RBA Annual Conference – 2005 Assessing the Sources of Changes in the Volatility of Real Growth Stephen G Cecchetti, Alfonso Flores-Lagunes and Stefan Krause[1]
Abstract
In much of the world, growth is more stable than it once was. Looking at a sample of 25 countries, we find that in 16, real GDP growth is less volatile today than it was 20 years ago. And these declines are large, averaging more than 50 per cent. What accounts for the fact that real growth has been more stable in recent years? We survey the evidence and competing explanations and find support for the view that improved inventory management policies, coupled with financial innovation, adopting an inflation-targeting scheme and increased central bank independence have all been associated with more stable real growth. Furthermore, we find weak evidence suggesting that increased commercial openness has coincided with increased output volatility.
1. Introduction
Today the world's economies appear to be much calmer than they were just a quarter-century ago. At the beginning of the 1980s, nearly two-thirds of the countries in the world were experiencing inflation in excess of 10 per cent per year. Today, it is one in six. Growth has risen as well. Two decades ago nearly one country in three was contracting. Today, five in six countries are growing at a rate in excess of 2 per cent per year.[2] But this is not the end of the story. Not only is inflation lower and output higher, they both appear to be more stable. The question is why.
Declines in the level and volatility of inflation are not that much of a mystery. The answer is almost surely better policy. Substantial changes in the operational framework of central banks over the past few decades have produced better inflation outcomes. Increased independence, as well as improved accountability and transparency have all played a role.[3] In an earlier paper, we find that improved monetary policy has been the driving force behind the better economic performance of the past decade.[4] But there we focus on weighted averages of output and inflation variability, and usually on cases in which inflation variability has a relatively high weight. Concluding that low and stable inflation is a consequence of better monetary policy is, therefore, not a big surprise.
In this paper we move to an examination of output volatility alone. Using techniques pioneered by McConnell and Perez-Quiros (2000) in their study of US GDP, we confirm the basic finding that the volatility of output growth has declined.[5] In fact, it has fallen in 16 of the 25 countries we study – it is unchanged in 9. And on average, for the countries in which it fell, the standard deviation of innovations to output growth has been cut in half. But, as we will discuss in more detail, the timing of the decline in volatility is far from synchronised.
Documenting the fact that the world has become more stable is only the first step. We go on to survey various possible explanations. There are five major ones:
- improved inventory management policies; cited by Kahn and McConnell (2005), Kahn, McConnell and Perez-Quiros (2002), McConnell and Perez-Quiros (2000), and McConnell, Mosser and Perez-Quiros (1999);
- better monetary policy, as discussed in Clarida, Galí and Gertler (2000) and our previous work;
- financial innovation and improvements in risk sharing, as discussed in Dynan, Elmendorf and Sichel (2005);
- increased international commercial openness, as suggested in Barrell and Gottschalk (2004); and
- luck in the form of smaller shocks, the answer given by both Ahmed et al (2004) and Stock and Watson (2002).
Additional explanations include the change in the composition of output, away from more volatile manufacturing and toward more stable services, and that reduced volatility is a consequence of changes in the methods used to construct the data.
The evidence is broadly consistent with improved inventory policy accounting for some portion of the decline in all 12 countries where we have the appropriate data. The better monetary policy hypothesis fares substantially worse, accounting for declines in output volatility in 10 of the 24 countries for which we have results. This is unsurprising given the fact that monetary policy faces a trade-off between inflation and output volatility, and that in the past two decades we have witnessed a dramatic shift towards keeping inflation low and stable.
While we have something to say about the implications of increased openness, our focus is primarily on the likely impact of financial innovation. To foreshadow our conclusions, we find that the volatility of output falls as a country's financial system becomes more developed and its central bank becomes more independent. Volatility fell by more in countries where credit became more readily available. Furthermore, we find weak evidence that more commercial openness, as measured by the ratio of imports plus exports to GDP, is negatively correlated with volatility across countries.
The remainder of the paper is divided into four parts. In Section 2 we outline the econometric testing procedures used to identify breaks in the volatility of output growth, and then report the results for both the timing and size of the changes in volatility. Section 3 presents a discussion of the numerous candidate explanations for the changes in output volatility, and Section 4 presents the second stage of our empirical analysis, where we present evidence in an attempt to distinguish them. Section 5 summarises our conclusions. Unfortunately, our analysis is sufficiently crude that we are only able to establish a set of correlations that are suggestive of which way to go next.
2. Identifying and Estimating the Changing Volatility of Growth
We begin our analysis by looking for structural breaks in the volatility of GDP growth. We do this in a series of steps. First, we estimate an equation of the form
where yt is the log of real GDP or the price level, Δ indicates the first difference, µ is a constant, ρ is a parameter representing the persistence of GDP growth, and ε is an innovation that is independent over time, but need not be identically distributed. Equation (1) is estimated allowing for breaks in the mean and persistence of output growth.
The result of this first step is a series of estimated residuals, . As noted by McConnell
and Perez-Quiros (2000), the transformed residuals,
, are unbiased estimators
of the standard deviation of εt. Using these, we
proceed to the second step, which is to search for breaks in an equation of
the following form:
That is, we look for breaks in the mean (α) of scaled absolute value of the estimated residuals from the simple regression (1), after allowing for the possibility of structural breaks in µ and ρ. (The details of the econometric procedures, which require a number of decisions, are described in Appendix A.)
