Research Discussion Paper – RDP 2019-01 A Model of the Australian Housing Market
March 2019
1. Introduction
The Australian housing market shows strong relationships between interest rates, investment, rents and prices. This paper combines these relationships in one – hopefully realistic – model. The model provides internally consistent projections for housing construction, prices and rents. It estimates responses to interest rates, allowing for feedback between quantities and prices. It helps explain historical developments. It can inform housing policy and taxation policy.
These key relationships include:
- Interest rates, income and housing prices have strong and clear effects on residential construction.
- Dwelling completions and changes in population explain the rental vacancy rate.
- The vacancy rate has a strong and clear effect on rents.
- Interest rates, rents and momentum have large effects on housing prices.
- Housing prices and construction are mutually determined, so examining bivariate relationships in isolation can be misleading.
Some of these observations are not new. However, most have not been estimated or publicly documented for a long time, if at all. Nor have their interrelationships been explored. This paper aims to fill those gaps. In doing so, we re-examine these relationships in the light of recent research and data. This gives us a system of equations that, for the most part, fit the data fairly closely, are stable across time, and are consistent with theory and previous research. There are some exceptions to this, which we note below.
Our model quantifies some important developments:
- The model suggests that much of the strength in housing prices and construction over the past few years can be explained by the fall in interest rates – some of this fall reflects lower world real interest rates and some is cyclical.
- A large part of the effect of interest rates on dwelling investment (and hence real GDP) occurs through the channel of housing prices.
- The model suggests that an increase in population growth will reduce rental vacancies, boost rents and housing prices, and increase construction. This helps to explain developments following the immigration surge of the mid 2000s.
- The model is consistent with some important longer-run trends. Construction activity is approximately cointegrated (trends together) with income, although the housing stock is not. The rental yield is cointegrated with the user cost of housing. Rents tend to grow slightly faster than inflation but slower than income per capita.
2. Previous Research
Most empirical research into the Australian housing market has focused on individual equations. For example, Bourassa and Hendershott (1995), Abelson et al (2005) and Tumbarello and Wang (2010) examine housing prices; McLaughlin (2011) and Gitelman and Otto (2012) examine housing construction. The IMF (2018) estimate and discuss equations for both variables separately. Our approach differs in that we put these and other equations together. We also re-examine this earlier work in the light of recent research and data.
Another strand of research has examined the housing market as part of structural macroeconometric models. Examples include Jovanoski, Stoney and Downes (1997), Powell and Murphy (1997) or the Reserve Bank of Australia's (RBA's) new MARTIN model (Cusbert and Kendall 2018). Our approach differs in that we look at the housing market in more detail, including variables that these models often exclude, such as building approvals, completions or the vacancy rate. The macroeconomic models are designed to settle down to an explicit steady state with simple properties, such as constant relative prices or expenditure shares. That facilitates the models' application to a wide range of questions. However, in discussing housing-specific issues, it is not desirable to have important results driven by assumptions for which evidence is weak. We do not constrain the steady state of our model except when the data suggest this is realistic.
These alternative approaches have advantages relative to our approach. Larger models allow more variables to be endogenous, allow for feedback and can answer a wider range of questions. Smaller models are less reliant on chains of causation, which can be as fragile as their weakest link. And more focused models allow examination of specific estimates in more detail. These different approaches are complementary. When a range of different approaches support similar results, we have more confidence in the conclusions. Accordingly, we discuss specific points of agreement and disagreement where they arise in the discussion.
In common with most previous Australian research on housing markets, and most macroeconomic forecasting, we focus on single-equation least squares estimates using aggregate quarterly data. Identification is typically through lags and a priori reasoning. For example, rents are explained by lagged vacancies and contemporaneous income, on the assumption that the right-hand side variables are weakly exogenous. Given that the future does not determine the past, that strikes us as plausible for most lagged variables. This argument is less compelling when the right-hand side variables are forward-looking, such as investment or asset prices. However, we are not aware of clear evidence or strong arguments that weak exogeneity fails in the relevant equations or of useful instruments that might rectify this. One alternative to our approach would be to assume model-consistent expectations, but that seems unrealistic. We recognise that finding ‘X regularly precedes Y’ does not prove that ‘X causes Y’. However, in the absence of reasonable arguments to the contrary, the latter statement seems a natural hypothesis to maintain pending more definitive tests. This approach – essentially that of structural macroeconometric modelling – rests on methodological assumptions that are controversial, but that debate is outside the scope of this paper.
3. Overview of the Model
Our model takes income, interest rates, population, and depreciation as given. More precisely, these variables are modelled simply, with no feedback from the housing sector. We examine how these and other factors combine to influence construction activity, the housing stock, rents and housing prices. Figure 1 shows a stylised summary of our model, with a more detailed version presented in Online Appendix A. This framework is more detailed than, but not conceptually different to, the housing blocks of the Australian macroeconometric models cited above. Oxford Economics (2016, Figure 1) describe their model of the UK housing market with a similar diagram.
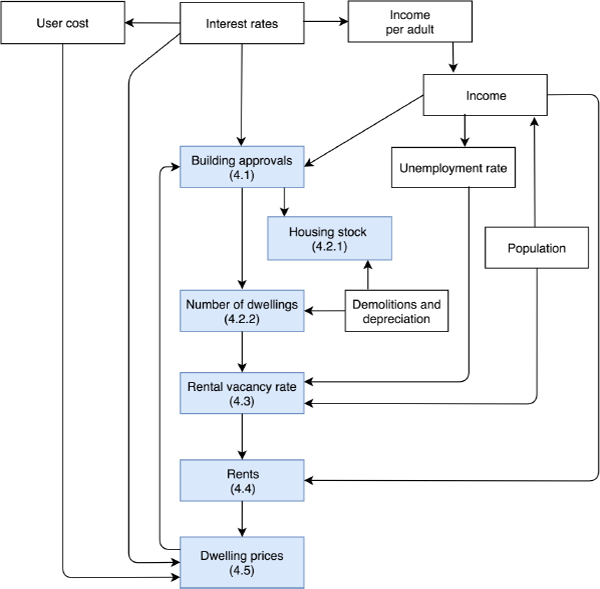
Note: Numbers in parentheses denote the section where the relevant equation is discussed
The model has six important equations, denoted by blue boxes, which we discuss in Section 4. It also contains a large number of identities and simple forecasting equations (typically autoregressions) used for projecting forward variables we think of as exogenous. The equations are presented in Online Appendix E, available in the supplementary information published with this paper on the RBA website.
4. Main Equations
4.1 Building Approvals
We model constant price building approvals for detached houses, higher-density housing, and alterations and additions separately.[1] This disaggregation is useful because the different types of construction behave differently and because alterations and additions do not contribute to the number of new dwellings, an important factor in explaining the vacancy rate. In Section 4.2 we then discuss how these equations are mapped into dwelling investment and completions. Modelling approvals – rather than dwelling investment as many other researchers do – has a couple of advantages. Approvals lead dwelling investment so should improve near-term forecasts. The earlier timing reduces scope for explanatory variables to react, allaying endogeneity concerns. It reduces the lag from shocks to observed decisions. And it facilitates distinctions between the number and value of new dwellings, a feature we exploit in modelling vacancies in Section 4.3.
There are several key features of the data that the model needs to reflect. First, as shown in Figure 2, dwelling investment has grown at a similar rate to real income over the past 60 years, with the ratio between these variables fluctuating around a fairly stable mean. Approvals data show a similar nexus but are only available from 1973. It might seem unsurprising that construction activity grows in line with the rest of the economy, however this is not a feature of some other models, for example Ball, Meen and Nygaard (2010, Table 2).
As we note below, the stability of the ratio in Figure 2 (bottom panel) means that other highly persistent variables, such as real interest rates, real construction costs or real housing prices, do not have large long-run effects on investment, even though some theories suggest they should. Accelerator models suggest that the level of net investment should depend on the growth, as well as the level, of income. However, we do not find the growth in income to have explanatory power. As can be seen in Figure 2 (top panel), the rapid mining-driven growth in income in the 2000s was accompanied by sluggish dwelling investment (from a high starting point). Slow growth in income over the past decade was accompanied by a construction boom.
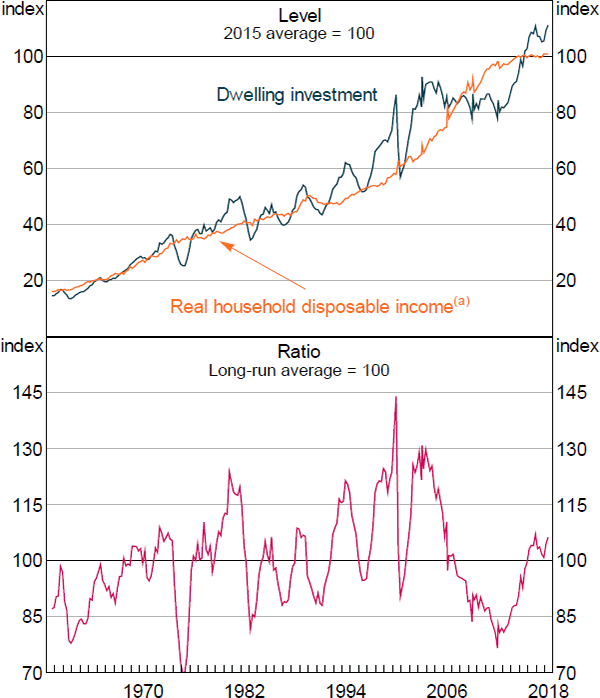
Note: (a) Before interest payments, including unincorporated enterprises; deflated by trimmed mean CPI from 1982 onwards and headline CPI prior to this
Sources: ABS; RBA
Second, in the short run, construction activity seems to be highly responsive to changes in interest rates (Figure 3, top panel) and changes in existing housing prices (bottom panel).
