RDP 2023-06: Firms' Price-setting Behaviour: Insights from Earnings Calls 6. Firms' Price-setting Behaviour
September 2023
- Download the Paper 1.6MB
Understanding what influences firms' price-setting behaviour is important for understanding the dynamics of the inflation process. In this section, we use panel regressions to study how firms' sentiment about final prices is associated with changes in sentiment regarding various input costs and demand and how these responses vary by industry. We also explore if there are any differences depending on whether the sentiment of the discussions about input costs and demand is increasing or decreasing, which enables us to test for evidence of price-setting asymmetries. Our estimates only represent reduced-form statistical correlations and caution should be exercised in drawing a causal interpretation from them. Caution should also be adopted when generalising our results beyond the sample of large, listed companies that are the focus here. Previous research has documented notable differences in the price-setting behaviour of larger firms compared to their smaller counterparts.[12] The insights from this exercise are complementary to those obtained from central bank surveys of firms' pricing behaviour.[13] However, compared to central bank surveys, the use of earnings calls means we can examine price-setting behaviour for a panel of firms over a long history of time and that any insights can be updated at regular intervals.
We begin by estimating the following panel regression, with the data beginning in 2007:
Here pit denotes the final price sentiment index for firm i in time period t, measured at a quarterly frequency.[14] The term is the demand sentiment index over the period 2007–2020, while is the index from 2021. Likewise, icn,it is the input cost index for input cost sentiment index . Including separate coefficients for each time period allows us to examine if there is anything different about the period since 2021 – which has been characterised by sizable supply shocks – relative to the historical sample. The regression includes firm, , and time fixed effects, . The firm fixed effects allows us to control for unobservable differences in the language used by each firm during their earnings calls. The time fixed effects control for changes in the operating environment that are common to all firms, including the effect of global supply shocks.
In Equation (4) below, we instead include separate coefficients for demand and aggregate input costs according to whether the change in sentiment was positive or negative . This allows us to examine whether price-setting sentiment changes asymmetrically in response to positive or negative changes in input costs or demand sentiment.
Finally, in Equation (5) below, the demand and input cost variables are interacted with industry dummies, , enabling us to examine variation in price-setting behaviour by industry. We also include industry-time fixed effects, , to control for changes in the operating environment that are common to all firms in a particular industry.
In all regressions standard errors are clustered at the industry level to accommodate within-industry serial correlation. All regressions are estimated using the sentiment indices from the zero-shot text classifier constructed according to Equation (2) in Section 3, with separate results presented for the overall and future-tense versions of the indices.
Before turning to the results, a description of the data used to estimate our regressions is provided in Table 4 below. Notably, in both the pre- and post-COVID-19 periods, there is ample within-firm variation in each of the indices to use for identifying conditional correlations between firms' final price sentiment and our various other sentiment indices. As a complement to Table 4 we can also plot the distribution of the sentiment indices for prices, demand and aggregate input costs, focusing only on changes in sentiment within firms – that is, each firm's deviation from its own average over time. Figure 9 shows there is substantial variation about the mean in both periods, with some minor right-skewness in the post-COVID-19 period. Finally, Table D1 shows cross-correlations between each of the sentiment indices, using within-firm variation only. Correlations are typically small and positive, indicating that multicollinearity is unlikely to affect the interpretation of our coefficient estimates.
Mean | Overall standard deviation |
Within standard deviation(a) |
Min | Max | |
---|---|---|---|---|---|
Price07–20 | 0.6 | 3.1 | 2.6 | −28 | 29 |
Price21–23 | 1.4 | 2.6 | 2.4 | −11 | 22 |
Demand07–20 | 6.7 | 8.3 | 5.0 | −22 | 64 |
Demand21–23 | 7.3 | 8.1 | 4.4 | −10 | 55 |
Aggregate input costs07–20 | 1.6 | 4.8 | 3.9 | −29 | 37 |
Aggregate input costs21–23 | 3.7 | 6.7 | 4.6 | −12 | 72 |
Import costs07–20 | −0.2 | 1.5 | 1.3 | −9 | 10 |
Import costs21–23 | −0.1 | 1.5 | 1.2 | −11 | 8 |
Labour costs07–20 | 0.1 | 1.4 | 1.2 | −8 | 10 |
Labour costs21–23 | 0.7 | 2.1 | 1.6 | −6 | 17 |
Supply shortages07–20 | 0.9 | 1.7 | 1.2 | na | 20 |
Supply shortages21–23 | 1.7 | 3.1 | 2.1 | na | 40 |
Transport costs07–20 | 0.0 | 1.6 | 1.3 | −9 | 21 |
Transport costs21–23 | 0.4 | 1.9 | 1.4 | −24 | 34 |
Note: (a) Defined as the standard deviation of the series, , where x is the topic of interest. Sources: Authors' calculations; Reuters. |
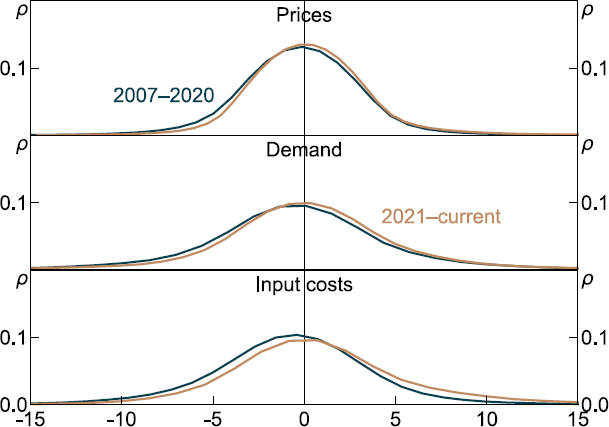
Sources: Authors' calculations; Reuters.
