RDP 2021-11: Smells Like Animal Spirits: The Effect of Corporate Sentiment on Investment 3. Company-level Information on Sentiment and Investment
November 2021
- Download the Paper 1.7MB
3.1 Company-level sentiment
There are two general approaches for quantifying sentiment in text – the dictionary- (or lexicon-) and the machine learning-based approaches. The dictionary-based approach relies on predefined lists of words with each word either classified as positive, negative or neutral. The machine learning approach predicts sentiment of any given set of text after training models with a large set of text that has been assigned sentiment ratings by human readers. For example, models have been developed using social media data, such as Twitter, that provide text that is combined with user feedback to identify the sentiment of the posts. This approach is better able to capture the nuances in human language but it is more complex and less transparent (Huang and Simon 2021).
I follow the dictionary-based approach to construct company-level indicators of sentiment and uncertainty. The method is simple and intuitive and has been demonstrated to be sufficient to be able to predict equity returns at the company level. The sentiment indicator measures the net balance of words used in company reports that are considered to be ‘positive’ and ‘negative’. When companies use more positive words and/or fewer negative words, this is an indicator that sentiment at the company has increased. The uncertainty indicator measures the share of uncertain words used (e.g. ‘uncertain’ and ‘risk’).
I rely on the Loughran and McDonald (2011) (hereafter LM) dictionary of predefined positive, negative and uncertain words that is specially designed for economics and finance. The Harvard Dictionary produces many different word lists, including positive words, negative words, and uncertain words. But nearly three-quarters of the words identified as ‘negative’ by the Harvard Dictionary are words that are not considered negative in business terminology. For example, the words ‘taxes’ and ‘debt’ are not necessarily negative in the context of economics. Similarly, the word ‘credit’ is not necessarily a positive word.
LM develop alternative word lists that better reflect tone in business terminology. For example, they identify close to 2,500 negative words. Some of the most common negative words that appear in company reports include ‘loss’, ‘failure’, ‘default’, ‘termination’ and ‘adverse’. They then examine how such word lists are linked to company-level factors such as stock trading volume, unexpected earnings and fraud.
For the sample, PDF versions of company annual reports are hand collected from the Connect 4 website and converted to text files. The LM dictionary is applied to these text files to create the sample. Common steps in the natural language processing literature are taken to clean the raw dataset before analysis: numbers, punctuation marks, white spaces and common stop words are removed from each article. All words are then reduced to their respective ‘stem’, which is the part of a word that is common to all of its inflections (for example, ‘performs’, ‘performing’, and ‘performed’ are reduced to ‘perform’).
The word clouds in Figure 3 indicate that the most common positive words in Australian corporate disclosures are terms such as ‘benefit’, ‘good’ and ‘success’. The most common negative words include terms such as ‘loss’, ‘defer’ and ‘impair’. These word clouds do not appear to have changed much over time, as shown by a comparison of the 2005 and 2020 clouds.
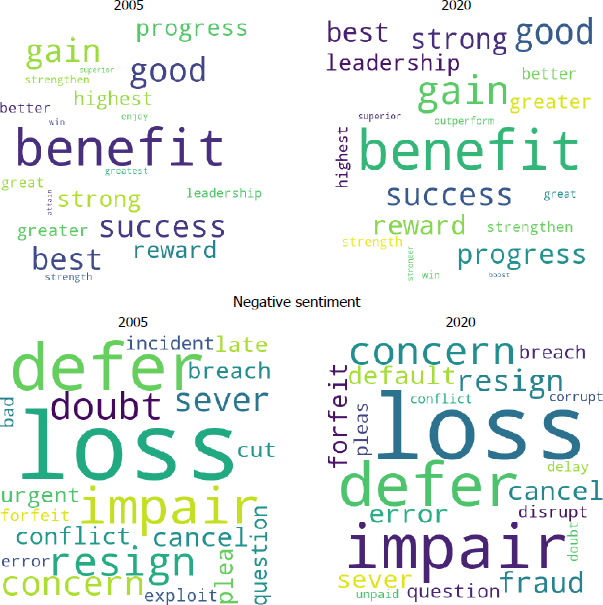
Note: The size of each word reflects its relative frequency
Sources: Author's calculations; Connect 4
However, the word clouds mask some apparent changes in the underlying language used in corporate disclosures. For instance, the net balance of positive words shows a clear downward trend over time based on the raw (unadjusted) data (top panel of Figure 4). This is caused by companies increasingly using words with a negative connotation (bottom panel of Figure 4). This may be due to a gradual shift in corporate disclosures towards ‘investor-friendly’ documents that are more transparent and include more discussion of risks and uncertainties to the outlook.