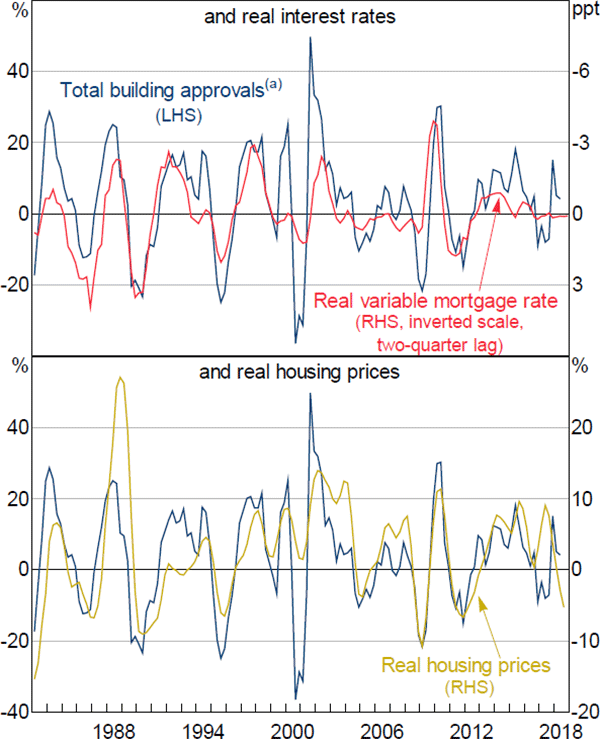
Notes:
All series are year-ended changes
(a) Alterations and additions have been scaled to be representative of their share of national accounts dwelling investment
Sources: ABS; Corelogic; RBA
Reflecting these stylised facts, our three equations for building approvals each take the following form:
where app is a constant price measure of building approvals, hhdy is real household disposable income, R is the real discounted mortgage rate, dp is the real dwelling price, GST is a dummy variable equal to one in the September quarter 2000 and zero otherwise, and ϕ is a time-varying intercept. Lower case variables are in natural logs. All variables in log differences have been demeaned. We estimate from March quarter 1987, following the deregulation of mortgage interest rates, to June quarter 2018. It was common to model dwelling investment before this period as credit constrained (see Jovanoski et al (1997, pp 11–12); Williams (1984)). A summary of estimates is in Table 1. More detailed estimates are provided in tables E1, E2 and E3 of Online Appendix E.
Regressor | Detached houses | Higher-density housing | Alterations and additions |
---|---|---|---|
−0.10** (0.048) |
−0.21 (0.14) |
−0.18* (0.097) |
|
0.19** (0.092) |
−0.60*** (0.19) |
−0.24*** (0.092) |
|
−0.062*** (0.014) |
−0.11*** (0.043) |
−0.037** (0.016) |
|
0.70** (0.33) |
3.0*** (0.95) |
0.77** (0.33) |
|
−0.50** (0.21) |
−0.49 (0.79) |
−0.39 (0.31) |
|
Notes: ***, ** and * denote significantly different from zero at 1, 5 and 10 per cent levels, respectively; standard errors are in parentheses |
We interpret the causation in our equations as running from right-hand side variables to the left-hand side. As noted earlier, we do not prove this, but it strikes us as a natural interpretation. Assumptions of reverse causation – for example, that residuals in the approvals equations cause earlier changes in interest rates – are inconsistent with our understanding of the monetary policy process and the information available to the central bank. Moreover, reverse causation would give rise to a positive correlation, biasing our coefficients towards zero and strengthening our results.
Subject to that qualification, several features of the estimates are worth noting:
- Interest rates have a large and highly significant direct effect on construction activity, as might be expected from Figure 3 (top panel). Figure 4 (top panel) shows responses of approvals to a sustained[3] change in interest rates, holding real housing prices and income constant.
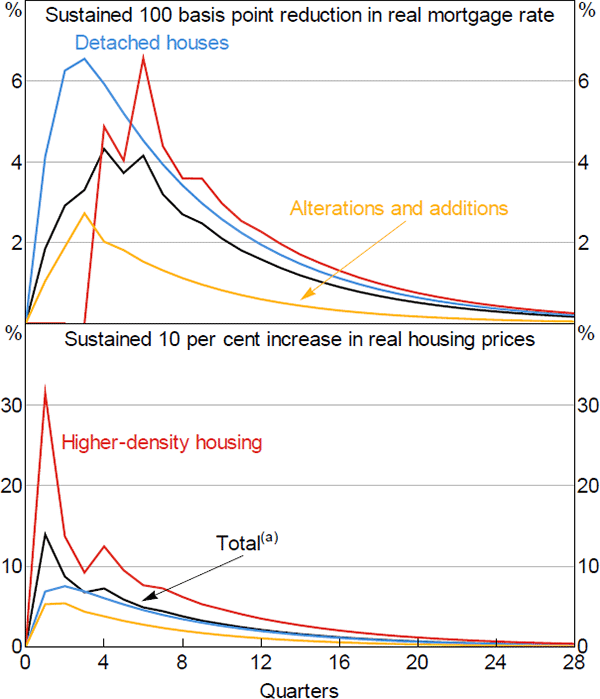
Notes:
Partial equilibrium responses
(a) Weighted by shares in national accounts dwelling investment
- The strong and clear response of residential construction to interest rates is evidence of the potency of monetary policy. It contrasts with the weak and imprecise response of other components of GDP. Researchers have had difficulty in finding an effect of interest rates on consumption, government spending, business fixed investment or inventories (e.g. Caballero 1999). In structural macroeconometric models (e.g. Brayton and Tinsley 1996; Powell and Murphy 1997; Treasury 2001; Fair 2004) effects of interest rates on other expenditure categories are often small or constrained. Consistent with this, structural macroeconometric models find that a large share of the effect of interest rates on GDP reflects dwelling investment[4], and research on the effects of monetary policy can usefully focus on the effects on housing.
- Real housing prices also have a large and highly significant effect on construction activity, as might be expected from Figure 3 (bottom panel). When the price of established housing increases relative to the cost of new housing, the incentive to build increases. Figure 4 (bottom panel) shows the estimated response, holding other explanatory variables constant.
- Although we describe our estimated responses to housing prices as large, estimates from Australian cross-section studies are much larger – often by several multiples. Examples include Gitelman and Otto (2012, Table 3), Liu and Otto (2014), McLaughlin (2011), McLaughlin, Sorensen and Glavac (2016), and Ong et al (2017, Section 3.3). Among other factors, we suspect this reflects differences between our time series estimates, which reflect decisions as to when to build, and cross-section estimates, which reflect decisions as to where to build. There seems to be much more substitution across space than across time.
- As shown in Figure 4 and Table 1, higher-density construction is much more sensitive to housing prices than are other components of dwelling investment. Gitelman and Otto (2012, Table 3) report similar results using census estimates of the housing stock. There are several possible reasons for this.[5]
- It is changes in interest rates and in existing housing prices that drive construction, not their level. So the direct effects of these variables are temporary. Although discussions of dwelling investment often emphasise levels, our attempts to include these in the model did not fit the data. For example, the ratio of approvals to income has been below average over the past decade (Figure 2, bottom panel), even though the level of real interest rates has been unusually low and the relative price of established housing has been unusually high. Similar results and extended discussions are provided by McLaughlin (2011) for Australia and by Mayer and Somerville (2000) for the United States.[6] The latter argue that the superior explanatory power of changes in housing prices and interest rates arises because the level of real housing prices should match the dwelling stock with housing demand. Therefore, a permanent shift in the level of housing prices (or interest rates) would have a finite long-run effect on the level of the dwelling stock, and hence only a temporary effect on the level of net investment. Another interpretation is that these relationships reflect changing political pressures that tighten or loosen land use regulations.
- Other variables that might be thought to directly affect dwelling investment include the rate of economic growth, population growth, construction costs, depreciation, the price of vacant land, rents, long-term interest rates and the user cost of capital. However, most of these were statistically insignificant in our approvals equations. Others had the incorrect sign – perhaps because they proxied for omitted trends (see below). This is not to say that these variables are unimportant – it may just be that they showed little variation over our sample period. In contrast to Ball et al (2010, Equation 10b), Caldera and Johansson (2013, Table 2A) and IMF (2018), we did not find the ratio of established housing prices to the cost of new housing, or Tobin's q, to be significant. The insignificance of the q-ratio may be because the change in existing housing prices captures most of its effect and because the cost of new houses and land is poorly measured.[7]
- While the ratio of dwelling investment to income seems to have been roughly stationary over the past 60 years, it is not precisely constant. Gradual changes in this ratio are likely due to omitted variables, such as the q-ratio, foreign investment, taxes, zoning regulations, chain aggregation and expectations of future demand. These variables are more difficult to quantify than the variables noted in the previous dot point, so it is harder to dismiss their importance. Moreover, shifts in the composition of housing from low to high density will also affect the component-level estimates of the approval-to-income ratios. To account for factors like these, we allow the intercepts in our models to change gradually over time. These can be interpreted as time-varying ratios to disposable income, shown in Figure 5. The use of time-varying intercepts is supported by coefficient stability tests for OLS versions of Equation (1); Quandt-Andrews exp-F tests of stability of the intercept have p-values of 0.6 per cent for detached houses, 0.8 per cent for higher-density housing and 11 per cent for alterations and additions.
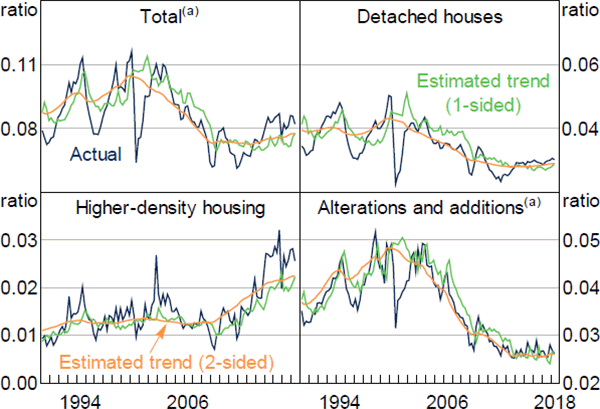
Note: (a) Alterations and additions have been scaled to be representative of their share of dwelling investment
Sources: ABS; Authors' calculations; RBA
4.2 Other Construction Variables
Our model contains a detailed set of equations that trace out the lags in the construction process. Building approvals feed into two separate but related chains of variables, which estimate:
- constant price measures of housing construction, which are predominately used for estimates of dwelling investment and the real value of the housing stock, and
- the number of new dwellings, which feeds into estimates of the rental vacancy rate.
The translation from approvals to the number or value of dwellings is complicated by timing lags, changes in quality, demolitions and other factors. However, these details are mainly of interest to specialists. We briefly mention some key results below and discuss the details in Online Appendix B. The estimated equations are E4–E16 in Online Appendix E.
