Research Discussion Paper – RDP 2016-02 Disagreement about Inflation Expectations
April 2016
1. Introduction
Inflation expectations play a central role in modern monetary economics. Reflecting the importance of inflation expectations to monetary policymaking, there is a large literature studying their behaviour. Much of the literature has focused on understanding how expectations are formed and adjust: Are expectations rational? How do expectations adjust in response to changes in monetary policy regimes? However, there is comparatively little work studying the behaviour of disagreement about inflation expectations.[1] In part, this reflects the absence of a role for different beliefs in most macroeconomic models.
Understanding the behaviour of disagreement is of interest to a central bank for a few reasons. First, the welfare costs of inflation are often thought to arise not from the level of inflation per se, but rather uncertainty about future inflation (Friedman 1977). An important justification for targeting a low level of inflation is that it is more likely to be associated with more predictable inflation (Ball (1992) provides a theoretical model linking the level of inflation to inflation uncertainty). More dispersed expectations are likely to be indicative of larger average absolute forecast errors, and so higher costs from inflation uncertainty.
Second, less disagreement might indicate better anchoring of expectations. In particular, disagreement might reflect different beliefs about trend inflation, possibly arising from different perceptions of the credibility of the central bank's inflation target. Previous research has found that inflation expectations tend to be less dispersed in economies that have inflation-targeting central banks, consistent with there being a link between disagreement and inflation credibility (Capistrán and Ramos-Francia 2010).
Third, the behaviour of disagreement can help discriminate between different models of expectations formation. In particular, dispersed expectations can result from costly, and therefore infrequent, processing of information among agents, or uncertainty about the structure of the economy. Models incorporating disagreement can explain some empirical regularities that representative agent models cannot. For example, models featuring disagreement can explain the delayed response of inflation and output to monetary policy shocks, whereas the benchmark New-Keynesian Phillips curve, which features no disagreement among agents, cannot (Mankiw and Reis 2002; Woodford 2003; Mackowiak and Wiederholt 2009). This makes it important to document the extent of disagreement and to understand its behaviour.
This paper examines key stylised facts about disagreement surrounding inflation expectations in Australia. In doing so, we employ several novel datasets. In particular, we use individual response data from five different surveys: two of market economists, one of union representatives, one of consumers, and a survey by The Age newspaper that is a mix of mostly professional forecasters from financial markets, unions and academia. For the consumer measure, we use a new method to identify the underlying distribution, separate from noise introduced by infeasible and clustered responses. We do not have survey data on expectations for managers involved in pricing decisions, but Kumar et al (2015) provide evidence from New Zealand that expectations of managers closely resemble those of consumers.
From an inflation forecasting perspective, the expectations of professional forecasters such as market economists might be most relevant, because professionals put significant resources into understanding the likely trajectory of inflation. But consumer and union measures may provide information on the expectations embedded in wage negotiations, and thereby influence the trend rate of inflation.
The empirical analysis addresses two key questions. First, we examine whether historical disagreement provides some clues as to how agents form their expectations. There are several prominent models of expectations formation that help us understand how disagreement in expectations can arise. Under the benchmark assumption used in most macroeconomic models – full information and rational expectations – there is no disagreement. However, disagreement can occur in models relaxing the assumption of full information. The sticky-information model proposed by Mankiw and Reis (2002) assumes only a fraction of agents are attentive each period and so form updated expectations; disagreement occurs because agents update their expectations at different points in time. Noisy-information models assume agents observe fundamentals with errors, so generate disagreement because individuals observe different signals. Disagreement may also arise because of persistent differences in beliefs about the mean level of inflation. We interpret the behaviour of disagreement in light of these models, making inference about how expectations are formed.
In all, the behaviour of disagreement over time does not fit neatly within any particular model, although we do find evidence consistent with information rigidities. The extent of disagreement appears to respond little to most macroeconomic news surprises, although we do find some evidence that disagreement rises in response to surprise changes in GDP growth, and when current inflation deviates from the middle of the Reserve Bank of Australia's (RBA) 2–3 per cent target. There have been several episodes since the introduction of inflation targeting in which disagreement has risen noticeably among all groups of agents.
Second, we consider disagreement as an indicator of the anchoring of inflation expectations in Australia, both across time and agents. Provided inflation expectations are close to the target level on average, a lower level of disagreement indicates more firmly anchored inflation expectations. We document an approximate halving in disagreement about inflation expectations among respondents to The Age's economic survey since the adoption of inflation targeting. We also find evidence of a decline in disagreement for both surveys of market economists, by about half relative to periods in the mid to late 1990s.
Consumers' inflation expectations generally appear much less anchored than those of professionals. The magnitude of disagreement among consumers is substantial – about five times that of professionals. A large majority of consumers expect inflation to lie outside of the RBA's target over time, with a sizeable fraction expecting inflation above 10 per cent. Unlike professionals, there is no evidence of a decline in disagreement since the introduction of inflation targeting. However, disagreement for consumer inflation expectations is estimated to co-move with the expected level of inflation, implying that disagreement among consumers may have declined since the 1980s when consumer inflation expectations were substantially higher; unfortunately unit record data before 1995 are unavailable to test this implication. Finally, there are persistent differences in inflation expectations according to individuals' demographic characteristics, which indicate different perceptions of long-run inflation.