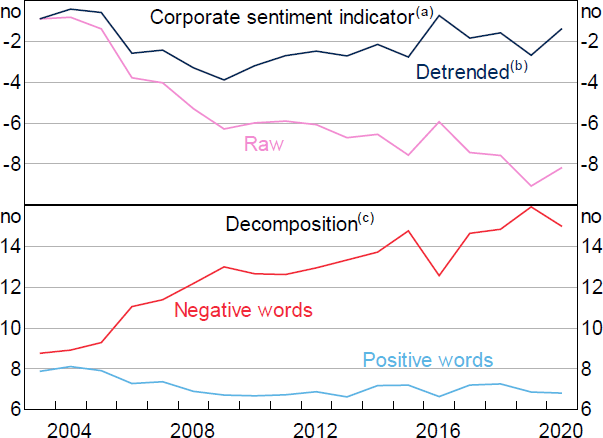
Notes:
(a) Net balance of positive and negative words per 10,000 words
(b) Residuals from OLS regression of corporate sentiment on company dummies and a linear trend
(c) Based on unadjusted sentiment data
Sources: Author's calculations; Connect 4
If the increasing propensity to use negative language is due to a shift towards more risk-based reporting, rather than growing concerns about the outlook, then this will make it more difficult to identify the true effect of corporate sentiment on investment. I adjust for the apparent trend by estimating an OLS regression of the sentiment indicator on a company fixed effect and a linear trend, and take the residuals. These residual estimates are referred to as the ‘adjusted’ corporate sentiment indicator, and will be used for the remainder of the analysis in the paper. However, the key results in the paper hold even when using the unadjusted measure of sentiment.[4]
3.2 Company-level investment and fundamentals
Corporate investment is measured as the log change in the net capital stock as reported on a company's balance sheet, which is equivalent to the net investment rate :
Here, the capital stock captures tangible assets such as property, plant & equipment and is measured on a net basis by deducting accumulated depreciation from the gross capital stock. I choose to use the capital stock measure because it is generally more commonly reported in Australian company reports than the gross capital expenditure measure. (The key results hold though using the gross capital spending measure.)
To proxy for corporate fundamentals I construct an estimate of Tobin's Q using company-level information on share prices and the number of outstanding shares. Tobin's Q is measured as:
where Q is the ratio of total market value of equity (E) plus the book value of liabilities (L) less inventories (Inv) divided by the book value of total assets (A).
There is extensive evidence that Tobin's Q is a poor guide to future corporate performance (e.g. Erickson and Whited 2000). So, in the empirical specification, I also consider a range of other firm-level indicators that could proxy for fundamentals, such as annual growth in sales (or turnover), the return on assets (measured as the ratio of earnings before interest and tax to total assets) and equity analysts' profit forecasts for the year ahead. I also explore the role of measurement error in Tobin's Q in a robustness test.
3.3 Publicly listed company sample
The sentiment indicator requires information on corporate disclosures, which means that the analysis is restricted to Australian publicly listed companies. The sample is an unbalanced panel of listed companies, with much of the data sourced from Morningstar. Share prices and equity analyst forecasts are sourced from Refinitiv Eikon. Before estimation, outliers are removed based on the top and bottom 1 per cent of the distribution of each of the investment rate, the sentiment indicator, the uncertainty indicator and the Q ratio. The sample covers close to 2,000 companies per year between 2003 and 2020.