4.2.1 Dwelling investment and the housing stock (constant prices)
Dwelling investment is a volatile component of GDP(E) and is important for macroeconomic forecasting and policy. It has also been a focus of other researchers and model builders, so our predictions for it facilitate comparisons. Approvals flow into dwelling investment with a long-run elasticity of 1. Specifically, for each category of housing construction, we map approvals through to commencements, then commencements to work done, work done to dwelling investment, and finally, dwelling investment to the constant price measure of the housing stock.
Figure 6 shows our estimated response of dwelling investment to real interest rates. The left-hand panel shows partial responses, holding housing prices, income and other variables constant. These results are directly comparable with the top panel of Figure 4. Comparable results are also shown for two leading Australian macroeconomic models, the AUS-M model of Outlook Economics and the RBA's MARTIN model (with thanks to Adam Hamilton). Over the first year, responses are essentially the same. AUS-M (like its predecessor TRYM) has larger long-term effects because its specification assigns a large persistent role to the level of interest rates, a point discussed above. For an overview of dwelling investment in AUS-M and TRYM, see Jovanoski et al (1997, Section 4.3).
The right-hand panel shows full model responses. In addition to the direct effect (blue), reproduced from the left-hand panel, this includes effects working through housing prices (dark red) and income (grey). The peak full effect is about four times as large as the direct effect, largely reflecting effects working through housing prices. AUS-M and MARTIN also show large effects through housing prices, though the magnitude of differences is sensitive to precise details of the experiment. Coupled with footnote 4, this implies that one of the largest channels through which interest rates affect real GDP is through housing prices. This experiment makes the important assumption that the change in interest rates is expected to be sustained, so flows through fully into long-term interest rates and the user cost of housing. If long-term rates did not change, the estimated contribution from prices would be much smaller, peaking at about 2½ per cent of dwelling investment. The relationship between interest rates and housing prices is discussed in Section 4.5.
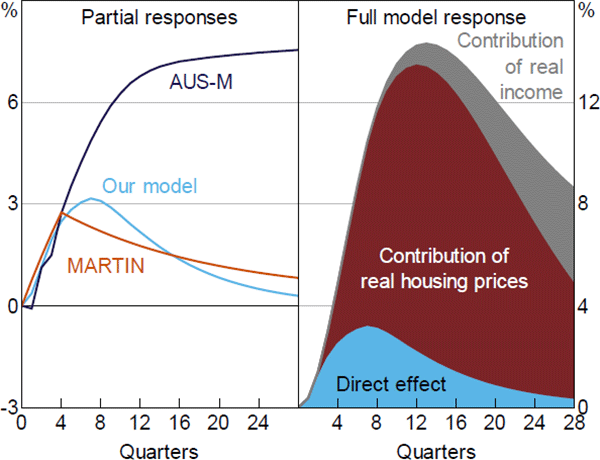
Sources: Authors' calculations; Outlook Economics; RBA
Coupled with (exogenous) depreciation, accumulated investment determines the stock of housing. The lighter line in Figure 7 shows partial responses of the housing stock to a sustained percentage point decrease in real interest rates, holding housing prices, incomes and other variables constant. This increases the housing stock by 0.4 per cent after four years.
As shown by the darker line in Figure 7, a sustained 10 per cent increase in real dwelling prices, holding interest rates, incomes and other variables constant, raises the constant price measure of the housing stock by about 0.7 per cent. This peak elasticity of 0.07 is substantially below estimated price elasticities of 0.4 for Sydney property by Gitelman and Otto (2012, Table 3) or 0.3 for regional NSW property by Liu and Otto (2014). As noted earlier, we suspect much of this difference represents a difference between time-series and cross-section studies. The contrast is even larger for studies such as Ball et al (2010) and Caldera and Johansson (2013) that find the level of prices permanently affects investment, which would make the long-run effect on the housing stock infinite (in the absence of depreciation).
It is often suggested that increased subsidies or tax concessions for housing would have little effect on supply, which is said to be constrained by limited land and planning permissions. That view seems to be inconsistent with the responsiveness to relative prices that we and others estimate. Although our estimates are smaller than others, they are still significant. Legal and physical restrictions on land development are important, but they appear to be either responsive to financial considerations or not fully binding.
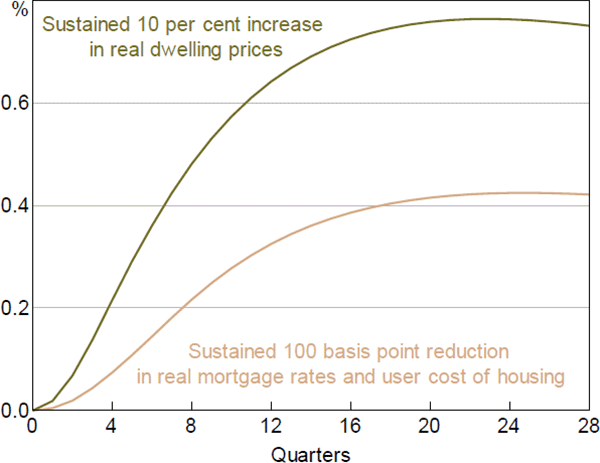
The housing stock plays an important role in some models of the housing market. For example, feedback from the stock can help explain ‘cycles’ or oscillations in construction (Jovanoski et al 1997, Section 2.1). However, we were unable to find a satisfactory role for the housing stock in our equations. The housing stock is highly correlated with income and fits the approvals data slightly better. However, our judgement was that the high correlation between construction and income is an important feature of the data and it was difficult for a model with a large role for the housing stock to capture this. As discussed below, the number of dwellings seems to matter for balance in the rental market. But the size and quality of those dwellings does not. As we show in Section 5, our model does not exhibit important ‘cobweb’ or oscillatory responses. Berger-Thomson and Ellis (2004) find that the cyclicality in dwelling investment reflects cyclicality in interest rates.
4.2.2 Completions and the number of dwellings
The second chain of variables affected by dwelling approvals explains the number (as distinct from the value or volume) of dwellings that are built. As discussed in more detail in Online Appendix B, to estimate the number of approvals we remove alterations and additions and the effect of the changes in quality (most evident in larger floor sizes) from the estimates of new dwelling approvals discussed in Section 4.1. We then map the number of new approvals through to the number of commencements, then commencements to completions, and completions to the number of dwellings, which feeds into estimates of the rental vacancy rate. For both detached houses and higher-density housing, approvals flow through to completions with an elasticity of 1, although with different lags. We estimate that 83 per cent of detached houses are completed one year after approval, and 95 per cent in the long run. For higher-density housing, 61 per cent of approvals are completed within a year and 85 per cent in the long run.
In simulations below, the number of dwellings typically reacts substantially less to shocks than does the constant price measure of the housing stock. As this implies, the average quality of dwellings plays a large part in responses. That partly reflects changes in alterations and additions, which affect the constant price stock, but not the number of dwellings. It is also because dwellings built now are of a higher quality than those in the current stock. So new construction increases the average quality.
As discussed in Online Appendix B, we assume that the average quality of new dwellings is proportional to income per capita. This implies that, while constant price approvals and residential investment are proportionate to total income in the medium run (Section 4.1), the number of approvals and completions is proportionate to population. Hence, in steady state, they grow at the same rate.
4.3 Rental Vacancies
Figure 8 shows components of what might be considered as ‘excess supply’ of housing. The green line in the top panel shows quarterly changes in the number of dwellings. This equals housing completions (as modelled in the previous section) less demolitions.[8] This is often called the supply of new dwellings, though the demand/supply distinction is difficult to apply to owner-occupied housing, which households provide to themselves. These estimates are lower than the ‘housing supply’ estimates of Ong et al (2017, Section 2) because we allow for both approvals that are not completed and demolitions. The purple line shows what we call ‘underlying’ household formation, defined as quarterly changes in adult population, which is an important and exogenous component of underlying demand, divided by a five-year trailing average of adults per dwelling.[9]
We refer to the gap between these series as ‘excess completions’ measured as
where K is the total number of dwellings, WAP is working-age population (15+), and is the five-year trailing average of adults per dwelling. Excess completions are plotted as the brown line in the bottom panel of Figure 8, as a share of the total number of dwellings. This resembles, though is somewhat simpler than, the ‘housing gap’ measure examined by Kohler and van der Merwe (2015) or the ‘State of Supply Reports’ of the National Housing Supply Council (e.g. NHSC 2014).
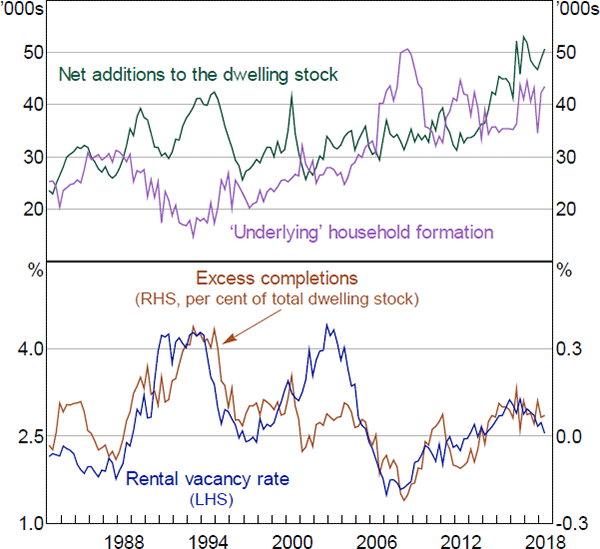
Sources: ABS; Authors' calculations; Real Estate Institute of Australia (REIA)
The blue line in the bottom panel shows the rental vacancy rate, measured as the number of properties listed as available for rent, divided by the total number of rental properties. As shown in the figure, excess completions and the vacancy rate move together closely. This is perhaps not surprising given that both series can be interpreted as measures of excess supply in the housing market. Despite these considerations, researchers have not been able to successfully model this relationship. See, for example, the discussion in Jovanoski et al (1997, p 9). That is partly because the vacancy rate is a stock whereas excess completions are a flow and partly because the high-frequency correlation between the series is very weak.