Part of the disagreement in consumer expectations appears to be due to a large share of uninformed (or misinformed) responses. Consistent with this, a notable feature of consumer inflation expectations is their sensitivity to petrol prices, which provides evidence of non-rationality. This also provides evidence against the sticky-information model of expectations, which assumes that the frequency of updating is time- rather than price-dependent.
The survey question, which asks consumers about changes in the prices of the things they buy, is another contributor to disagreement about consumer inflation expectations. Because price changes differ across goods, individual inflation experience depends on differences in spending patterns across consumers. However, we find that differences in individual inflation experience can account for only a small fraction of the disagreement about consumer inflation expectations.
2. Why Does Disagreement Arise?
Before investigating the behaviour of disagreement in inflation expectations, we briefly summarise prominent models of expectations formation. This helps us understand how disagreement about expectations can arise, and generates predictions about its behaviour. Furthermore, because theoretical models make different predictions about the behaviour of forecast disagreement, we can use the observed behaviour of forecast disagreement to draw implications about the expectations formation process. We focus on four prominent models of expectations formation.
Full information rational expectations
The full information rational expectations (FIRE) model assumes that agents efficiently process all available information; forecasts are identical across agents, and thus there is no disagreement about expectations. Because the FIRE model incorporates no frictions, it is a stark description of reality. But the FIRE assumption underpins a wide range of macroeconomic models, and so provides a benchmark from which to gauge the predictions of richer models.
Sticky information
The sticky-information model assumes that information acquisition and processing requires costly effort (Reis 2006). Accordingly, agents only periodically update their forecasts. The sticky-information model retains the assumption of rational expectations; that is, agents are assumed to form forecasts consistent with rational expectations when updating their beliefs. Disagreement rises in response to a macroeconomic news surprise because at any point in time only a fraction of agents update their forecasts to incorporate the news (the models of Mankiw and Reis (2002) and Reis (2006) assume updating occurs at random intervals). Disagreement will tend to decay over time as the fraction of agents incorporating the news into their expectations increases, but providing the frequency of macroeconomic news surprises is high relative to the speed at which agents update their expectations (as is the case in most parameterisations of the model), there will be disagreement among agents at all times.
At a conceptual level, the sticky-information model provides a plausible description of consumer behaviour. Interpreted literally, the assumption that agents only periodically update their expectations is inconsistent with the behaviour of professional forecasters but, interpreting the information friction more broadly to reflect periodic or infrequent changes in model parameters and structural breaks, the sticky-information framework may nonetheless apply to professional forecasters.
Noisy information
Noisy-information models assume that agents filter noisy data to infer the true state of the economy (Lucas 1972; Sims 2003; Woodford 2003). In contrast to the sticky-information model, agents are assumed to update their forecasts continuously. A striking prediction of the baseline noisy-information model is that disagreement will not respond to macroeconomic news (Coibion and Gorodnichenko 2012). Although individual agents face signals with different idiosyncratic errors at any point in time, the precision of signals in the baseline noisy-information model is assumed to be uncorrelated with macroeconomic news shocks and the same across agents, in which case disagreement is constant over the business cycle. Coibion and Gorodnichenko (2012) show that the absence of a response of disagreement to macroeconomic news extends to richer noisy-information models featuring strategic interaction among forecasters (e.g. forecasters may not want to deviate too far from the average). But they show that the noisy-information model can generate an increase in disagreement in response to macroeconomic news if the precision of signals differs across agents.
Disagreement about means
Disagreement among agents may arise because of differences in beliefs about long-run means (Patton and Timmerman 2010). For example, agents may have different priors on the inflation credibility of a central bank, and so form different views about the expected level of inflation at short and long horizons. Because this type of disagreement does not reflect different information sets available to forecasters, disagreement does not respond to macroeconomic news surprises (Coibion and Gorodnichenko 2012).
This discussion is summarised in Table 1 below.
Full information rational expectations | Sticky information | Noisy information(a) | Disagreement about means | |
---|---|---|---|---|
Scope for disagreement | No | Yes | Yes | Yes |
Response of disagreement to news | Increases | No | No | |
Long-run disagreement | No | No | Yes | |
Note: (a) Noisy-information models featuring heterogeneous precision of signals across agents predict an increase in disagreement in response to macroeconomic news (see Coibion and Gorodnichenko (2012)) |
3. Data Sources
We use data from five separate surveys of inflation expectations, comprising different types of agents: the Consensus Economics survey and RBA survey, both of market economists; the Sydney University Workplace Research Centre survey of trade union officials (union survey); the Melbourne Institute Survey of Consumer Inflationary Expectations (consumer survey); and The Age Economic Survey, which comprises a mix of market economists, unions, and academics.
