RDP 2015-05: The Social Costs of Currency Counterfeiting 5. Robustness of Results
May 2015 – ISSN 1448-5109 (Online)
- Download the Paper 881KB
5.1 Alternative Model Specifications
This section discusses the robustness of our results to different model specifications. Figure 4 compares the response of each variable to a counterfeiting shock in our baseline model to the responses using two alternative model specifications: first we introduce the savings rate into the model; second we model variables in flows rather than stocks.[21] These two alternative model specifications test the robustness of our interpretation of the model results as substitution effects. It is possible that the increase in bank deposits in response to the counterfeiting shock reflects the public converting cash used as savings, or a store of value, into bank deposits. It is also possible that the response could reflect the public taking out less cash than they otherwise would. However, the results of this robustness analysis suggest that our baseline results are representative of changes in the public's payments decisions rather than changes in savings behaviour.
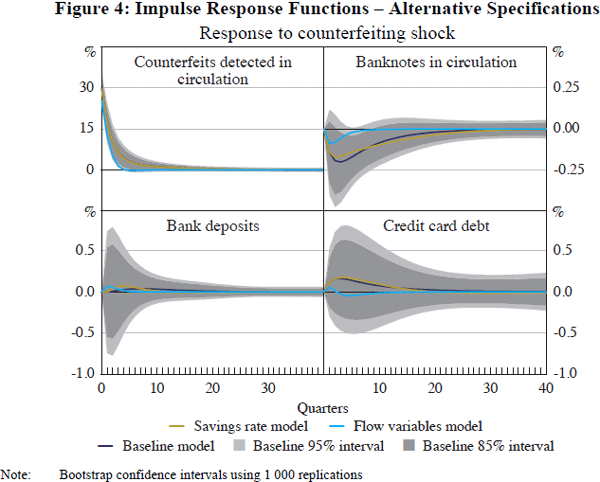
First, we estimate the model including the savings rate as an additional endogenous variable. This alternative specification captures possible changes in savings behaviour through the savings rate, and leaves the response of each variable to a counterfeiting shock to be interpreted as changes in payment preferences. The responses in all variables are broadly similar to the baseline, which gives us confidence that our interpretation of the impulse response functions as substitution effects between payment methods is plausible.
Second, we estimate a model with the endogenous variables in the form of flows as opposed to stocks. We use debit card expenditure and credit card expenditure rather than bank deposits and credit card debt (see Appendix A for details). These variables directly capture the effect of payments activity. The model implies responses smaller in magnitude for all variables compared to the baseline model. The results are qualitatively similar, with the exception of credit card debt which declines in the medium term, causing the cumulative effect to be slightly negative. All responses lie within the 85 per cent and 95 per cent confidence intervals of the baseline model.
5.2 Credit Card Fraud
It is possible that fraud in other payment mechanisms could affect the identification of the counterfeiting shock in our model. For example, our expectation is that credit card fraud would have a negative effect on credit card use. However, if counterfeiting and credit card fraud are contemporaneously correlated – for example, via a generalised increase in criminal activity of this nature – the positive response of credit card debt to the counterfeiting shock in our model could be biased. A useful thought experiment is to consider the effect of simultaneous shocks to counterfeiting and credit card fraud. We would expect the counterfeiting shock to reduce the demand for banknotes and increase the aggregate use of credit cards, while the credit card fraud shock would reduce the aggregate use of credit cards. To the extent that counterfeiting and credit card fraud are found to be contemporaneously correlated, the impulse response functions in Figure 3 could be negatively biased.
The Australian Payments Clearing Association (APCA) publishes year-ended data on credit card fraud for every financial year and calendar year. There are 16 observations in the data between June 2006 and December 2013, which does not provide enough degrees of freedom for use as a separate endogenous variable in the structural VAR model. While this would enable separate identification of counterfeiting shocks and credit card fraud shocks and could perhaps produce unbiased estimates, the credit card fraud data upon first inspection appears to lag counterfeiting activity in both log levels and log differences (Figures 5 and 6). While this relationship between counterfeiting and credit card fraud is most likely spurious, we mention it here to support our model identification assumption of the counterfeiting shock.
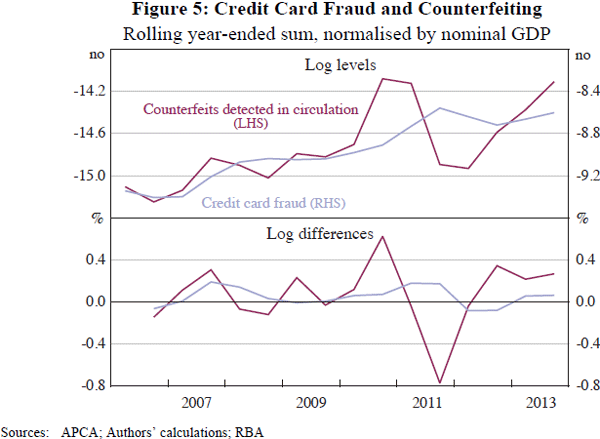
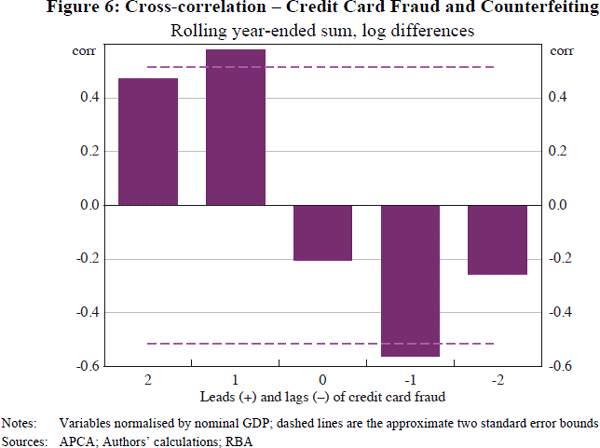
Footnote
In separate robustness analyses, we also performed the following variations of the model, with either similar or inconclusive results: different lag lengths; data adjusted for structural breaks; various deposit account rates as an alternative to the cash rate to capture the opportunity cost of holding currency; different monetary aggregate variables; credit card debt not accruing interest as opposed to total credit card debt; payments system variables associated with currency demand models such as eftpos terminals, ATMs per capita, and ATM withdrawals; and, vector error correction models to account for possible cointegrating relationships. [21]