Observation of local housing markets suggests that the relationship between excess completions and the vacancy rate is causal. When extra households are formed, for example due to higher immigration, the market tightens and vacancies fall. When extra stock comes onto the market following a building boom, vacancies rise. Accordingly, we assume that excess completions drive the vacancy rate. However, we assume that they do so gradually, so that the correlation evident in Figure 8 holds in the long run, but not instantaneously. Moreover, we add the change in the unemployment rate and reversion to the mean, both discussed below. Specifically:
where V is the rental vacancy rate, EC is excess completions, K is the total number of dwellings, UR is the unemployment rate and White t-statistics are in brackets. Detailed estimates are at equation E17 in Online Appendix E.
The change in unemployment represents the effect of the business cycle: extra employment and income increase demand for housing and reduce desired household size. Ideally, these effects – like reversion to the mean – would be captured by changes in household size and hence as an additional determinant of household formation. In practice we are unable to distinguish these changes in household size from those that endogenously accompany and neutralise building completions. As an expedient, we fix the number of adults per dwelling at its previous five-year average and allow factors that affect household size to enter Equation (2) directly. Although this complicates the interpretation, these variables have high explanatory power, as judged by the t-statistics in Equation (2).
The estimated steady-state vacancy rate – at which the vacancy rate has no tendency to change – is a function of excess completions. When excess completions are zero, so the number of dwellings is increasing as quickly as underlying household formation, the steady-state vacancy rate would be 2.4 per cent. This benchmark corresponds to some interpretations of ‘balance’ in the housing market and we use it in our equation for rents. However, other benchmarks are also interesting. To stabilise vacancies at their historic mean, 2.8 per cent, would require excess completions of 0.06 per cent of the housing stock per quarter. A vacancy rate of 3 per cent would keep rents growing at the same rate as other consumer prices; this would require excess completions of 0.09 per cent of the housing stock per quarter.
The short-run contribution of excess completions is not estimated but constrained to a value that seems plausible and provides reasonable system properties. Specifically, new completions increase the number of rental vacancies in proportion to the share of rental accommodation in the housing stock, which was 29 per cent at the time of the 2011 ABS Census. That is, if the total housing stock increased by 100 dwellings, the number of vacant rental properties would initially increase by 29 dwellings. Although the short-term fit of this equation is not good, the model does explain longer-term variations in vacancies, as judged by a dynamic simulation starting from 1983:Q1 (Figure 9) and model simulations discussed in Section 5. Moreover, combined with other equations, the overall system has similar properties to a reduced-form regression in which growth of real rents is directly explained by excess completions (not shown) or studies of the effect of housing supply on prices (Section 5.3).
A limitation of our model is that we assume that household formation is largely a function of exogenous population. This means that imbalances in the housing market are corrected by adjustments to supply, not demand. The assumption that population growth is exogenous seems reasonable (overseas arrivals are cyclical, but feedback from the housing sector is small). However, household size surely responds to price signals. In preliminary work, we estimated that rising real rents have increased the number of adults per dwelling since the early 1980s, but this effect has been more than offset by higher real incomes. However, this result was highly sensitive to the chosen model specification and sample period. This is an issue for future research.
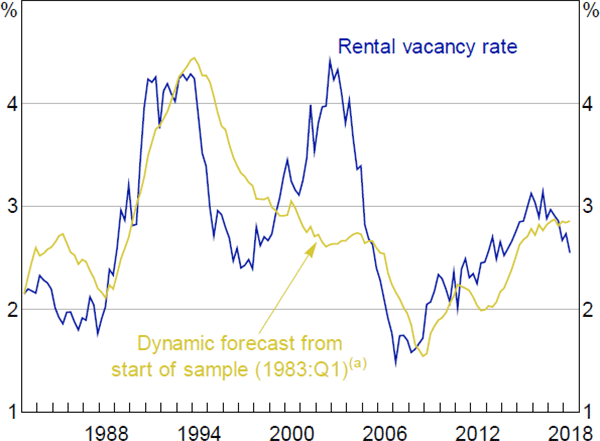
Note: (a) The forecasts use actual data for the explanatory variables
Sources: Authors' calculations; REIA
4.4 Rents
Our equation explaining the growth of real rents is as follows:
where rent is real CPI rents, V is the rental vacancy rate (entered as the deviation from its benchmark value of 2.4), and Δinc is the growth in real household disposable income (measured as the deviation from its sample mean). Rents and income are in logs and deflated by annualised trimmed mean CPI inflation over the previous three years. βi, αi and ϕi are lag weights, each of which sums to 1. White t-statistics are in brackets. Detailed estimates are at equation 18 in Online Appendix E.
The dominant influence on real rents is the vacancy rate, as shown in Figure 10. This effect is analogous to the Phillips curve in the labour market. However, whereas the Phillips curve seems increasingly difficult to see (Gillitzer and Simon 2015), the effect of an excess supply of housing on changes in rents is clear, with the coefficients on the vacancy rate in Equation (3) being highly significant.[10] It is sometimes questioned whether the balance of supply and demand affects the cost of housing (Phibbs et al 2017; Coates 2018), but the evidence seems to be strong.
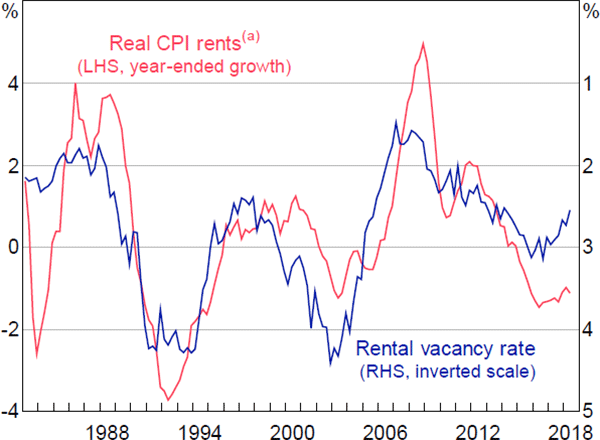
Note: (a) Deflated by annualised growth of trimmed mean CPI over the previous three years
Sources: ABS; Authors' calculations; RBA; REIA
When the vacancy rate is 2.4 per cent, the rate consistent with the housing stock increasing as fast as population, real rents are estimated to grow at 0.9 per cent a year. The vacancy rate will deviate from this benchmark due to changes in population growth, underlying household size, zoning regulations and so on. Any projection of rents, housing prices or other features of the housing market requires an assessment as to how these factors will evolve, which our model does not explore.
Rents exhibit a high level of momentum, with coefficients on the lagged changes in real rents summing to 0.7 (standard error = 0.06). This inertia, coupled with the high explanatory power of the equation (R 2 = 0.80), makes rents unusually easy to forecast, relative to other components of the CPI.
Equation (3) resembles that of Jovanoski et al (1997, Section 4.1). The main difference is that we smooth non-rental prices by averaging over time rather than by controlling for import prices. Neither the real nor the nominal share of rent in consumption or income, nor the relative price of rent have been stationary. So we do not impose these as long-run conditions.
4.5 Housing Prices
Research on the determinants of housing prices, both in Australia (Otto 2007; Abelson 2018) and overseas (Duca, Muellbauer and Murphy 2011; Glaeser and Nathanson 2014; Oxford Economics 2016), commonly focuses on the user cost of housing. The user cost represents the annual cost of owning a house, comprising the sum of interest payments, repairs, rates and other running costs, less expected capital appreciation. For recent Australian estimates and discussion, see Fox and Tulip (2014).[11] In the long run, this tends to be close to the cost of renting a similar dwelling. If the user cost were lower than rents, households would be financially better off owning than renting and might be expected to bid up the price of houses. Conversely, when renting is cheaper, there would be downward pressure on housing prices. This implies the arbitrage condition:
where USERCOST is the user cost of housing expressed as a share of the dwelling value. In our estimated equation, housing prices gradually adjust to this long-run target so as to keep the cost of owning close to the cost of renting. In the short run, prices exhibit strong momentum and respond to short-term variable rates. Specifically:
where dp is real dwelling prices, rent is real CPI rents, usercost is the user cost of housing, and R is the real discounted variable mortgage rate. Lower case variables are in natural logs (so rent – dp is the log of the rental yield). Housing prices and the mortgage rate are deflated by annualised trimmed mean CPI inflation over the previous three years. White t-statistics are in brackets. Detailed estimates are at equation E19 in Online Appendix E. Otto (2007) finds that similar specifications explain city-level changes in house prices in Australia.
The gradual adjustment of the rental yield towards the user cost and the momentum in short-term price movements make housing prices quite predictable. Our equation has an R 2 of 0.69, with no contemporaneous regressors. The predictability of changes in housing prices is well established (Case and Shiller 1989; Glaeser and Nathanson 2014). It is also inconsistent with models of efficient asset pricing, in which predictable movements are arbitraged away. One possible interpretation of the large coefficient (0.74, t = 11) on the lagged change in prices is that it reflects extrapolative expectations, which could make the market prone to bubbles. Another interpretation is that it reflects gradual adjustment to omitted variables.
However, any deviations from efficiency appear to be transitory. In the long run, prices move towards their efficient level given by Equation (4). One implication is that a change in rents is fully reflected in a change in housing prices, though this takes many years to flow through.
Our estimates of the user cost assume that expectations of capital gains are exogenous and approximately constant, equal to the historical mean. These expectations are simple, naïve and involve an inconsistency: market participants will bid up housing prices on the implicit assumption that other buyers will behave differently. More complicated assumptions are easy to imagine but fit the data less well.[12] As discussed in Section 5.4, our assumption of mean expectations is quite different to model-consistent expectations. However, it is unclear how expectations should be modelled. In the long run, it would be desirable if expectations were consistent with the model, though the literature on housing bubbles suggests that model-consistent expectations are an unrealistic assumption in the short run.
User cost models like ours differ from reduced-form models in which housing prices are directly determined by fundamentals such as housing construction or population.[13] As shown in Section 5, variables like these have large effects on housing prices in our model, but they do so indirectly by affecting the balance of demand and supply of housing as measured by vacancies and changes in rents. One advantage of our approach is that it directly maps into the decisions of home buyers, who will take rents and the user cost as given. Reflecting this, user cost models provide a relevant measure of ‘overvaluation’ that can be used in household financial decisions and in detecting bubbles, as argued by Fox and Tulip (2014).[14] Another advantage is that our approach is more comprehensive. If some factor were to boost the demand for housing, but this effect was offset by higher supply, so that vacancies and rents do not change, there should be no effect on house prices. Controlling directly for rents allows all factors determining demand and supply – including those the econometrician does not observe – to enter. Weaknesses of our approach would be if our estimates of rents and the user cost were mismeasured or if fundamental factors affected future but not current rents. Empirically, those problems do not seem to be important. When added to Equation (5), these other variables are not statistically significant over our sample period.
