Sydney, 19 September.
Kent C (2021a), ‘Small Businesses Finance in the Pandemic’, Address to the Australian Finance Industry Association, Sydney, 17 March.
Kent C (2021b), ‘The Term Funding Facility, Other Policy Measures, and Financial Conditions’, Keynote Address to the KangaNews Debt Capital Markets Summit, Online, 9 June.
Khwaja AI and A Mian (2008), ‘Tracing the Impact of Bank Liquidity Shocks: Evidence from an Emerging Market’, The American Economic Review, 98(4), pp 1413–1442.
Kohler M (2021), ‘Australian Securities Markets Through the Pandemic’, Keynote Address to the Australian Securitisation Forum Virtual Conference 2021, Online, 23 November.
Kwapil C and K Rieder (2021), ‘The Effects of the Monetary Policy Response to the COVID-19 Pandemic: Preliminary Evidence from a Pilot Study Using Austrian Bank-Level Data’, Oesterreichische Nationalbank Monetary Policy & the Economy, Q4/20–Q1/21, pp 131–152.
Laine O-M (2021), ‘The Effect of Targeted Monetary Policy on Bank Lending’, Journal of Banking and Financial Economics, 1(15), pp 25–43.
Mian A and A Sufi (2012), ‘The Effects of Fiscal Stimulus: Evidence from the 2009 Cash for Clunkers Program’, The Quarterly Journal of Economics, 127(3), pp 1107–1142.
Minoiu C, R Zarutskie and A Zlate (2021), ‘Motivating Banks to Lend? Credit Spillover Effects of the Main Street Lending Program’, Board of Governors of the Federal Reserve System Finance and Economics Discussion Paper Series No 2021-078.
Acknowledgements
We are particularly grateful for James Bishop's feedback and technical help. We would also like to thank Ben Jackman, Carl Schwartz, Chris Kent, Claudia Kwapil, John Simon, Jonathan Kearns, Kilian Rieder, Marion Kohler, Susan Black and seminar participants at the Reserve Bank of Australia for helpful comments and discussion. The views expressed in this paper are those of the authors and do not necessarily reflect the views of the Reserve Bank of Australia. The authors are solely responsible for any errors.
Footnotes
This is because when bank bonds mature, banks repay bondholders by crediting their account with deposits. See Kent (2018) and Doherty, Jackman and Perry (2018). [1]
The size of the initial allowance was 3 per cent of credit before the outbreak of COVID-19. For banks reporting data on a monthly basis, this figure was based on the average of three months ending 31 January 2020; for banks reporting quarterly, the quarter ending 31 December 2019 was used. [2]
In contrast to repo funding available through the RBA in open market operations, banks could post self-securitisation notes as collateral for the TFF. Self-securitisation notes are structured pools of assets, such as their own residential mortgages, created by banks specifically to use as collateral to access liquidity from the RBA. The RBA accepts self-securitisations by banks to access liquidity through standing facilities, exceptional liquidity assistance and the TFF only. [3]
Whereas a bank's additional allowances varied with growth in their business credit during the drawdown period for the TFF, the value of each bank's additional allowance was fixed after the end of April 2021. As a result, a bank cannot be overdrawn in respect of their additional allowance due to a decline in their business credit outstanding after the end of the final reporting period. [4]
See Cantú et al (2021) for a database on central banks' monetary policy responses to COVID-19. [5]
TLTRO III was first introduced in 2019 and adapted in 2020 in response to the pandemic. Under TLTRO III, banks could borrow at a rate as low as –1 per cent if they exceed lending targets. [6]
Churm et al (2021) examine the macroeconomic effects of unconventional monetary policies in the United Kingdom, focusing on additional quantitative easing (QE2) and the Funding for Lending Scheme. They find positive effects on GDP and inflation. [7]
Balance sheet reporting is audited. All information provided by a bank under the balance sheet reporting standards must be the product of systems, processes and controls that have been reviewed and tested by an external auditor. Auditors must also provide attestations about the quality of reporting on business credit data. [8]
While we capture the broader range of channels through which the TFF may affect business lending, our approach does not capture general equilibrium effects. [9]
In the very short-term, some businesses did access additional credit to manage cash flow challenges associated with the JobKeeper payment being paid monthly in arrears. [10]
We run a test of prior trends, which uses a simple difference-in-difference model. In this model, our dependent variable is log credit and our coefficient of interest is the interaction between the relevant group variable (SME borrowers, lender type and lenders that accessed the TFF) and a linear period variable. We run this model using only data from before the treatment period (before April 2020) for our three base models, and generate coefficients that are not significantly different from zero. This suggests that our pre-trends are not materially different. [11]