The results from estimating Equation (3) are shown in Table 5; results from estimating Equation (4) are shown in Table 6; and results from estimating Equation (5) are summarised in Figure 10. We have four key empirical findings.
First, sentiment about final prices has a significantly stronger association with sentiment about input costs compared to sentiment about demand (Table 5, column 1). The coefficients on demand and input costs shown in column 1 are statistically different at the 1 per cent level. Specifically, the results suggest a 10 percentage point increase in the share of paragraphs about demand increasing (on net) is associated with around a 1 percentage point increase in the share of paragraphs about final prices increasing (on net), whereas the same increase in references to input costs increasing (on net) is associated with a 2 percentage point increase in the share of paragraphs about final prices increasing (on net).[15] This result holds over the period 2007–2020 as well as in the post-COVID-19 period. The extra emphasis on costs is in line with messages the Reserve Bank of Australia receives from its periodic surveys of firms' pricing strategies, which indicates that the predominant pricing strategy of firms is to set prices as a mark-up on costs (Park et al 2010). It is also consistent with empirical work showing firms' expectations for their own costs are an important determinant of their price-setting behaviour – see, for example, Meyer, Parker and Sheng (2021) and Asghar, Fudurich and Voll (2023). This notwithstanding, when talking about the future, firms appear to place equal emphasis on the outlook for input costs and demand (Table 5, column 2).
Second, disaggregating input costs into selected subindices shows that the historically positive association between labour cost sentiment and final price sentiment has been weaker and statistically insignificant in the post-COVID-19 environment (Table 5, column 3). These two coefficient estimates are statistically different from each other at the 15 per cent level (t-test, p-value = 0.14). On the other hand, there has been a statistically significant increase in the association between import costs and final prices (t-test, p-value = 0.06).[16] Because we control for the impact of shocks that are common to all firms, this result cannot be attributed to the increase in global supply shocks that have occurred over the past few years. Instead, it provides tentative evidence that the pass-through of import costs to final prices may have risen recently, while the pass-through of labour costs may have fallen. This latter finding is consistent with Amiti et al (2022) for the United States, who use industry-level data to show that higher pass-through of import prices has made a significant contribution toward recent higher inflation, though they also point to higher pass-through of labour costs.
Aggregated input costs | Disaggregated input costs | ||
---|---|---|---|
All tenses | Forward-looking tense | All tenses | |
Demand07–20 | 0.085*** | 0.104*** | 0.092*** |
Demand21–23 | 0.080*** (−0.048) |
0.089*** (−0.015) |
0.084*** (−0.009) |
Aggregate input costs07–20 | 0.199*** | 0.106*** | |
Aggregate input costs21–23 | 0.162*** (−0.037) |
0.089** (−0.017) |
|
Import costs07–20 | 0.045 | ||
Import costs21–23 | 0.165** (+0.120*) |
||
Labour costs07–20 | 0.076* | ||
Labour costs21–23 | −0.003 (−0.079) |
||
Supply shortages07–20 | 0.094 | ||
Supply shortages21–23 | 0.117** (+0.023) |
||
Transport costs07–20 | 0.144* | ||
Transport costs21–23 | 0.138** (−0.006) |
||
Sample | 5,145 | 5,145 | 5,145 |
Within R2 | 0.174 | 0.087 | 0.122 |
Note: Standard errors are clustered at the industry level; ***, ** and * denote statistical significance at the 1, 5 and 10 per cent levels, respectively. |
Third, we uncover no pricing asymmetries with respect to whether demand sentiment has improved or deteriorated (Table 6, column 1). That is, the association with discussions about final prices is the same regardless of whether sentiment regarding demand conditions increased (indicated by in Table 6) or decreased (indicated by in Table 6). This finding appears in contrast to messages from firm-level surveys of firms' price-setting behaviour, which tend to find that falls in demand are more likely to prompt price changes – see, for example, Fabiania et al (2006). Moreover, when focusing on forward-looking indices, firms' price-setting is significantly more responsive to an improvement in demand sentiment compared to a deterioration in sentiment (t-test, p-value = 0.04) (Table 6, column 2).