The effect of interest rates on housing prices plays an important role in several of this paper's conclusions and is somewhat complicated. So the effect and the evidence of its strength are worth explaining.
At a user cost of 6 per cent we estimate that a sustained percentage point drop in interest rates would, in the long run, boost housing prices by 17 per cent, holding rents and other components of the user cost equal. This semi-elasticity (the proportionate increase in housing prices for a given percentage point increase in interest rates) is larger than the time series estimates of Abelson et al (2005), perhaps because they control for many other variables affected by interest rates (e.g. share prices and income), so only capture part of the effect of interest rates. Our estimate is similar to Gitelman and Otto's (2012, p 183) estimate of 20 per cent for Sydney housing prices using disaggregated census data, and Himmelberg, Mayer and Sinai's (2005, p 78) estimated range of 19 to 33 per cent for the United States in a calibrated model.
A user cost (or rental yield) of 6 per cent is the approximate average over Gitelman and Otto's sample. User cost models, such as Equation (4), imply that the semi-elasticity increases as the user cost declines.[15] Effects on prices become extremely large as the denominator in Equation (4) approaches zero. With a current user cost of 3½ per cent (well below that observed in the studies above), a percentage point drop in the expected real mortgage rate would boost housing prices by 28 per cent in the long run.
These large effects reflect cointegration between the rental yield and the user cost (Equation (4)). Our estimates of Equation (5) (E19 in Online Appendix E) and textbook models of asset pricing both support this assumption, but we would not describe this evidence as strong. While the coefficient on the error correction term is statistically significant (t-statistic of 2.6), it is small (0.023) and sensitive to alternative specifications.
Stronger evidence in favour of Equation (4) is its ability to explain the level of prices. According to CoreLogic, the average rental listing for houses across seven capital cities in August 2017 was $24,000 a year.[16] Divided by the September quarter 2017 user cost of 3.6 per cent, Equation (4) implies home buyers would be as financially well off paying $667,000 for a similar dwelling. This is close to CoreLogic's estimated valuation of those same rental properties of $735,000.
This comparison can be repeated at earlier dates, as shown by Figure 11, which shows the user cost of housing and two estimates of the rental yield. The brown line uses CoreLogic data on matched samples, where rents and imputed prices are measured for the same properties. As Fox and Tulip (2014) argue, this is a relevant measure for explaining housing prices and their ‘fair value’. The CoreLogic series is available from 2005 to September quarter 2017. Before 2005 we splice with REIA estimates of median rents and prices, which are not matched but use consistent definitions. Both the level and low-frequency changes in this measure of the rental yield match the user cost – especially in the latter part of the sample, when data quality is higher. Close long-term relationships between alternative measures of the user cost and the rental yield are also shown for Australia by Otto and Stapledon (2017, Figure 2) and for the United States by Duca et al (2011, Figure 2).
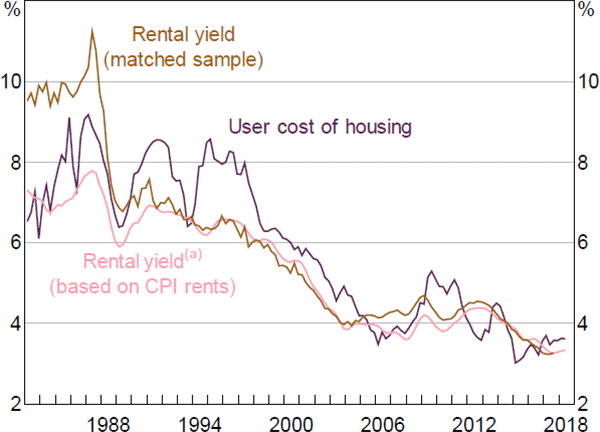
Note: (a) Scaled to have same mean as the matched sample rental yield from 1988 to 2018
Sources: ABS; Authors' calculations; Corelogic; REIA
Our model uses more widely known and accessible series for rents and prices: the rent component of the consumer price index and the CoreLogic hedonic price index. The ratio of these series is shown as the pink line in Figure 11. In contrast to the matched sample, these series are in different units, so they do not explain the level of housing prices, however, they still explain longer-run variations. We have adjusted the mean of their ratio to equal the matched sample rental yield from 1988 to 2018. In principle, different quality adjustments in the numerator and denominator of this measure of the rental yield make its longer-term movements difficult to interpret. In practice, the correspondence of this series to the matched sample estimate suggest this inconsistency has not been important.
It is the path of interest rates expected over the ownership of the asset that determines the full cost of purchase. So long-term interest rates enter the user cost. However, housing prices seem to also respond to variable mortgage rates in the short run, possibly because of cash flow constraints. To account for this, we have included changes in the real variable mortgage rate in Equation (5).
Short-run responses will depend on how long a change in interest rates is expected to be sustained and on how quickly this is reflected in long-term interest rates. Modelling expectations is outside the scope of this paper. However, in Section 5.1 we consider a percentage point cut in interest rates that is expected to be sustained for three years, which may be representative. In this scenario, dwelling prices increase by 8 per cent after two years. This estimate is near the middle of the range of estimates surveyed by Williams (2016, Table 1).
To summarise, although our estimated responses of housing prices to interest rates may seem surprisingly large, they are in line with other research (both in Australia and overseas), and are consistent with theory and the available data.
Since 1982, real dwelling prices have grown at an average annual rate of around 3 per cent, approximately tripling in value (depending on the measure). About 18 percentage points of this increase is attributable to the increase in real rents over this period. The substantial majority of the change represents a decline in the rental yield, which as Figure 11 indicates is essentially explained by the fall in the user cost. Most of this fall reflects a decline in expected real mortgage rates from a peak of 6 per cent in the 1980s to 3.2 per cent recently. Holding rents and other components of the user cost constant, this would account for a 45 per cent increase in housing prices. Other components of the user cost, in particular running costs, also contribute. However, reflecting the nonlinearity discussed above, the interaction of these components is more important than any other single contribution. Moreover, reflecting volatility of bond yields in the early 1980s, any quantitative decomposition is quite sensitive to the assumed starting point.
This explanation of the trend increase in housing prices differs from other popular accounts. It is straightforward to reconcile some of these:
- Other factors that raise demand for housing, such as immigration or income growth, boost prices by raising rents, which the implicit counterfactual above takes as given. When this assumption is relaxed – as in Section 5.2, for example – these demand-side factors have large effects on prices.
- Kendall and Tulip (2018) attribute higher prices to zoning regulations. In terms of a conventional supply-demand diagram, zoning restrictions make the supply curve steep, while lower interest rates (or other factors) shift the demand curve out. It is the interaction of rising demand with inelastic supply that explains higher prices.
- The liberalisation of credit markets, emphasised by Yates (2011, Section 2.2) among others, led to a narrowing in lending margins. This is a significant factor in the large decline in real mortgage interest rates over this period.
- According to the Taylor principle, nominal interest rates should respond more than one-for-one to deviations of inflation from target. Hence the change in inflation from above target at the beginning of our sample to below target recently accounts for some of the downtrend in real interest rates.
In contrast, our model would need to be modified to allow for some other suggested explanations of the boom in house prices.
- Anecdotal observation, the important role of variable rates and previous RBA work (see the references in RBA (2014, p 6)) suggest, without quantifying, that changes in borrowing limits affect housing prices in the short run. For example, tightening in lending limits seems to be important in the decline in house prices in 2018. Some international research (e.g. Duca et al 2011) finds a larger role. However, Australian empirical research, such as Otto (2007) or our investigations, has not found borrowing limits to have longer-run effects on house prices. Borrowing constraints affect the demand for housing assets not the demand for housing services. As Kaplan, Mitman and Violante (2017) discuss, this would be expected to affect the ownership rate, not the level of house prices.[17]
- Our measure of the user cost is relevant to owner-occupiers. A more complicated measure for investors would be needed to assess the effect of tax changes. This is a task for future research. Daley, Wood and Parsonage (2016, Box 6) estimate that the concessional treatment of capital gains tax and negative gearing may boost the level of housing prices by about 2 per cent.
4.6 Other Equations
The full model contains 62 equations, presented in Online Appendix E. Most of these are identities or autoregressions. However, three further equations might be worth mentioning. Our equation for real income is calibrated so that it reflects the response of GDP to the real cash rate in the MARTIN model.[18] The unemployment rate reacts to income in line with Okun's rule. Long-term interest rates (which feed into the user cost) equal the average of future variable rates.[19]
5. Model Responses
We now bring the equations together. Specifically, this section presents full-model responses to interest rates, population, approvals and housing prices. Our model is nonlinear, so responses will vary somewhat in different conditions.[20] When we show deviations from baseline, it is from the model projection described in Online Appendix C.
5.1 Responses to Interest Rates
Figure 12 shows deviations from baseline arising from a 1 percentage point reduction in real variable interest rates. Aqua lines show a change that lasts – and is expected to last – for three years, which resembles some changes in monetary policy. Maroon lines show a change that is expected to last indefinitely, which is more relevant for longer-run effects and explaining some historical developments.
The two scenarios differ not only in the duration of effects but also in their strength. A cash rate change that is expected to be long-lasting feeds one-for-one into long-term interest rates and the user cost of housing (top right panel), with the temporary change having much less effect. Changes in the user cost give rise to similar, but lagged, changes in the rental yield (2nd row, left) which involves substantial increases in housing prices (2nd row, right).
The combination of lower interest rates, higher housing prices and higher income boost dwelling investment (3rd row, left, the maroon line is the total in the right-hand panel of Figure 6). That increases the dwelling stock (3rd row, right and 4th row, left) and rental vacancy rate (4th row, right). Rents (bottom left) initially rise due to the income boost from lower interest rates, but as extra supply builds, they begin to fall.[21] The signs of the effects on vacancies and rents both vary with time and with the expected duration of the shock.