We examine shifts in the volatility of growth in 25 countries. Briefly, we begin by taking the first-difference of deviations of the log of real GDP from an HP-filtered trend, then look for breaks in persistence and, conditional on those, search for breaks in volatility. This is exactly equivalent to studying the deviations of growth from a time-varying mean. Where available, we use quarterly data starting in 1970.[6] The results for this exercise are reported in Table 1. First, note that we identify at least one break in persistence for 10 of the 25 countries, with two breaks for two countries. We then find at least one break in volatility in all but 9 countries (Austria, Belgium, Chile, France, Japan, Mexico, Norway, Peru and Switzerland), and two breaks in 6 of the 25 countries we study (Netherlands, New Zealand, South Africa, Spain, Sweden and the UK). We allow for as many as five breaks, but in no country do we find more than two. While our dating of the breaks suggests that persistence and volatility often change simultaneously within a country, these dates are not synchronised across countries. Of the total of 22 breaks in volatility that we identify, only one takes place in the 1970s, 12 are in the 1980s, and another 9 are in the 1990s.[7]
Country | Persistence | Volatility | |||
---|---|---|---|---|---|
1st break | 2nd break | 1st break | 2nd break | ||
Australia | 1981:Q3*** | 1984:Q3*** | |||
Austria | none | none | |||
Belgium | none | none | |||
Canada | 1980:Q4* | 1987:Q2*** | |||
Chile | none | none | |||
Denmark | none | 1994:Q3*** | |||
Finland | none | 1995:Q2* | |||
France | none | none | |||
Germany | none | 1993:Q3*** | |||
Greece | none | 1991:Q1*** | |||
Israel | none | 1985:Q2** | |||
Italy | 1979:Q4** | 1983:Q3*** | |||
Japan | none | none | |||
Mexico | 1984:Q1*** | 1995:Q1*** | none | ||
Netherlands | 1986:Q3* | 1983:Q4* | 1994:Q3* | ||
New Zealand | none | 1975:Q3* | 1987:Q3*** | ||
Norway | none | none | |||
Peru | none | none | |||
South Africa | 1976:Q4*** | 1986:Q3*** | 1996:Q3* | ||
South Korea | 1992:Q2* | 1980:Q3** | |||
Spain | 1980:Q2*** | 1992:Q2** | 1985:Q2*** | 1993:Q2*** | |
Sweden | 1992:Q2*** | 1984:Q3*** | 1993:Q1*** | ||
Switzerland | 1980:Q1*** | none | |||
UK | none | 1981:Q2*** | 1991:Q4*** | ||
US | none | 1984:Q2*** | |||
Notes: Breaks are estimated using the first-difference of deviations of
log real GDP from an HP-filtered trend, conditional on possible breaks
in persistence. See Appendix
A for details. All sample periods end in 2003:Q4. Sample
period begins in 1970 for all countries except Austria (1976), Belgium
(1980), Chile (1980), Denmark (1978), Finland (1975), Israel (1980), Mexico
(1980), the Netherlands (1977),
Peru (1980), and Switzerland (1972). Source: authors' calculations |
Figure 1 plots the volatility of output before and after the estimated break dates. Volatility declined for all countries for which we identified a single break. There was also a steady decline in output volatility in five of the countries for which we identified two breaks (the Netherlands, New Zealand, South Africa, Sweden and the UK), while Spain experienced an increase in volatility after the first break, and then a decline following the second break. None of the countries experienced an increase in the standard deviation of growth in the last period as compared to the first. Across all countries, the declines ranged from just over 10 per cent for Spain to almost 80 per cent for New Zealand (combining the two breaks). The average decline from the beginning to the end of each country's sample was close to 50 per cent. In other words, these are not small numbers.
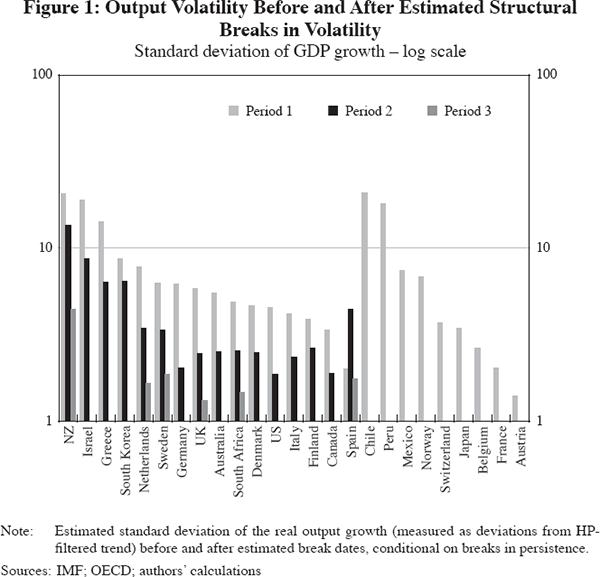
3. Explaining the Decline in the Volatility of Growth
Previous authors have delineated five possible explanations for the observed decline in output volatility. These include shifts to just-in-time inventory control methods, improvements in monetary policy, financial innovation, increases in openness to international trade, and luck. We summarise each of these, together with a discussion of some of the evidence drawn from the US case. In the next section, we explore the possible explanations for the cross-country declines in volatility documented in the previous section.
Before getting started, there are two hypotheses that we do not investigate or discuss: that the change in the variability of growth is a result of changes in fiscal policy or that it is an artefact of a change in data construction techniques. Both of these have been dismissed in the US case (see the appendix to Dynan et al 2005 for a summary). Data construction techniques have not changed all that markedly in the past 30 years and there is little evidence that the stabilising ability of fiscal policy has improved.[8] This still leaves a set of five possible explanations.
3.1 Changes in inventory control policies
Inventory changes account for a very small portion of GDP, averaging about ½ per cent and rarely exceeding 1 per cent of the total; they account for virtually none of trend growth. Even so, changes in private inventories account for something like 20 per cent of the volatility in quarterly GDP growth. From 1959 to 2003 the standard deviation of quarterly US total GDP growth, measured at a quarterly rate, was approximately 1 percentage point. Excluding inventory changes, this falls to 0.8 of a percentage point.
Given the importance of inventories in aggregate fluctuations, changes in inventory management policies could easily have an impact on the volatility of GDP. Improvements in technology that allow flexible production, smaller batch sizes, better monitoring of real-time sales, and the like have created substantial opportunities for reduced volatility. Today, an automobile assembly plant keeps only a few hours worth of parts on hand – the rest are in transit to the factory, timed to arrive at just the right moment. Similarly, a supermarket or superstore like Wal-Mart or Target will hold only one to two days’ supply of most products. The result is a great deal of flexibility in responding to changes in demand and sales.[9]
McConnell and Perez-Quiros (2000), Kahn et al (2002), and Kahn and McConnell (2005) marshal evidence in support of the view that changes in inventory management policies are the source of output's increased stability. They begin by noting that the volatility of output growth in the durable goods sector has fallen dramatically, but that the variance of final sales growth has not. McConnell and co-authors then show that inventory levels have fallen noticeably, and that the decline was most pronounced in the mid 1980s.[10] This is clearly consistent with the results in Table 2, which provides an accounting of the likely sources of the change in the variance of real growth in the US.
1959–1983 | 1984–2003 | Decline | |
---|---|---|---|
Variance of real GDP | 1.23 | 0.30 | 0.93 |
Fraction of decline in variance attributable to: | |||
Component | GDP excluding component | (Twice) covariance | |
Consumption | |||
Durable goods | 0.04 | 0.69 | 0.28 |
Non-durable goods | 0.03 | 0.86 | 0.11 |
Services | 0.03 | 0.88 | 0.09 |
Investment | |||
Non-residential | 0.03 | 0.74 | 0.24 |
Residential | 0.06 | 0.83 | 0.11 |
Change in private inventories | 0.35 | 0.54 | 0.12 |
Net exports | 0.05 | 1.16 | −0.20 |
Government | 0.05 | 0.94 | 0.00 |
Federal | 0.04 | 1.00 | −0.04 |
State & local | 0.01 | 0.94 | 0.05 |
Notes: The table shows the decomposition of the change in the variance
of GDP growth from 1959–1983 to 1984–2003 into the variance
of each component (individually), the variance of GDP excluding the component
and twice the covariance of the two. This is done by noting that: var(x+y) = var(x) + var(y) + 2cov(x,y).