Publicly listed companies account for about 1 in 2,000 companies and are typically older and larger than the average (unlisted) company. However, it is hard to be definitive about whether this sample leads to biased estimates of the effect of sentiment on investment. The regression sample appears to be broadly representative of the universe of listed companies. I find that there are no statistically significant differences between firm–year observations that are in the regression sample and those that are not in the sample on most measures, including investment and sentiment. The only exception is that firms in the regression sample are a bit larger (based on the book value of assets) and have a slightly lower Q ratio than those that are not in the regression sample.
3.4 Information sets of company managers and investors
To empirically identify the effect of sentiment on investment it is important to consider what is in the information sets of the managers and investors at the time of the release of the company annual reports, which are used to measure sentiment.
As an example, consider a hypothetical company that releases its annual report for the financial year 2019/20 in August 2020. This report includes balance sheet information on the book value of the capital stock at the start (1 July 2019) and end of the financial year (30 June 2020), and therefore the flow of investment during 2019/20. The managers and investors can observe the share price on a daily basis at any time during 2019/20.
To capture the determinants of company investment decisions, I conservatively assume the relevant measure of Tobin's Q is based on the market and book values of the capital stock at the start of the financial year (1 July 2019), as these values are definitely observed by the managers at the time of any investment decision. I therefore restrict investor knowledge of the company, as captured in Tobin's Q, to the start of the period.
The end-of-year financial report is typically released a couple of months after the relevant financial year. This means that the language used in the report could reflect the knowledge of managers about investment-relevant events that occurred during and even after the reported financial year. This gives an information advantage to company managers, which may mean that the sentiment indicator is not a pure measure of animal spirits but is also capturing ‘insider’ knowledge of events that are relevant to investment, but which are not observed by the market at the start of the period.
To address this, I also consider an alternative version of the model in which Tobin's Q is measured at the end of June 2020 and sentiment is measured based on the language in the 2018/19 financial report. I report on these alternative estimates in a robustness test.
3.5 Summary statistics
Some summary statistics are shown in Table 1. Investment is clearly skewed across the corporate population with the average company investing about 10 per cent of its capital stock each year, while the median company invests closer to 3.3 per cent, with a high standard deviation. The average company also has a relatively high Q ratio, which appears to be mostly due to high valuations for mining companies during the mining boom period. This is despite the fact that the average company makes losses, as shown by the negative return on assets. The average company uses 7.2 positive words, 13.3 negative words and 5.7 uncertain words for every 10,000 words in its annual reports.
Mean | Median | Standard deviation | 25th percentile | 75th percentile | |
---|---|---|---|---|---|
Investment rate (%) | 10.3 | 3.3 | 84.1 | −20.5 | 31.9 |
Positive sentiment (per 10,000 words) | 7.2 | 7.0 | 1.9 | 6.0 | 8.2 |
Negative sentiment (per 10,000 words) | 13.3 | 13.2 | 3.5 | 11.1 | 15.4 |
Uncertainty (per 10,000 words) | 5.7 | 5.7 | 1.9 | 4.5 | 6.9 |
Q ratio (times) | 2.2 | 1.4 | 2.3 | 0.9 | 2.5 |
Return on assets (%) | −15.3 | −3.7 | 87.7 | −23.3 | 12.9 |
Sales growth (%) | 10.0 | 7.2 | 110.2 | −21.5 | 38.6 |
Note: Sample statistics based on estimation sample for the baseline fixed effects regression Sources: Author's calculations; Connect 4; Morningstar; Refinitiv Eikon |
Footnote
Note that the net balance measure is normalised by the number of words per company report, and the trend over time in the net balance is not due to a decrease in the length of corporate disclosures (in fact, there has been an increase in average word count over time). The fact that the LM dictionary includes more negative words than positive words can explain why companies use more negative terms on average. However, the relative length of the word lists should be less of an issue for changes over time in sentiment, which is the main focus of the analysis in this paper. [4]