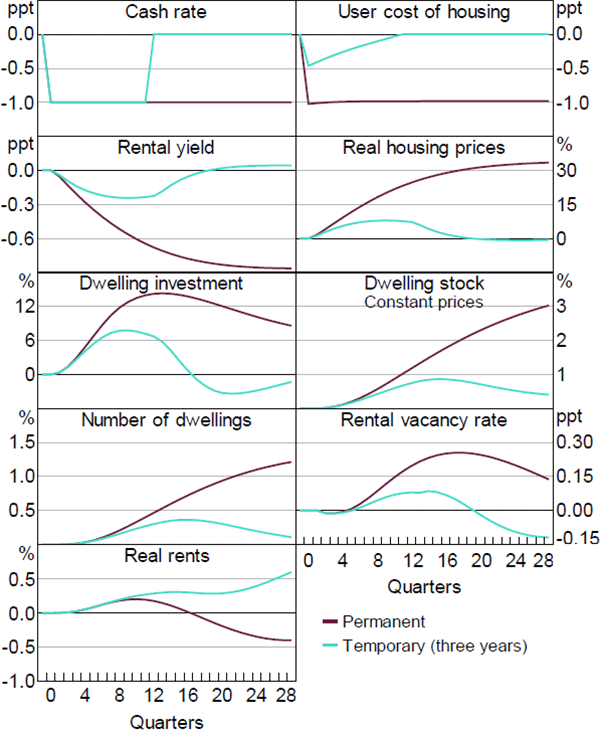
For a slightly different perspective, Figure 13 shows estimated effects of the recent decline in interest rates. Since its peak in 2011, the cash rate has fallen from 4¾ per cent to 1½ per cent, while the user cost has fallen from almost 5 per cent to around 3½ per cent. The decline in the user cost reflects a fall in long-term real interest rates, which in turn reflects falls in global rates and expectations that the decline in short rates will be persistent. Actual outcomes are in maroon and a counterfactual, in which interest rates remain at their June quarter 2011 levels, in aqua. The model estimates that the reduction in real interest rates accounts for most of the subsequent boom in dwelling prices (middle left panel) and a large part of the boom in dwelling investment (middle right).[22] The increase in housing supply boosts the vacancy rate and reduces rents. However, these effects are offset by the effect of higher income, with neither the vacancy rate (bottom left) nor rents (bottom right) being much changed on net.
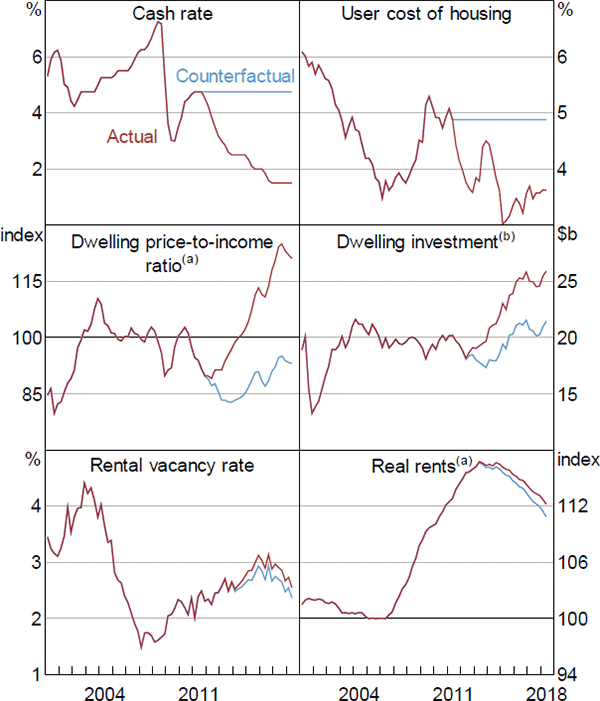
Notes:
(a) 2005 average = 100
(b) Chain volume
5.2 Responses to Population Growth
Figure 14 shows estimated effects of the population boom of the mid-to-late 2000s, with actual outcomes shown in red. Reflecting a surge in immigration, year-ended growth in the adult population (15 years and older) rose from 1.5 per cent in 2005 to 2.4 per cent in 2008.[23] To assess the effects of this surge, we run a counterfactual simulation, shown in blue, in which adult population growth continues to grow at its 2005 rate of 1.5 per cent.
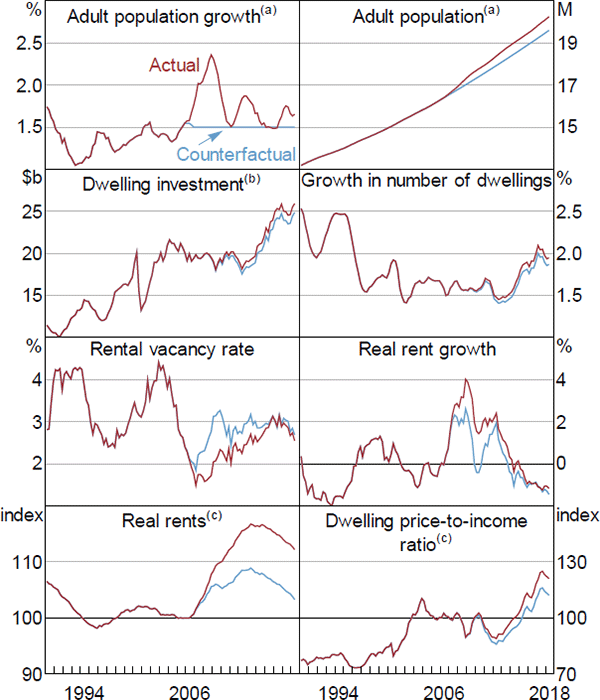
Notes:
Growth rates are year-ended
(a) 15+ years
(b) Chain volume
(c) 2005 average = 100
The population surge is shown in changes (top left) and levels (top right). By 2018, it boosts the adult population by 3.3 per cent. With per capita income unchanged by assumption, total income (not shown), and hence dwelling investment (2nd row, left) also increase by about 3.3 per cent. This raises housing supply slightly (2nd row). However, the short-run boost to housing demand is much larger, leading to a fall in the rental vacancy rate to a near-record low of 1½ per cent in 2008 (3rd row, left). Rents, which were already growing quickly, accelerate to grow 4 percentage points faster than the overall rate of inflation. Without the extra population, our simulation suggests that real rents would have only grown by 2 per cent a year, as shown by the 3rd row, right panel. The cumulative result was that real rents were 9 per cent higher in 2018 than they would have been otherwise (bottom left). The increase in rents gradually flows on to a similar increase in dwelling prices (bottom right), although this effect is small relative to the effect of interest rates, discussed in the previous section.
Our estimate of the effect of population on real rents and housing prices is similar to that estimated for Spain by Gonzalez and Ortega (2013). It is larger than that estimated for the United States by Saiz (2007), presumably because land use regulations make housing supply more elastic in much of the United States. It is substantially larger than many studies find for local effects on house prices as surveyed by Larkin et al (2018). The effects of immigration on relative neighbourhood prices tend to be more negative than effects on aggregate prices, so estimates of the effect increase with the level of geographic aggregation.
5.3 Responses to Completions
Figure 15 shows the effects of an exogenous increase in construction as a deviation from baseline. We assume that each component of building approvals increases by 10 per cent for one year (top left panel), which represents about 21,400 extra approvals for new dwellings. This would boost the total number of dwellings by about 18,200 (allowing for approvals not completed and demolitions) or 0.16 per cent (middle left). The extra ‘supply’ (as it is commonly termed) would increase the vacancy rate (middle right), and hence lower rents (bottom left) and housing prices (bottom right). The proportionate response of rents and prices (0.4 per cent) is 2.5 times as large as the increase in the number of dwellings (0.16 per cent). This ratio (2.5) represents the inverse of the elasticity of housing demand. It also applies to larger and more sustained shocks. As a rule of thumb, every 1 per cent increase in the number of dwellings (when driven by an increase in supply) lowers the cost of housing by 2½ per cent.
Our estimate of the elasticity of housing demand lies well within the range of other estimates. Abelson et al (2005) estimate that a 1 per cent increase in the Australian housing stock per capita leads to an estimated decrease in real housing prices of 3.6 per cent in the long run. Girouard et al (2006) summarise ten international studies, which have an average estimate of 3.1. Two recent and arguably more thorough studies point to smaller effects. Albouy, Ehrlich and Liu (2016, Table 3) find that a 1 per cent increase in real housing expenditure in the United States is associated with less than a 2 percentage point reduction in price. Oxford Economics (2016) find that a 1 per cent increase in the number of houses in the United Kingdom would reduce house prices by 1.8 per cent and cite (their Figure 20) several other elasticities that range between 1.1 and 2.2.[24]
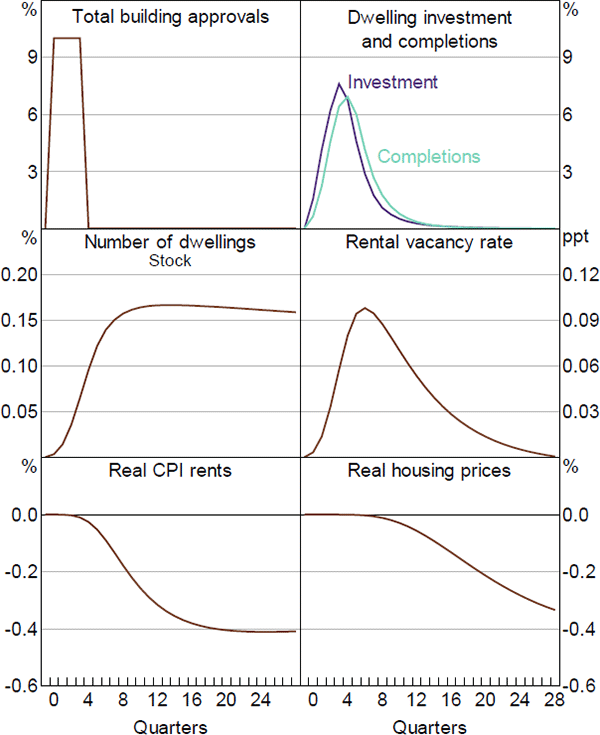
5.4 Responses to Changed Price Expectations and the User Cost
Our measure of the user cost assumes that home buyers expect real constant-quality housing prices to continue rising at their post-1955 average rate of 2½ per cent a year. This is a simple assumption that is consistent with some of the main features of the data. However, forecasts from our model, such as the projection discussed in Online Appendix C, imply that real housing prices (measured with a different quality adjustment) will grow at an annual average rate of 0.2 per cent over the next ten years. Figure 16 shows the effect of a change in expectations about capital appreciation from the former assumption to the latter. This scenario can also be interpreted as capturing the effect of an exogenous fall in housing prices. Were the model to be extended to include taxes on housing, this is an important channel through which they would operate.