The variances and covariances are then scaled by the overall change in
GDP variance, so that each row sums to 1.0. Source: <www.bea.gov/bea/dn/home/gdp.htm> |
The standard deviation of quarterly real GDP growth (measured at a quarterly rate) dropped by 0.56 percentage points, from 1.11 to 0.55. Table 2 examines the decomposition of the variance of quarterly real GDP growth (which fell from 1.23 to 0.30) into the portion that can be accounted for by various components. Arithmetically, the fall in the variance in GDP can be a consequence of the change in the variance of an individual component, the change in the variance of GDP excluding that component, or the change in (twice) the covariance of the component and GDP excluding the component. Each row of the table shows the fraction of the change of the variance accounted for by each of these. To see which components matter, look for rows in which the first column is big and the second column is far from 1. Inventories have that property. Looking at the covariances, we see that there is a role for non-residential construction and durable goods as well.
Cross-country comparisons point in the same direction. Table 3 reports the change in GDP volatility and the change in the volatility of the contribution to growth[11] attributable to inventory accumulation for a subset of 12 countries in our sample.[12] In all 12 cases, the decline in the standard deviation of the contribution of inventory changes to GDP growth is large. Furthermore, it is usually a substantial fraction of the overall decline in volatility, accounting, on average, for nearly 60 per cent of the decline in output growth volatility across countries.
Country | Beginning of sample | Break date | Standard deviation of quarterly GDP growth at quarterly rate | Standard deviation of growth contribution from inventories accumulation | |||||
---|---|---|---|---|---|---|---|---|---|
First sub-period | Second sub-period | Difference | First sub-period | Second sub-period | Difference | ||||
Australia | 1974:Q3 | 1984:Q3 | 1.34 | 0.69 | 0.65 | 0.92 | 0.73 | 0.19 | |
Canada | 1979:Q1 | 1987:Q2 | 1.05 | 0.65 | 0.41 | 0.78 | 0.59 | 0.19 | |
Denmark | 1978:Q1 | 1994:Q3 | 1.20 | 0.67 | 0.53 | 1.59 | 0.96 | 0.64 | |
Germany | 1970:Q1 | 1993:Q3 | 1.78 | 0.56 | 1.22 | 0.73 | 0.48 | 0.25 | |
Italy | 1970:Q1 | 1983:Q3 | 1.09 | 0.64 | 0.45 | 1.06 | 0.86 | 0.20 | |
Netherlands | 1983:Q4 | 1994:Q3 | 0.86 | 0.57 | 0.29 | 1.14 | 0.65 | 0.49 | |
New Zealand | 1975:Q3 | 1987:Q3 | 3.41 | 1.18 | 2.23 | 1.85 | 1.03 | 0.82 | |
South Korea | 1970:Q1 | 1980:Q3 | 2.39 | 1.69 | 0.70 | 1.38 | 0.88 | 0.50 | |
Spain | 1985:Q2 | 1993:Q2 | 1.24 | 0.46 | 0.77 | 1.16 | 0.88 | 0.28 | |
Sweden | 1984:Q3 | 1993:Q1 | 0.95 | 0.43 | 0.52 | 0.92 | 0.81 | 0.11 | |
UK | 1981:Q2 | 1991:Q4 | 0.73 | 0.36 | 0.37 | 0.81 | 0.49 | 0.32 | |
US | 1970:Q1 | 1984:Q2 | 1.20 | 0.51 | 0.69 | 0.55 | 0.37 | 0.18 | |
Notes: For countries in which there are two breaks, the ‘beginning of sample’ is the date of the first break. Changes in inventories are the series labelled ‘stockbuilding’. Real GDP is volume data at market prices. All data are real, seasonally adjusted. Break dates are determined by the procedure described in the text, using the first difference of the HP-filtered log of GDP, starting in 1970. Source: OECD Economic Outlook No 76 |
The natural interpretation of these results has a potential flaw arising from the possibility that the increased stability of inventories could be a consequence of more stable demand. When demand is stable (because either shocks are smaller or monetary policy is conducted more efficiently), firms see less reason to hold inventories. With smaller shocks overall, everything will be smoother.[13] This argument is the centrepiece of the work of Herrera and Pesavento (2004), who find that the volatility of both inventories and shipments has declined.
3.2 Better monetary policy
The second candidate explanation for the decreased volatility of output growth is that it is a result of improved monetary policy. Beginning in the mid 1980s, the structure of central banks changed in many parts of the world. There was an increase in independence and transparency, as well as a new-found commitment to low, stable inflation. And, as central bankers often emphasise, price stability is the foundation for high growth. In other words, inflation is bad for growth.
Today economists have a much better understanding of how to implement monetary policy than they did as recently as 20 years ago. To succeed in keeping inflation low and stable while at the same time keeping real growth high and stable, central bankers must focus on adjusting real interest rates either when inflation differs from its target level and/or when output deviates from potential output.
There are several pieces of evidence supporting the view that improved macroeconomic outcomes can be traced to better monetary policy. For the case of the US, Clarida et al (2000) show that the actions of the 1970s implied a policy reaction curve, or Taylor rule, in which inflation increases were met with insufficiently aggressive nominal interest rate increases. Under Chairman Arthur Burns, when inflation went up, the Federal Reserve increased their policy-controlled interest rate by less than one for one, so the real interest rate went down. The result was instability – both in inflation and output growth.
In an earlier paper, Cecchetti et al (2004), we develop a method for measuring the contribution of improved monetary policy to observed changes in macroeconomic performance and then use it to explain the observed increase in macroeconomic stability in a cross-section of countries. Our technique involves examining changes in the variability of inflation and output over time. We estimate a simple macroeconomic model of inflation and output for each of 24 countries, and use it to construct an output-inflation variability efficiency frontier. Specifically, for each country we specify the dynamics of inflation and output as a function of the interest rate – our measure of the central bank policy instrument – and some additional exogenous variables. Using the estimated model, we are able to compute the output-inflation variability frontier describing the best possible outcomes that a policy-maker can hope to achieve. Movements toward this frontier are interpreted as improvements in monetary policy efficiency. Our estimates suggest that improved monetary policy has played a greater stabilising role in 21 of the 24 countries (even though the comparison is between a base period – 1983–1990 – when many observers believe monetary policy had already greatly improved in many countries).[14] Seventeen countries experienced reduced supply shock variability, but overall this had a modest impact on performance.
Table 4 is derived from the results in that paper. However, in the current exercise we assume that the sole objective of monetary policy is to focus on output stability. The columns labelled ‘Output volatility, actual’ report the observed decline in the volatility of output growth (measured using industrial production) from the 1980s to the 1990s. Output volatility fell in 14 of the 24 cases. Next, in the columns labelled ‘Output volatility, minimising’, the table reports the minimum attainable variance of output computed from an estimated structural model. This is the best performance that could have been obtained if policy-makers focused all of their attention on output stabilisation (and none on inflation stabilisation). In all but six of these cases, the best attainable outcome was lower output volatility in the second period, so innovation variances fell – this reflects either some ‘good luck’ (that is, smaller shocks) or the presence of favourable structural changes that reduced the effect of shocks in the economy. The difference between these two – the change in actual minus the change in minimal output volatility – is a measure of policy effectiveness. We do not report this difference to simplify the table presentation. The final column shows the proportion of the volatility change that can be attributed to policy; a negative number here implies that policy contributed to an increase in output volatility.