Prior to the EFS collection, there was limited data on business credit outstanding by size reported by banks permitted to use an internal ratings-based approach for credit risk management. These data suggested that large business lending had been growing but SME lending had been flat for several years. [12]
This approach follows Berger, Turner and Zwick's (2020) evaluation of the First-Time Homebuyer Credit policy and Mian and Sufi's (2012) assessment of the Cash for Clunkers program. [13]
The TFF additional allowance encouraged lending to small businesses and medium businesses equally, so we should not expect to find a differential effect on credit to these business size types. [14]
As mentioned earlier, this model uses data on SME and large business credit outstanding reported by 15 banks directly to the RBA from November 2019 until April 2021. As such, results for our full sample of banks is only available to April 2021, rather than October 2021 as presented in most of our regressions. As additional allowances were finalised in April 2021, it is unlikely that they would continue to directly stimulate growth in business credit beyond this date. [15]
The model in Section 7 estimates an intention-to-treat effect, while this model is the average treatment effect on the treated (ATT). [16]
For simplicity we assign this a value of 1 for institutions that accessed the TFF by the end of the drawdown period (i.e. we do not identify whether an institution had accessed it by a particular time period). [17]
We considered a version of Equation (8) where the dependent variable was bank-level drawdowns of the TFF in value terms; this would have allowed us to evaluate the effect of actual drawdowns of the TFF (in contrast with simply accessing some level of funding through the TFF). However, the strength of self-securitised assets as an instrument was considerably weaker in this regression. [18]
However, more recent studies suggest that the threshold for instrument validity needs to be much higher, so it is possible that we still have a weak instrument problem. [19]
Anderson–Rubin confidence intervals, or weak instrument-robust confidence intervals, provide a valid measure of uncertainty even if our instruments are weak (Anderson and Rubin 1949). We use these confidence intervals for our instrumental variable analysis. [20]
The first instrumental variable regression, using self-securitisations, is intended to estimate the treatment effect among those banks that accessed the TFF only because they had self-securitisations on their balance sheet. The second instrumental variable regression captures the treatment effect for banks that accessed the TFF, regardless of whether or not they had self-securitisations. In other words, the set of ‘compliers’ is different between the two models. [21]
Examples include Boosting Cash Flows for Employers, enhancements to instant asset write-off and the SME loan guarantee (Bank and Lewis 2021; Black, Kane and Lane 2021; Kent 2021a). [22]
Under the EFS collection, businesses with turnover greater than or equal to $50 million are classified as large businesses. For businesses with turnover less than $50 million, when the lender has an exposure of more than $1 million, the business is classified as medium. When the exposure is less than $1 million, the business is classified as small. [23]
Data reported by these 15 banks are used in our baseline SME regression (Equation (1)) and our triple-difference regression (Equation (5)). As banks with less than $2 billion in business credit outstanding could choose whether to report SME and large business credit directly to the RBA, this poses a potential self-selection bias (as these banks may have been more likely to increase lending anyway). As a check, we ran these regressions without these 15 banks and also found no statistically significant effects of the TFF on business credit. [24]
This results in a break in two of our regression results (Equations (1) and (5)). In our coefficient figures, we have chosen to present the full set of reporters for a smaller period of time. Coefficient results up to October 2021 are presented in our regression tables in Appendix C, but include a series break at May 2021. [25]
These banks did not access the TFF and had very volatile growth rates. We also test the robustness of our results to outliers by running weighted regressions. [26]
Banks may reclassify loans between industries following data reviews. Grouping into broader industry groups may reduce the risk that reclassification leads to big movements in the data (if industries are more likely to be reclassified to other industries within the broad group). [27]
Lending to the following industries are excluded from our analysis: financial and insurance (lending to the RBA, authorised deposit-taking institutions, registered financial corporations and central borrowing authorities); and public administration and safety. [28]
This includes forms ARF 720_0 A/B, ARF 720_1 A/B, ARF 720_2 A/B, ARF 330_0_l, ARF 330_1_L, ARF 110_0_1 and ARF 323_0. [29]