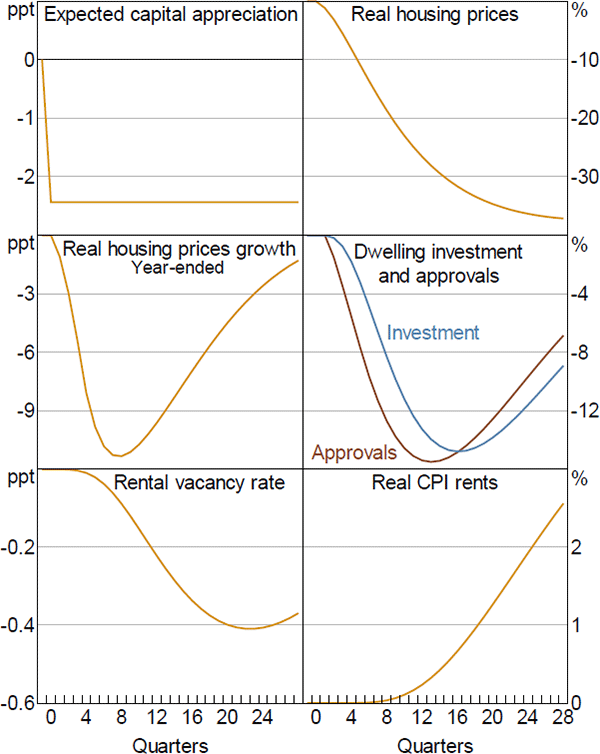
As shown in the top left panel, expected capital appreciation declines 2½ percentage points. The user cost (not shown) rises by the same amount. Housing prices (top right and middle left) then take a long time to adjust, falling gradually, but substantially, to be one-third lower after five years. As Krugman (2005) argues, housing bubbles do not ‘burst’, they gradually deflate. This scenario is extremely unlikely: nothing like it has happened in Australia before. However, in scale and duration, it resembles the largest housing collapses seen during the global financial crisis (Ireland, Spain, United States), so is relevant as a worst-case scenario to be guarded against. Falling house prices result in large falls in investment (middle right), which reduce vacancies (bottom left), boosting rents (bottom right). The decline in construction dampens the fall in prices.
The limitations of this partial equilibrium exercise should be emphasised. In a more complicated model, falling house prices would reduce household wealth and hence consumption. The net worth of financial intermediaries would fall. Offsetting this, interest rates would fall, moderating the macroeconomic impact. That said, the housing market response would be an important part of the overall effect.
6. Conclusion
Our main results, which we do not repeat, were stated in the introduction. Several limitations of our model and directions for further research are worth noting.
Some of our equations are better than others. The stability and precision of our estimates give us confidence in the responsiveness of construction to interest rates and prices and in the response of rents to vacancies. We are much less certain about the response of vacancies to completions and household formation. More broadly, we know more about the response of quantities to prices than we do about the response of prices to quantities.
To keep our analysis relatively transparent and manageable, we have ignored some minor variables (like taxes, lending restrictions, the approvals pipeline and first home owner grants). And we have assumed that many relevant variables are exogenous. A more complicated analysis would relax those assumptions. A priority is to allow household size to depend on income and rents. This would then affect household formation and housing quality. It is not clear that adding taxes to the user cost would substantially change the behaviour of the model, but it would be useful for policy discussions.
We have also assumed that most behaviour is backward looking (though house prices depend on expectations of interest rates and capital gains). That assumption seems consistent with the stability of our equations. The conditions in which it would hold in the future would be interesting to explore.
Although our equations represent a long search over alternative specifications, this was far from exhaustive. Further examination of aggregate time series would doubtless improve on our model. However, we suspect that a more fruitful line of enquiry might involve examining alternative approaches and datasets. Cross-section, panel and event studies provide information that should corroborate or modify our estimates, particularly of long-run parameters.
References
Abelson P (2018), ‘House Prices, Rents, Home Ownership and Affordability: The Facts and a Mainstream Economics Explanation’, Presentation given at Henry Halloran Trust, University of Sydney, Sydney, 31 May.
Abelson P, R Joyeux, G Milunovich and D Chung (2005), ‘Explaining House Prices in Australia: 1970–2003’, The Economic Record, 81(s1), pp S96–S103.
ABS (Australian Bureau of Statistics) (2007), Information Paper: Statistical Implications of Improved Methods for Estimating Net Overseas Migration, Australia, 2007, ABS Cat No 3107.0.55.005, Australian Bureau of Statistics, Canberra.
Albouy D, G Ehrlich and Y Liu (2016), ‘Housing Demand, Cost-of-Living Inequality, and the Affordability Crisis’, NBER Working Paper No 22816.
Ball M, G Meen and C Nygaard (2010), ‘Housing Supply Price Elasticities Revisited: Evidence from International, National, Local and Company Data’, Journal of Housing Economics, 19(4), pp 255–268.
Berger-Thomson L and L Ellis (2004), ‘Housing Construction Cycles and Interest Rates’, RBA Research Discussion Paper No 2004-08.
Bourassa SC and PH Hendershott (1995), ‘Australian Capital City Real House Prices, 1979–1993’, The Australian Economic Review, 28(3), pp 16–26.
Brayton F and P Tinsley (eds) (1996), ‘A Guide to FRB/US: A Macroeconomic Model of the United States’, Board of Governors of the Federal Reserve System Finance and Economics Discussion Series No 1996-42.
Caballero RJ (1999), ‘Aggregate Investment’, in JB Taylor and M Woodford (eds), Handbook of Macroeconomics: Volume 1B, Handbooks in Economics 15, Elsevier Science, Amsterdam, pp 813–862.
Caldera A and Å Johansson (2013), ‘The Price Responsiveness of Housing Supply in OECD Countries’, Journal of Housing Economics, 22(3), pp 231–249.
Case KE and RJ Shiller (1989), ‘The Efficiency of the Market for Single-Family Homes’, The American Economic Review, 79(1), pp 125–137.
Coates B (2018), ‘Overview of Poll Results’, Poll conducted by The ESA Monash Forum, viewed 22 January 2019. Available at <https://www.monash.edu/business/economics-forum/polls/will-building-more-homes-make-housing-cheaper/overview-of-poll-results-by-brendan-coates>.
Cusbert T and E Kendall (2018), ‘Meet MARTIN, the RBA's New Macroeconomic Model’, RBA Bulletin, March, viewed 22 January 2019.
Daley J, D Wood and H Parsonage (2016), Hot Property: Negative Gearing and Capital Gains Tax Reform, Grattan Institute Report No 2016-8, Grattan Institute, Melbourne.
Duca JV, J Muellbauer and A Murphy (2011), ‘House Prices and Credit Constraints: Making Sense of the US Experience’, The Economic Journal, 121(552), pp 533–551.
Fair RC (2004), Estimating How the Macroeconomy Works, Harvard University Press, Cambridge.
Fox R and P Tulip (2014), ‘Is Housing Overvalued?’, RBA Research Discussion Paper No 2014-06.
Gillitzer C and J Simon (2015), ‘Inflation Targeting: A Victim of Its Own Success?’, RBA Research Discussion Paper No 2015-09.
Girouard N, M Kennedy, P van den Noord and C André (2006), ‘Recent House Price Developments: The Role of Fundamentals’, OECD Economics Department Working Papers No 475.
Gitelman E and G Otto (2012), ‘Supply Elasticity Estimates for the Sydney Housing Market’, The Australian Economic Review, 45(2), pp 176–190.
Glaeser EL and CG Nathanson (2014), ‘Housing Bubbles’, NBER Working Paper No 20426.
Gonzalez L and F Ortega (2013), ‘Immigration and Housing Price Booms: Evidence from Spain’, Journal of Regional Science, 53(1), pp 37–59.
Himmelberg C, C Mayer and T Sinai (2005), ‘Assessing High House Prices: Bubbles, Fundamentals and Misperceptions’, Journal of Economic Perspectives, 19(4), pp 67–92.
IMF (International Monetary Fund) (2018), ‘Housing Market Imbalances in Australia: Development, Prospects, and Policies’, in ‘Australia: Selected Issues’, IMF Country Report No 18/45, pp 44–63.
Jovanoski S, N Stoney and P Downes (1997), ‘Modelling the Dwelling Cycle in the Treasury Macroeconomic (TRYM) Model’, Paper presented at the 1997 Conference of Economists, Economic Society of Australia, Hobart, 29 September–1 October.
Kaplan G, K Mitman and GL Violante (2017), ‘The Housing Boom and Bust: Model Meets Evidence’, NBER Working Paper No 23694.
Kendall R and P Tulip (2018), ‘The Effect of Zoning on Housing Prices’, RBA Research Discussion Paper No 2018-03.
Kohler M and M van der Merwe (2015), ‘Long-Run Trends in Housing Price Growth’, RBA Bulletin, September, pp 21–30.
Krugman P (2005), ‘That Hissing Sound’, The New York Times online, 8 August, viewed 22 January 2019. Available at <https://www.nytimes.com/2005/08/08/opinion/that-hissing-sound.html>.
Krugman P (2014), ‘Notes on Easy Money and Inequality’, The New York Times online, 25 October, viewed 22 January 2019. Available at <https://krugman.blogs.nytimes.com/2014/10/25/notes-on-easy-money-and-inequality/>.
Larkin MP, Z Askarov, C Doucouliagos, C Dubelaar, M Klona, J Newton, TD Stanley and A Vocino (2018), ‘Do House Prices Sink or Ride the Wave of Immigration?’, Institute of Labor Economics, IZA Discussion Paper No 11497.
Liu XL and G Otto (2014), ‘The Elasticity of Housing Supply in Regional NSW’, Paper presented at the Economic Measurement Initiative of the Centre for Applied Economic Research Annual International Workshop, University of New South Wales Business School, Sydney, 5 December.