Country | Output volatility, actual | Output volatility, minimising | Proportion of improved performance due to better policy | |||||
---|---|---|---|---|---|---|---|---|
1983–90 | 1991–98 | Decline | 1983–90 | 1991–98 | Decline | |||
Australia | 5.49 | 2.21 | 3.28 | 2.19 | 0.53 | 1.66 | 0.49 | |
Austria | 5.41 | 8.80 | −3.39 | 0.51 | 2.03 | −1.52 | −0.55 | |
Belgium | 4.05 | 6.19 | −2.14 | 1.63 | 2.48 | −0.85 | −0.60 | |
Canada | 8.20 | 5.76 | 2.44 | 2.12 | 0.56 | 1.56 | 0.36 | |
Chile | 68.29 | 14.02 | 54.27 | 26.27 | 3.38 | 22.90 | 0.58 | |
Denmark | 7.53 | 7.19 | 0.34 | 3.87 | 3.11 | 0.75 | −1.23 | |
Finland | 5.69 | 11.94 | −6.25 | 1.46 | 1.52 | −0.06 | −0.99 | |
France | 2.62 | 4.31 | −1.69 | 0.61 | 1.75 | −1.14 | −0.33 | |
Germany | 3.99 | 6.82 | −2.83 | 1.51 | 1.05 | 0.46 | −1.16 | |
Greece | 5.47 | 1.99 | 3.48 | 3.34 | 1.13 | 2.21 | 0.36 | |
Ireland | 12.90 | 8.34 | 4.56 | 3.85 | 4.07 | −0.22 | 1.05 | |
Israel | 9.20 | 4.49 | 4.71 | 3.56 | 1.14 | 2.42 | 0.49 | |
Italy | 3.29 | 5.34 | −2.06 | 1.77 | 0.41 | 1.35 | −1.66 | |
Japan | 14.80 | 9.08 | 5.73 | 0.82 | 1.94 | −1.12 | 1.20 | |
Mexico | 9.20 | 16.11 | −6.91 | 3.97 | 2.94 | 1.03 | −1.15 | |
Netherlands | 4.38 | 3.23 | 1.15 | 2.37 | 1.09 | 1.28 | −0.12 | |
New Zealand | 13.83 | 10.92 | 2.91 | 6.31 | 2.38 | 3.94 | −0.35 | |
Portugal | 7.89 | 16.97 | −9.08 | 3.72 | 3.22 | 0.50 | −1.06 | |
South Korea | 21.83 | 16.53 | 5.30 | 8.46 | 4.69 | 3.77 | 0.29 | |
Spain | 3.03 | 8.54 | −5.52 | 1.90 | 0.84 | 1.06 | −1.19 | |
Sweden | 5.69 | 12.73 | −7.04 | 4.07 | 3.25 | 0.82 | −1.12 | |
Switzerland | 10.15 | 4.98 | 5.17 | 5.09 | 2.94 | 2.15 | 0.58 | |
UK | 3.64 | 2.90 | 0.74 | 1.38 | 0.38 | 1.00 | −0.36 | |
US | 4.10 | 1.75 | 2.35 | 1.24 | 0.17 | 1.07 | 0.54 | |
Notes: Actual output volatility is computed from the standard deviation of the growth in deviations of log industrial production from an HP-filtered trend. The column labelled ‘Proportion of improved performance due to better policy’ is the ratio of the (change in the actual – change in the optimal) to the ‘change in the actual’. Source: Computed using techniques described in Cecchetti et al (2004) |
Overall, the results suggest that policy was a stabilising force in only 10 of the 24 countries. In the remaining 14, the contribution of policy was to increase the volatility of output. This should come as no surprise since, as we show in our other paper, the primary impact of policy during this period was to stabilise inflation. By focusing on inflation stability, policy-makers moved along an output-inflation volatility frontier and made output more volatile, not less.
It is worth emphasising that it is likely to be very difficult to distinguish better policy decisions from a better institutional environment, regardless of the actual macroeconomic outcomes. As two of us discuss in Cecchetti and Krause (2001), the acumen of policy-makers is irrelevant if they are operating in an institutional environment in which monetary policy is ineffective. There are a number of examples of changes that improve the ability of policy-makers' actions to influence inflation and output. The traditional ones include the degree of a central bank's political independence and the implementation of explicit inflation-targeting regimes. As noted by Krause and Méndez (2005), these sorts of institutional changes, as well as membership of the European Monetary Union, are associated with higher relative preference for inflation stability. For a country operating on its inflation-output variability frontier, this could lead to an increase in output volatility.[15]
Changes in financial structure can also influence the efficacy of monetary policy. For example, movements away from a government-controlled banking system can result in improved macroeconomic outcomes that are likely to be indistinguishable from those that come from improved policy-making itself. With that in mind, we now turn to a discussion of changes in the financial system.
3.3 Financial innovation
Dynan et al (2005) provide a detailed discussion of the potential link between the decline in the volatility of US GDP growth and American financial innovations of the 1980s. These include the development of active secondary markets for loans (especially for home mortgages), the increased popularity of junk bonds, the phasing-out of deposit interest rate controls, regulatory changes aimed at creating access to credit for low-income households, and the eventual elimination of the prohibition on interstate banks.
The case of home mortgages provides an excellent example. Prior to the mid 1980s, households wishing to borrow for the purpose of purchasing a home had to obtain financing from a local financial intermediary. This meant that they were reliant on the ability of bankers to obtain sufficient deposit liabilities to provide the loan. If funds were plentiful in one locale, but scarce in another, there was no way for the funding to flow to where it was needed. The creation of asset-backed securities changed all of this.
In 1970, the Government National Mortgage Association (GNMA) issued the first mortgage-backed securities. These were pass-through securities composed of government guaranteed mortgages. The Federal National Mortgage Association (FNMA) then issued mortgage-backed securities backed by private insurance in 1981. Because of prepayment uncertainties, these initial asset-backed securities had durations that could not be computed with confidence. This problem was solved in 1983 when the Federal Home Loan Mortgage Corporation (FHLMC) issued the first tranched collateralised mortgage obligations (CMOs). CMOs divided the pool of mortgages into maturity categories based on when they are prepaid, and reduced the prepayment risk. The result was a very liquid mortgage market. McCarthy and Peach (2002) provide a detailed discussion of these changes to the US mortgage market, and find that it has damped the response of residential fixed investment to changes in monetary policy.
Today, mortgages are just the tip of the asset-backed security iceberg. With the exception of certain types of small-business loans, virtually every type of credit is securitised. This includes commercial and industrial loans, credit-card debt, student loans, and motor vehicle loans. The latter provide another interesting example. In early 2005 the business news reported the downgrading of US motor vehicle manufacturers. For example, Moody's and Standard and Poor's lowered General Motors long-term credit rating to the lowest investment grade level. At the same time, asset-backed car loans were receiving triple-A ratings. The default rate on these loans is predictable, so pools have very little risk in them.
All of this has come along with a dramatic increase in the use of debt by both households and businesses. Individuals can better smooth consumption in the face of short-term income variation, while firms can invest more steadily, even when faced with transitory revenue fluctuations.[16] Overall, risk is able to flow to those best able to bear it, thereby increasing the efficiency of the economy as a whole.