By contrast, the association between input cost sentiment and final price sentiment is stronger when firms are talking about input costs increasing as opposed to decreasing (t-test, p-value = 0.06) (Table 6). This asymmetry could suggest that rising prices could remain front of mind for company executives even as input costs decline from their current high levels. Our findings in this regard are consistent with the narrative-based evidence presented in Pitschner (2020). Here the author uses archived corporate filings for a large sample of US firms to show that cost shocks only appear to matter for price increases, concluding that prices are likely to be stickier after cost decreases than they are after cost increases.
All tenses | Forward-looking tense | |
---|---|---|
0.096*** | 0.055*** | |
0.081*** (−0.015) |
0.107*** (+0.051**) |
|
0.152*** | 0.076*** | |
0.193*** (+0.040*) |
0.117*** (+0.041) |
|
Sample | 4,599 | 4,599 |
Within R2 | 0.183 | 0.097 |
Note: Standard errors are clustered at the industry level; ***, ** and * denote statistical significance at the 1, 5 and 10 per cent levels, respectively. |
Finally, the results suggest that pricing behaviour differs significantly across industries, after controlling for shocks to prices that are common to all firms within an industry (Figure 10). Cost-focused strategies appear more important for the consumer staples, utilities, materials and industrial industries. Demand-focused strategies also appear important for the materials industry and to a lesser extent the real estate, consumer discretionary, financial and health care industries. These findings appear consistent with the fundamental business properties of the respective industries. For instance, prices in the consumer staples industry, which includes supermarket chains and agricultural goods and services providers, are highly exposed to supply-side shocks, such as breaks in supply chains, but the price elasticity of demand for consumer staples is typically considered to be low. On the other hand, the price elasticity of demand in the consumer discretionary industry, which includes firms providing entertainment services, tourism services, consumer durables and speciality retail is typically considered to be higher. Prices in the materials industry, which includes Australia's largest miners, are also strongly influenced by global demand factors. Taking a step back, the substantial heterogeneity by industry in these estimates illustrates that the macroeconomic impact of aggregate shocks crucially depends on which industries are particularly affected by them, a conclusion also reached by Dias et al (2015).
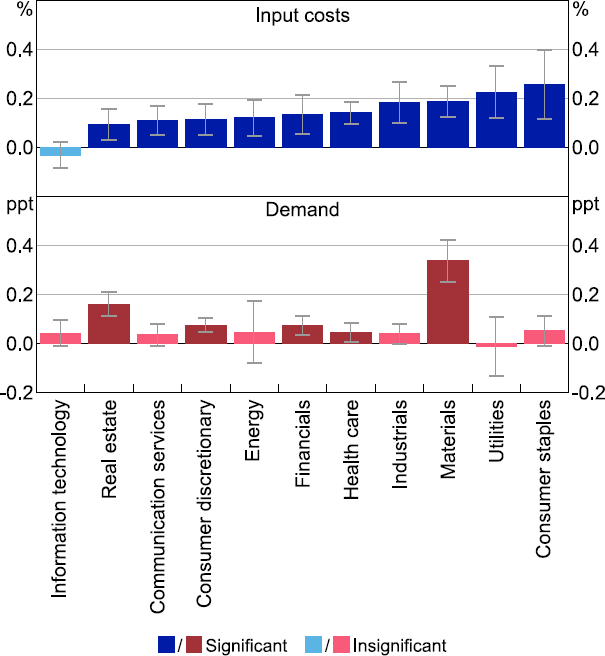
Note: Whiskers show 90 per cent confidence intervals.
Sources: Authors' calculations; Reuters.
Footnotes
For example, Amiti, Itskhoki and Konings (2019) find that small firms exhibit complete cost pass-through whereas the pass-through of larger firms is only around 50 per cent. [12]
For example, Bunn et al (2022) for a Bank of England survey; Fabiania et al (2006) for a European Central Bank survey; and Park et al (2010) for a survey by the Reserve Bank of Australia. [13]
The methodology used here is broadly similar to that used in Young et al (2021). [14]
The relativity in these impacts is similar if we standardise the variables and examine the association to a one standard deviation change. [15]
One potential explanation for the results about labour and import costs is that executives have limited speaking time and so only focus on some issues (e.g. import costs) at the expense of other issues (e.g. labour costs). If this was the case, there would be a negative within-firm correlation between references to our topics of interest. Instead, Table D2 shows that all of the correlations are positive. [16]