Mayer CJ and CT Somerville (2000), ‘Residential Construction: Using the Urban Growth Model to Estimate Housing Supply’, Journal of Urban Economics, 48(1), pp 85–109.
McLaughlin RB (2011), ‘Metropolitan Growth Policies and New Housing Supply: Evidence from Australia's Capital Cities’, Australasian Journal of Regional Studies, 17(1), pp 60–80.
McLaughlin RB, T Sorensen and S Glavac (2016), ‘Intra-Metropolitan Housing Supply Elasticity in Australia: A Spatial Analysis of Adelaide’, Australasian Journal of Regional Studies, 22(3), pp 435–458.
Mishkin FS (2007), ‘Housing and the Monetary Transmission Mechanism’, in Housing, Housing Finance, and Monetary Policy, A Symposium sponsored by the Federal Reserve Bank of Kansas City, Kansas City, pp 359–413.
NHSC (National Housing Supply Council) (2014), ‘State of Supply Report: Changes in How We Live’, Unpublished manuscript. Available at <https://treasury.gov.au/publication/state-of-supply-report-changes-in-how-we-live/>.
Ong R, T Dalton, N Gurran, C Phelps, S Rowley and G Wood (2017), Housing Supply Responsiveness in Australia: Distribution, Drivers and Institutional Settings, AHURI Final Report No 281, Australian Housing and Urban Research Institute Limited, Melbourne.
Otto G (2007), ‘The Growth of House Prices in Australian Capital Cities: What Do Economic Fundamentals Explain?’, The Australian Economic Review, 40(3), pp 225–238.
Otto G and N Stapledon (2017), ‘How Predictable? Rent Growth and Returns in Sydney and Melbourne Housing Markets’, UNSW Business School Research Paper No 2017 ECON 01.
Oxford Economics (2016), ‘Forecasting UK House Prices and Home Ownership’, A Report for the Redfern Review into the Decline of Home Ownership, November.
Phibbs P, N Gurran, B Randolph and H Pawson (2017), ‘Institutional Thinking’, Letters, The Sydney Morning Herald, 24 November, p 21.
Powell AA and CW Murphy (1997), Inside a Modern Macroeconometric Model: A Guide to the Murphy Model, 2nd edn, rev and enlarged, with a contribution by N Conron, Springer-Verlag, Berlin.
RBA (Reserve Bank of Australia) (2014), ‘Submission to the Inquiry into Affordable Housing’, Submission to the Senate Economics Reference Committee Inquiry into Affordable Housing, February.
RBA (2018), ‘Box B: The Impact of Lending Standards on Loan Sizes’, Financial Stability Review, October, pp 32–36.
Saiz A (2007), ‘Immigration and Housing Rents in American Cities’, Journal of Urban Economics, 61(2), pp 345–371.
Treasury (Department of the Treasury) (2001), ‘Treasury Macroeconomic (TRYM) Model Overview’, viewed 13 February 2019. Available at <http://archive.treasury.gov.au/contentitem.asp?ContentID=235&NavID>.
Tumbarello P and S Wang (2010), ‘What Drives House Prices in Australia? A Cross-Country Approach’, IMF Working Paper WP/10/291.
Williams JC (2016), ‘Measuring the Effects of Monetary Policy on House Prices and the Economy’, in Expanding the Boundaries of Monetary Policy in Asia and the Pacific, Proceedings of a Conference held on 19–21 August 2015 in Jakarta, Indonesia and co-hosted by Bank Indonesia and the Bank for International Settlements, BIS Papers No 88, Bank for International Settlements, Basel, pp 7–16.
Williams R (1984), ‘An Australian Housing Model’, Economic Record, 60(2), pp 143–155.
Yates J (2011), ‘Housing in Australia in the 2000s: On the Agenda Too Late?’, in H Gerard and J Kearns (eds), The Australian Economy in the 2000s, Proceedings of a Conference, Reserve Bank of Australia, Sydney, pp 261–296.
Acknowledgements
We are especially grateful to Glenn Otto for detailed thoughtful comments. Thanks also to Tom Cusbert, Peter Downes, Luci Ellis, Liz Kendall, Matt Larkin, Dominic Meagher, Ewan Rankin, Dan Rees, Tom Rosewall, Paul Ryan, John Simon, Luke Willard and seminar participants at the Reserve Bank of Australia, Treasury, the Grattan Institute and the University of New South Wales. The views expressed in this paper are those of the authors and do not necessarily reflect the views of the Reserve Bank of Australia. The authors are solely responsible for any errors.
Footnotes
The Australian Bureau of Statistics (ABS) measure of building approvals (ABS Cat No 8731.0) is based on local council building permits. It should not be confused with development approvals, which typically occur months or even years earlier. [1]
For these and other figures, variable definitions and sources are given in Online Appendix D. [2]
By ‘sustained’ we mean that the shock lasts for at least as long as the effects that are shown and long-term interest rates move by the same amount as short-term rates. It is tempting to describe this change as ‘permanent’, except that permanent shifts in interest rates are not feasible in many macroeconomic models. [3]
In our simulations of the AUS-M model of the Australian economy, about half the effect of interest rates on GDP comprises dwelling investment. Unpublished simulations of MARTIN by Tom Cusbert find that housing accounts for about 30 per cent of the total response. Estimates for other countries seem to be somewhat smaller (e.g. Mishkin 2007), though consistent comparisons are difficult. As Paul Krugman (2014) writes, ‘one of the dirty little secrets of monetary policy is that it normally works through housing, with little direct impact on business investment’. [4]
One possibility is that changes in the price of existing dwellings largely reflect land prices. When land prices increase, higher-density housing becomes more attractive. Another possibility is that higher-density housing is more flexible than detached housing, which often requires land release and neighbourhood planning. However, it is difficult to reconcile these explanations with how quickly higher-density approvals respond. A more important factor may be variations in the availability of finance. This is because the sequential timing of cash flows for large developments makes their equity position quite sensitive to near-term price movements. [5]
As we show in Figure 6 below, interest rates also have only temporary direct effects on the level of dwelling investment in the MARTIN model. However, once effects on other expenditure components and their interactions are allowed for, MARTIN finds that interest rates have highly persistent effects on real GDP growth. So, while it is the change in interest rates that matters for dwelling investment (as a short-run approximation), it is the level of interest rates that matters for overall economic growth and the integral that matters for the level of overall activity. [6]
We are unaware of any aggregate time series data on the cost of new houses that includes land costs. The series we used for these exercises were constructed using ABS data on the cost of building new houses and data from a number of sources on land prices. [7]
Demolitions are estimated as the interpolated difference between accumulated completions and the census estimate of the number of dwellings. In projections, they are assumed to be a constant 8 per cent of completions. See Section B.3 in the Online Appendices for more details. [8]
Actual household size is highly endogenous, adjusting so as to keep demand and supply in approximate balance, so lacks predictive power. [9]
A percentage point increase in the vacancy rate reduces the annual growth of real rents by 2 percentage points initially and by 1.4 percentage points (= 0.001 x 400 / (1 – 0.72)) in the long run. [10]
Following Fox and Tulip (2014, Equation (2)), we define the user cost as:
where c is running costs; s is transaction costs; and d is the physical depreciation rate. After 2014, these are held constant. π is the expected real appreciation rate, assumed to equal its post-1955 mean. r is the average real expected variable mortgage rate, which Fox and Tulip estimated using 10-year fixed mortgage rates. However, subsequent data and analysis suggest that expected interest rate changes can be more reliably estimated from the yield curve on government bonds. From 1997 r is estimated as:
where vmr is the (package or average discounted) variable mortgage rate for owner-occupiers, bond is the 10-year government bond yield, cash is the cash rate, inflation is 10-year inflation compensation derived from indexed bonds and the superscript bar represents the sample mean, used to remove the average term premium from the yield curve. The average discounted rate is publicly available from 2005, prior to which we splice to the standard variable mortgage rate. Variable mortgage rates have closely followed the cash rate since 1997, but before then were noticeably less volatile. From 1982 to 1997, we assume the expected variable mortgage rate is a constant spread (equal to its 1997 level) to the 10-year government bond yield.
[11]The simplest alternative would be to assume lower expectations and include a constant ‘ownership premium’. This would be observationally equivalent. [12]
For examples of such reduced-form models, see Bourassa and Hendershott (1995), Abelson et al (2005), Caldera and Johansson (2013), Tumbarello and Wang (2010), and IMF (2018). [13]
Our most recent data, for June quarter 2018, imply that if prices are expected to grow at the same pace as in the past, then renting is about as expensive as buying (the user cost and the rental yield are both 3½ per cent), and hence that houses are fairly valued. If prices were expected to grow slower, as implied by our forecast in Online Appendix C, houses would be overvalued. We discuss further in Section 5.4. [14]
The semi-elasticity equals the inverse of the user cost, which in equilibrium will equal the price-to-rent ratio. See Fox and Tulip (2014, Equation (9)). [15]
This is the most recent period for which we have matched data. Estimates are seasonally adjusted and rounded. [16]
The RBA (2018) finds that borrowing limits are typically not binding. [17]
A sustained 1 percentage point reduction in the real cash rate raises the level of income by about 1.8 per cent after two years and about 3.7 per cent after five years. Thanks to Adam Hamilton for these estimates. [18]
Specifically, the 10-year government bond yield is the geometric average of the path for the cash rate over the coming ten years, which in our baseline projections follow zero-coupon forward rates. [19]
The most important nonlinearity is that housing prices, and hence activity, are more responsive to interest rates when interest rates are low. [20]
Technically, we show a reduction in real rents, whereas the text above refers to changes in nominal rents. Empirically, changes in the price level are quite small relative to the changes shown in Figure 12, so the distinction can be ignored. [21]
Short- and long-term interest rates move together, so disentangling their separate contributions is not clearly meaningful. Nevertheless, as an accounting exercise, the reduction in long-term rates has a larger effect on prices than the reduction in short-term rates, while short-term rates are more important for investment. [22]
A small part of the increase in measured population growth represents a broadening of the definition of long-term migration, which increased net overseas migration by 19,000–29,000 a year (ABS 2007, Tables 2.6 and 2.7). [23]
Whereas estimates of the elasticity of supply vary between and within countries, reflecting differences in land use regulations, estimates of the elasticity of demand reflect household preferences and might be expected to be similar. [24]