The improved ability of financial markets to efficiently distribute risk is consistent with Comin and Philippon's (2005) observation that firm-level volatility has risen even as aggregate volatility has fallen. In a world with poorly functioning financial markets, high transaction costs make it costly for investors to obtain diversified portfolios. As a result, they will push firms to diversify internally, creating large conglomerates like General Electric (GE). GE produces everything from light bulbs to power generating plants, jet engines and financial services. Diversification of this sort reduces the risk of the enterprise as a whole and is surely good for the managers of GE. And if financial transaction costs are high, it is good for investors, too. But as financial markets become deeper and more liquid, investors will prefer to choose their own portfolio weights for the different sectors, and there will be a push toward smaller firms with more volatility. At the same time, aggregate volatility will fall.
Returning to the case of households, Figure 2 provides some evidence that debt has improved the ability of households to smooth consumption in the face of income shocks. The figure plots the ratio of total US household debt to personal income together with the backward-looking five-year rolling standard deviation of consumption growth. These two series clearly have trends, but if we look at the changes we see that a 10 percentage point increase in the ratio of debt to income was associated with a decline of 50 basis points (0.5 of a percentage point) in consumption volatility over the following five years. That is, the impact is economically meaningful.[17] While we make no attempt to prove that increased debt has caused consumption to be smoother, we note that many of the legal and regulatory changes that allowed financial innovations to occur during the late 1980s and 1990s seem independent of consumption growth.
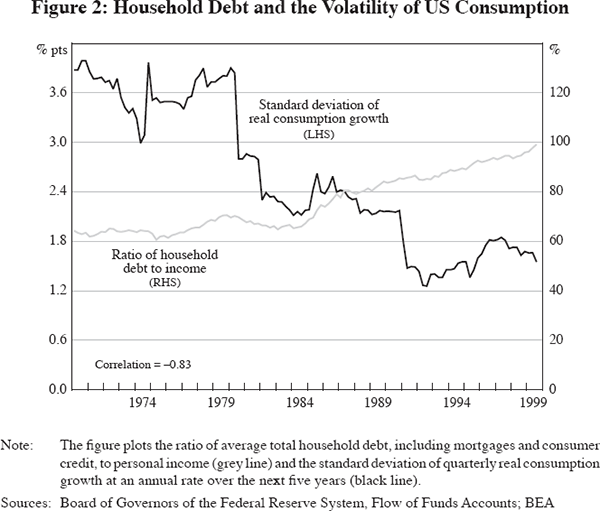
3.4 International openness
Over the last half of the 20th century, trade barriers were reduced or eliminated worldwide and transportation costs plummeted. The result has been a dramatic increase in the amount of cross-border trade in goods and services. In the US, for example, the ratio of imports plus exports to GDP has risen from just over 10 per cent in 1970 to 26 per cent today. Something similar has happened worldwide, with this measure of openness rising from 23 per cent in 1970 to 54 per cent in 2004.[18] With moves like the elimination of the multi-fibre agreement at the beginning of 2005, we can expect this trend to continue. More trade has also brought with it increased financial transactions. Current and capital account flows have both risen.
Greater commercial and financial openness can affect aggregate volatility in a number of ways. First, it provides an opportunity for international risk sharing – both purely financial and real. On the financial side, in the same way that mortgage financing in the US does not have to come from the geographic home of the borrower, now financing can come from outside a country.[19] Households, firms, and governments in one country now have access to funds from elsewhere in the world. In the same way, demand for real goods and services comes both from inside and outside a country. As the importance of trade flows increases, fluctuations in domestic aggregate demand become less important for domestic production.
A second mechanism by which openness can lower volatility is by allowing developed countries to send their more volatile industries offshore.[20] A developed country that is able to push its volatile manufacturing sector into the less-developed world will have a more stable domestic economy. As it turns out, this seems an unlikely explanation; the shift from goods to services in the US accounts for virtually none of the fall in the volatility of real growth.
These arguments also imply that larger countries could be more stable just because they are better diversified. Smaller economies, which are typically more open, may be more susceptible to certain shocks, given that their economic structure is more likely to be concentrated in a few industries. The result could be more, not less volatility. Emerging-market countries that are more open are more exposed to the impact of shocks arising from events like the Asian crisis of 1997. In the end, commercial openness could either raise or lower output volatility. We provide modest evidence for the former in the next section of the paper.
3.5 Smaller shocks
A number of authors conclude that improved macroeconomic performance, especially in the US, is a consequence of smaller shocks. Ahmed et al (2004) and Stock and Watson (2002) provide the most detailed arguments for this case. Their results are based on the following logic. Any stochastic model of the economy can be thought of as combining some shocks with a propagation mechanism. If output volatility has declined it is either a consequence of a change in the nature of shocks or a change in the propagation mechanism. Both sets of authors are unable to find changes in the latter, so they ascribe the observed stabilisation of the real economy to the former.[21]
There are a number of issues that arise in evaluating the case for luck. First, there is casual empirical evidence against it. It is difficult to argue that the stability of the 1990s was mere good fortune. Surely, the decade was not a calm one for the financial markets. Major economic crises occurred in Latin America and Asia, and Long-Term Capital Management nearly collapsed, paralysing the bond markets. Raw materials prices fluctuated wildly. The price of oil spiked at more than US$35 a barrel late in 1990, then plunged below US$12 a barrel at the end of 1998 before beginning a steady rise to US$30 a barrel by the beginning of 2000.
Second, the observation that the shocks hitting the economy have been effectively smaller is completely consistent with the view that stabilisation has been a consequence of improved monetary policy. One possibility, and the one consistent with the previous discussion, is that central bankers have both created smaller shocks of their own and succeeded in neutralising the shocks that they have seen. The Clarida, Galí and Gertler result is clearly of the first type. Their finding that policy-makers engaged in destabilising behaviour is consistent with the idea that central bankers were exacerbating, rather than ameliorating, shocks. In standard econometric analyses these will show up as the ‘monetary policy shocks’ identified from residuals in structural models.
Finally, Kahn and McConnell (2005) show that improved inventory control policies are also consistent with the finding of smaller shocks. The intuition of their result is the same as the one for monetary policy. Economic agents are doing a combination of neutralising external shocks and making smaller mistakes. Again, the result is increased stability. More generally, the problem is that any improved structural flexibility not explicitly captured in a simple macroeconomic model will be wrongly attributed by researchers to good luck.
4. Financial Development, Trade Openness, Central Bank Structure, and the Volatility Decline
In the previous section, we focused on possible explanations for the volatility decline in the US. The next step is to examine evidence for the panel of 25 countries. Is it possible to explain both the dispersion in the level of volatility of real growth across countries as well as the change within countries? To see, we look at the correlation of estimates of the standard deviation of real GDP growth with measures of central bank structure, financial development, commercial openness, and the absolute size of each country. (These measures are discussed in more detail in Table 5.)
Financial development and openness to trade |
---|
1. Private credit to GDP ratio: extent to which private sector activities are financed through bank lending. |
2. Trade in goods to GDP: the ratio of imports plus exports to GDP. |
Central bank structure |
3. Central bank independence (CBI): we compute an index that uses the average tenure of the central bank governor as a proxy for CBI as in Cukierman (1992) and de Haan and Kooi (2000). The turnover ratio of the central bank governor (TOR) has the advantage that it can be computed for a larger set of countries and for different periods, so it becomes technically possible to use it to construct a measure of CBI for the periods separated by the structural break. |
4. Inflation targeting: we construct the variable by dividing the number of years an inflation-targeting regime has been in place for a particular country, by the number of years of the respective sub-period. For the information on the dates that inflation targeting was introduced we employ the data from Mishkin and Schmidt-Hebbel (2002). |
Other variables |
5. Inflation volatility: the log of the standard deviation of inflation. |
To assess the sources of changes in output volatility we use a country-specific fixed-effects model, with the periods separated by the estimated structural breaks. So, for a given country we regress the difference in the standard deviation of real growth (measured as changes in deviations from the HP-filtered trend), before and after the estimated volatility break date, on the change in the right-hand-side variables computed by the same break date. In order to avoid problems associated with extreme values (see Figure 1), we take the log of the standard deviation of output innovations.
The results shown in Table 6 are quite striking. First, they suggest that a more developed financial system, measured by bank credit to the private sector, is associated with lower volatility in GDP growth. This outcome is consistent with the lending view: more developed financial markets increase the impact of a given change in monetary policy, making stabilisation efforts more successful. The first row of the table shows that increases in this financial development variable are associated with large declines in volatility, and the effects are estimated precisely (p-values are all 0.05 or less).
Explanatory variable | (1) | (2) | (3) | (4) |
---|---|---|---|---|
1. Private credit to GDP(a) | −1.73 (0.00) |
−1.49 (0.00) |
−1.44 (0.00) |
−1.35 (0.00) |
2. Trade in goods to GDP(b) | −2.03 (0.20) |
−1.04 (0.54) |
−0.58 (0.40) |
−0.17 (0.89) |
3. Central bank turnover ratio | 0.57 (0.63) |
−0.13 (0.87) |
||
4. Inflation targeting | −0.39 (0.10) |
−0.20 (0.39) |
||
5. Inflation volatility | 0.51 (0.01) |
0.46 (0.03) |
||
F-statistic for joint test | 13.38 (0.00) |
15.44 (0.00) |
20.91 (0.00) |
11.78 (0.00) |
Notes: p-values (in parentheses) are computed using standard errors
that are robust to heteroskedasticity. The F-statistics are for the joint
test that all of the slope coefficients in the regression are simultaneously
zero. Source: authors' calculations |
An example helps to reinforce the size of the estimated effects. For the case of South Korea, we identify a break in volatility in the third quarter of 1980. The ratio of private Korean credit rose from 48 per cent of GDP before the break to 102 per cent after. The estimates in Table 6 suggest that this doubling of credit would reduce the standard deviation of Korean GDP volatility by between 44 per cent and 56 per cent.[22] In fact, the volatility fell by half. From this we conclude that financial development has played an important role in reducing the volatility of output.
Second, commercial openness is negatively, but not significantly, correlated with fluctuations in GDP growth. This result is consistent with our previous discussion – commercial openness can either raise or lower output volatility.
Turning to the importance of monetary arrangements, we do not find evidence supporting the view that higher central bank independence, measured by a lower average turnover ratio of central bank governors, is correlated with lower output growth volatility. This outcome is consistent with the evidence provided by Cukierman (1992) and others. However, the results for inflation volatility suggest that the higher the variance of inflation, the higher the variance of output.
Finally, the analysis suggests that adoption of an inflation-targeting scheme is correlated with reductions in the volatility of real growth. One possible explanation for this is that adoption of a disciplined monetary policy framework helps central bankers to move the economy toward the efficient frontier, reducing both output and inflation volatility. The evidence suggests that this effect is larger than the one associated with the trade-off faced by the policy-maker who, under optimal or near-optimal policies, may only be able to reduce inflation volatility at the expense of increasing GDP growth fluctuations.
A potential criticism of the results in Table 6 is the fact that, by employing a fixed-effects model, we are only able to include countries for which we have econometrically identified structural breaks in the volatility of real growth. This means ignoring the information from 9 of the 25 countries in our sample. To address this problem, and include the entire sample of countries, we arbitrarily break our data into sub-periods and examine changes between the initial and final four years of the sample period common to all countries; that is, between the period 1980:Q1–1983:Q4 and the one from 2000:Q1 to 2003:Q4. This division has the advantage that 17 out of the 22 structural breaks fall within the middle period (1984:Q1–1999:Q4), suggesting that we have retained much of the integrity of the subdivision studied above.
Table 7 reports these results. This alternative subdivision of the data does not affect the main results – financial development is negatively and significantly correlated with the standard deviation of growth in real GDP, while the effect of openness to trade on output volatility remains insignificant. The only difference is that under this subdivision of the data, neither inflation targeting, nor inflation volatility seem to be correlated with the changes in growth fluctuations.[23]
Explanatory variable | (1) | (2) | (3) | (4) |
---|---|---|---|---|
1. Private credit to GDP(a) | −1.20 (0.00) |
−1.02 (0.00) |
−1.14 (0.00) |
−1.08 (0.00) |
2. Trade in goods to GDP(b) | −0.27 (0.74) |
−0.40 (0.61) |
−0.47 (0.56) |
−0.25 (0.76) |
3. Central bank turnover ratio | 1.25 (0.26) |
0.87 (0.52) |
||
4. Inflation targeting | −0.21 (0.19) |
−0.16 (0.49) |
||
5. Inflation volatility | 0.07 (0.52) |
−0.02 (0.86) |
||
F-statistic for joint test | 10.78 (0.00) |
10.86 (0.00) |
10.12 (0.00) |
6.13 (0.00) |
Notes: p-values (in parentheses) are computed using standard errors
that are robust to heteroskedasticity. The F-statistics are for the joint
test that all of the slope coefficients in the regression are simultaneously
zero. Source: authors' calculations |
5. Conclusion
While everyone who has looked agrees with the McConnell and Perez-Quiros (2000) observation that the volatility of real growth in the US fell by more than one-third in the mid 1980s, there is substantial disagreement over the causes of the decline. Is it inventory policy, monetary policy, or just luck? Could it be changes in financial development or possibly commercial openness? The purpose of this paper is to address these questions by examining data from a broad set of countries to see first, whether volatility changes occurred in the rest of the world, and second, to provide additional evidence to assess the causes of this change.
Our first result is that output volatility has fallen in a broad cross-section of countries; all of the 16 countries with at least one break experienced lower volatility in the more recent period. In assessing the causes of the change in the volatility of real growth, our primary findings link two previous results. For some time we have known that more stable economies grow faster.[24] We have also known that a sound financial system provides the foundation for economic development.[25] Countries with deeper, more sophisticated, financial systems grow faster. Our results show that financial development, as measured by the importance of bank lending, is linked to real economic stability.
Beyond the importance of financial development, we also provide evidence in favour of the view that improved inventory control policies played a role in the more stable growth that we have observed. Furthermore, increased commercial openness, measured by the ratio of imports plus exports to GDP, does not appear to be associated with more stable growth.
Finally, we should note that what we have done is established a set of correlations. Real volatility is negatively correlated with bank lending and positively correlated with the importance of trade flows. And a significant fraction of the decline in the volatility of real GDP, for those countries where it fell, can be accounted for by changes in the behaviour of inventory accumulation. What we have not done is show causal links. It is surely possible, for example, that financial systems are more prone to develop in countries that are more stable and that less stable countries may trade more. Determining the ultimate causes of these changes must be high on the agenda for future research.
Appendix A
Let Δyt denote the rate of growth of HP-filtered log real GDP. We assume a simple AR(1) model:
Our first step, for each country, is to search for multiple breaks (up to five) in the AR(1) coefficient, that is, persistence (ρ) in Equation (A1).
After finding any breaks in the persistence of Δyt, that model specification is used for the country
in obtaining the residuals . Then, following
McConnell and Perez-Quiros (2000), each set of residuals is assumed to follow
a normal distribution and the transformations
are unbiased estimators
of the standard deviation of
εt.[26]
Finally, we search for multiple breaks in the mean of the following volatility equation:
We search for multiple breaks in the different series above using the GAUSS code made available by Bai and Perron (2003) that is based on theoretical results in Bai and Perron (1998). The reason for considering tests for multiple breaks is that tests for a single break typically have low power in the presence of multiple breaks (Bai 1997 and Bai and Perron 2003).
Bai and Perron (1998, 2003) present a number of tests that are available in their GAUSS programs. To decide on the number of breaks and the break dates we employ the ‘sequential’ method described below, which is reported by Bai and Perron (2003) to outperform other methods, based on simulations they conduct. First, we estimate up to five breaks in the series for each country. Second, we use the method proposed by Bai and Perron (1998) based on the sequential application of the sup Ft(l+1|l) test, which is designed to detect the presence of (l+1) breaks conditional on having found l breaks (l = 0, 1,…, 5). The statistical rule is to reject l in favour of a model with (l+1) breaks if the overall minimal value of the sum of squared residuals (over all the segments where an additional break is included) is sufficiently smaller than the sum of squared residuals from the model with l breaks. The dates of the breaks selected are the ones associated with this overall minimum.[27] We identify a break (or an additional break) if the test statistic allows rejection of the null hypothesis at a 10 per cent level of significance or higher.
Appendix B
Data on private credit and trade on goods come from the World Bank World Development Indicators, December 2004 and from the Reserve Bank of Australia.
Turnover ratio of the central bank governor is constructed from information taken from each central bank's website, as well as inquiries to central bank staff.
Inflation targeting data are taken from Mishkin and Schmidt-Hebbel (2002).
GDP and CPI inflation data were obtained from the IMF International Financial Statistics CDROM (December 2004) and the OECD Economic Outlook No 76, December 2004.
Footnotes
Cecchetti: Brandeis University and NBER; Flores-Lagunes: University of Arizona; and Krause: Emory University. The authors would like to thank our discussant Mardi Dungey, as well as participants at the conference, especially David Wilcox. In addition, Margaret M McConnell, Anil K Kashyap, Jeffrey Miron and Leonardo Bartolini provided numerous helpful comments and discussions. [1]
All of these numbers are computed from the International Monetary Fund's World Economic Outlook database. [2]
For a detailed discussion of these issues see Cecchetti and Krause (2001, 2002). [3]
See Cecchetti, Flores-Lagunes and Krause (2004). [4]
For the US, the fact that the volatility of GDP growth has fallen since 1984 has been confirmed by virtually everyone who has looked at the data. See, for example, Ahmed, Levin and Wilson (2004), Nelson and Kim (1999), and Stock and Watson (2002). [5]
Our results are robust to the use of unfiltered GDP growth, assuming that we allow for breaks in the mean growth rate, µ in Equation (1), before testing for breaks in persistence. [6]
Our results are consistent with the timing of breaks identified by Smith and Summers (2002), who study Australia, Canada, Germany, Japan and the UK. [7]
We note, but do not investigate, the possibility that fiscal consolidations had an impact on the financial system, leaving it freer to accommodate private credit needs. [8]
Recent press reports suggest that these large retailers have gone even further, no longer holding their store inventories on their own books. For example, a tube of Procter and Gamble-produced toothpaste on a Wal-Mart store shelf will be on Procter and Gamble's books until it is sold to the final consumer. Only when they are sold does Wal-Mart actually pay for the items that are in their stores. This change in accounting has the potential to drive reported retail inventories to very low levels, as well as reducing the volatility of measured inventories. [9]
Ramey and Vine (2004b) take issue with the inventory-sales ratio evidence used by Kahn et al (2002), noting that the drop seen in the nominal data is not mirrored in the real data. That is, when looking at the ratio of real, deflated, inventories to real sales, the drop emphasised by Kahn et al is no longer apparent. [10]
Computationally, it is the growth of the component times the (lagged) share of that component in GDP. So, for example, if service consumption were to grow by 5 per cent, since it accounts for 40 per cent of total GDP, its growth contribution would be 5 x 0.4 = 2 per cent. [11]
We report results for all countries that both exhibit at least one break in volatility and for which the OECD reports inventory data. [12]
For a discussion see Ramey and Vine (2004a). [13]
In the cases of Austria, Germany and Switzerland we find that monetary policy contributed to increased volatility. This is likely a consequence of a combination of events including the fiscal and monetary consequence of German unification and preparations for the creation of the European Monetary Union. [14]
Cecchetti and Ehrmann (2002) find modest evidence that inflation-targeting countries experience slightly higher output volatility than non-inflation-targeting countries. [15]
Campbell and Hercowitz (2005) link the reduced volatility of output to the increase in household borrowing resulting from the relaxation of collateral constraints in the 1980s. They point to increases in the availability of home equity loans as a potentially important source of an individual's ability to smooth consumption in the face of income volatility. [16]
The t-statistic for the coefficient in a regression of the change in consumption volatility on the debt-to-income ratio is −1.7. [17]
These are the IMF World Economic Outlook aggregates. [18]
In their study of 24 OECD countries, Buch, Döpke and Pierdzioch (2002) find that business cycles are less pronounced in countries with more open financial markets. [19]
This would not lower volatility globally. Unfortunately, we do not have data to test this hypothesis. [20]
Ahmed et al reach their conclusion by noting that output can be written as an infinite-order moving average. The MA coefficients in this Wold representation correspond to a reduced form for coefficients in the transmission mechanism, and the innovations are simply the white noise shocks hitting the economy. Ahmed et al show that the primary source of stabilisation is the reduced magnitude of the shocks. This result is also consistent with the work of Arias, Hansen and Ohanian (2004), who suggest that the reduced volatility arises from a smaller variance of real shocks. [21]
The estimated impact is equal to the inverse of e raised to the power of the change in the credit to GDP ratio times the coefficient estimate from the first row of Table 6. [22]
We perform other robustness exercises, such as expanding the analysis to include the decade of the 1960s (data available for a number of countries only) and restricting the analysis to the post 1980 period and beyond. We also use a measure of growth volatility without applying the HP filter. Our main conclusions are robust to these alternative measures and definitions of time periods. [23]
See Ramey and Ramey (1995). [24]
See Ross Levin's (1997) survey. [25]
Footnote 3 of McConnell and Perez-Quiros (2000) indicates that this absolute value specification of the error is more robust to departures from conditional normality. See also Davidian and Carroll (1987). [26]
All testing procedures allow for serial correlation and different variances across segments in the residuals. In addition, the variance-covariance matrices used in constructing the various test statistics are robust to heterogeneity and autocorrelation by using Andrews (1991) automatic bandwidth with AR(1) approximation and a quadratic kernel. The residuals used are pre-whitened. [27]
References
Ahmed S, AT Levin and BA Wilson (2004), ‘Recent US macroeconomic stability: good policies, good practices, or good luck?’, Review of Economics and Statistics, 86(3), pp 824–832.
Andrews DWK (1991), ‘Heteroskedasticity and autocorrelation consistent covariance matrix estimation’, Econometrica, 59(3), pp 817–858.
Arias A, GD Hansen and LE Ohanian (2004), ‘Why have business cycle fluctuations become less volatile?’, UCLA (University of California, Los Angeles), unpublished manuscript.
Bai J (1997), ‘Estimating multiple breaks one at a time’, Econometric Theory, 13(3), pp 315–352.
Bai J and P Perron (1998), ‘Estimating and testing linear models with multiple structural changes’, Econometrica, 66(1), pp 47–78.
Bai J and P Perron (2003), ‘Computation and analysis of multiple structural change models’, Journal of Applied Econometrics, 18(1), pp 1–22.
Barrell R and S Gottschalk (2004), ‘The volatility of the output gap in the G7’, National Institute Economic Review, 188, pp 100–107.
Buch CM, J Döpke and C Pierdzioch (2002), ‘Financial openness and business cycle volatility’, Kiel Institute for World Economics Working Paper No 1121.
Campbell JR and Z Hercowitz (2005), ‘The role of collateralized household debt in macroeconomic stabilisation’, NBER Working Paper No 11330.
Cecchetti SG and M Ehrmann (2002), ‘Does inflation targeting increase output volatility? An international comparison of policy-makers' preferences and outcomes’, in N Loayza and K Schmidt-Hebbel (eds), Monetary policy: rules and transmission mechanisms, Proceedings of the Fourth Annual Conference of the Central Bank of Chile, Santiago, pp 247–274.
Cecchetti SG, A Flores-Lagunes and S Krause (2004), ‘Has monetary policy become more efficient? A cross-country analysis’, NBER Working Paper No 10973.
Cecchetti SG and S Krause (2001), ‘Financial structure, macroeconomic stability and monetary policy’, NBER Working Paper No 8354.
Cecchetti SG and S Krause (2002), ‘Central bank structure, policy efficiency and macroeconomic performance: exploring empirical relationships’, Federal Reserve Bank of St. Louis Economic Review, 84(4), pp 47–59.
Clarida R, J Galí and M Gertler (2000), ‘Monetary policy rules and macroeconomic stability: evidence and some theory’, Quarterly Journal of Economics, 115(1), pp 147–180.
Comin D and T Philippon (2005), ‘The rise in firm-level volatility: causes and consequences’, NBER Working Paper No 11388.
Cukierman A (ed) (1992), Central bank strategy, credibility, and independence: theory and evidence, MIT Press, Cambridge, pp 347–455.
Davidian M and RJ Carroll (1987), ‘Variance function estimation’, Journal of the American Statistical Association, 82(400), pp 1079–1091.
de Haan J and W Kooi (2000), ‘Does central bank independence really matter? New evidence for developing countries using a new indicator’, Journal of Banking and Finance, 24(4), pp 643–664.
Dynan KE, DW Elmendorf and DE Sichel (2005), ‘Can financial innovation explain the reduced volatility of economic activity?’, paper presented at the Carnegie-Rochester Conference Series on Public Policy (Financial Innovation, Risk and Fragility), University of Rochester, 15–16 April.
Herrera AM and E Pesavento (2004), ‘The decline in US output volatility: structural changes and inventory investment’, Emory Economics Working Paper No 0301. Forthcoming in Journal of Business and Economic Statistics.
Kahn JA and MM McConnell (2005), ‘The decline in US output volatility: what's luck got to do with it?’, Federal Reserve Bank of New York, unpublished manuscript.
Kahn JA, MM McConnell and G Perez-Quiros (2002), ‘On the causes of the increased stability of the US economy’, Federal Reserve Bank of New York Economic Policy Review, 8(1), pp 183–202.
Krause S and F Méndez (2005), ‘Institutions, arrangements, and preferences for inflation stability: evidence and lessons from a panel data analysis’, Emory University, Department of Economics Working Paper No 05-01.
Levin R (1997), ‘Financial development and economic growth: views and agenda’, Journal of Economic Literature, 35(2), pp 688–726.
McCarthy J and RW Peach (2002), ‘Monetary policy transmission to residential investment’, Federal Reserve Bank of New York Economic Policy Review, 8(1), pp 139–158.
McConnell MM, PC Mosser and G Perez-Quiros (1999), ‘A decomposition of the increased stability of GDP growth’, Federal Reserve Bank of New York Current Issues in Economics and Finance, 5(13), pp 1–6.
McConnell MM and G Perez-Quiros (2000), ‘Output fluctuations in the United States: what has changed since the early 1980s?’, American Economic Review, 90(5), pp 1464–1476.
Mishkin FS and K Schmidt-Hebbel (2002), ‘A decade of inflation targeting in the world: what do we know and what do we need to know?’, in N Loayza and R Soto (eds), Inflation targeting: design, performance, challenges, Central Bank of Chile, Santiago, pp 171–219.
Nelson CR and C-J Kim (1999), ‘Has the US economy become more stable? A Bayesian approach based on a Markov-switching model of the business cycle’, Review of Economics and Statistics, 81(4), pp 608–616.
Ramey G and VA Ramey (1995), ‘Cross-country evidence on the link between volatility and growth’, American Economic Review, 85(5), pp 1138–1151.
Ramey VA and DJ Vine (2004a), ‘Tracking the source of the decline in GDP volatility: an analysis of the automobile industry’, NBER Working Paper No 10384.
Ramey VA and DJ Vine (2004b), ‘Why do real and nominal inventory-sales ratios have different trends?’, Journal of Money, Credit, and Banking, 36(5), pp 959–963.
Smith PA and PM Summers (2002), ‘Regime switches in GDP growth and volatility: some international evidence and implications for modelling business cycles’, Melbourne Institute Working Paper Series No 21/02.
Stock JH and MW Watson (2002), ‘Has the business cycle changed and why?’, in M Gertler and K Rogoff (eds), NBER macroeconomics annual 2002, MIT Press, Cambridge, pp 159–218.