While the Melbourne Institute survey measure asks consumers about the ‘prices of things you buy’, the other measures are based on surveys of expectations of headline CPI inflation. The union survey is an exception, in which respondents were principally asked about their expectations for headline inflation, although at some points in time expectations for underlying inflation were recorded. The surveys also differ in their frequency, sample periods, and sample size, as reported in Table 2.
Respondent type | Survey organisation | Beginning of sample period | Frequency | Number of respondents | Surveyed inflation measure |
---|---|---|---|---|---|
Market economists | Consensus Economics | 1990 | Monthly | 15–20 | Headline CPI |
Market economists | RBA | 1993 | Quarterly | 10–20 | Headline CPI |
Unions | Sydney University Workplace Research Centre | 1996 | Quarterly | 15–20 | Mix of headline and underlying CPI |
Consumers | Melbourne Institute of Applied Economic and Social Research | 1995 | Monthly | 1,000–1,300 | Change in ‘prices of things you buy’ |
Mixed | The Age | 1979 | Semi-annual | 18–42 | Headline CPI |
Notes: Unit record data for the Melbourne Institute survey are unavailable prior to 1995; The Age survey is conducted semi-annually, but we use data only from the end-of-year survey |
There are some limitations to the data. Ideally, we would examine the behaviour of disagreement at a range of horizons, with long-horizon disagreement potentially most informative about the degree of anchoring of inflation expectations. However, disaggregated survey data on long-horizon expectations are largely unavailable for Australia. A key exception is the union survey, for which inflation expectations are available at short- and medium-term horizons, corresponding to year-ahead inflation and inflation over the next 5 to 10 years.[2] Notably, the level of disagreement is similar and co-moves for both horizons (discussed in Section 4.1). This gives us greater confidence in the relevance of our findings regarding the anchoring of inflation expectations.
Although we make use of individual response data to measure disagreement about inflation expectations, our data form repeated cross-sections rather than panel data. While we would ideally make use of panel data to isolate persistent versus transitory differences in expectations between survey respondents, this is not possible for most survey measures. The consumer survey measure comprises a random sample of consumers each month, while the Consensus Economics, RBA, and union surveys record the survey participant's employer rather than the name of the survey respondent; turnover of respondents within surveyed organisations means we cannot track individuals through time. An exception is The Age survey, which does record the names of surveyed economists. Some individuals have participated in The Age survey for an extended period of time, but many have not. Because relatively few individuals can be tracked for an extended period of time, we do not make use of the panel aspect of these data.
Except for the Consensus Economics survey, respondents are asked to report inflation expectations for the year ahead. The Consensus Economics survey asks for inflation over calendar years, including the current year, and so the monthly series will include forecasts at horizons of between 6 and 17 months. Thus, disagreement about inflation expectations is mechanically lower in later months of each year because there are fewer unknown inflation outcomes. In our regressions, we include time dummy variables to remove this predictable variation in disagreement.
4. Descriptive Analysis
4.1 Professional and Union Surveys
Figures 1 and 2 plot the mean, range, and standard deviation of responses for each survey measure of inflation expectations, except for the consumer measure. The consumer measure has several unique features and is discussed in the next section. We present two measures of disagreement: the range of responses (highest minus lowest response in each survey), and the standard deviation of responses. We prefer the standard deviation measure because it is more robust to a single extreme survey response than the range, but in general the information conveyed by each measure is qualitatively similar.
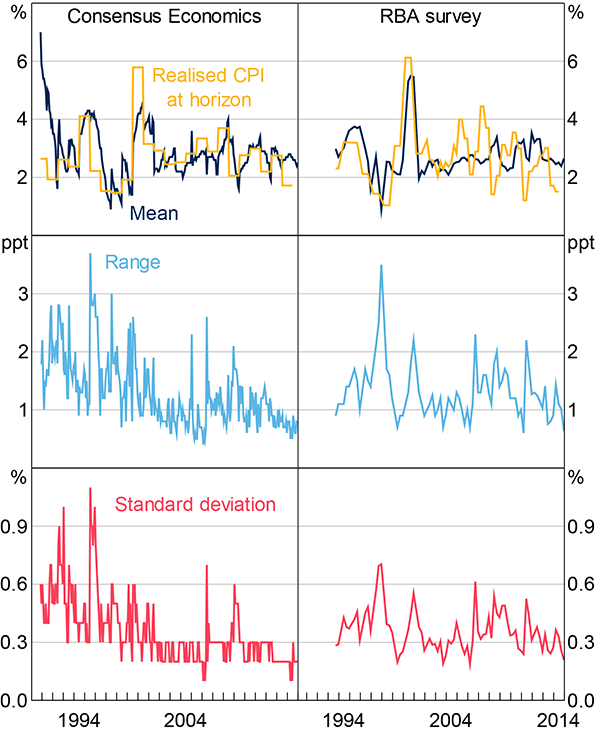
Note: The standard deviation of responses to the Consensus Economics survey is reported to one decimal place
Sources: ABS; Authors' calculations; Consensus Economics; RBA
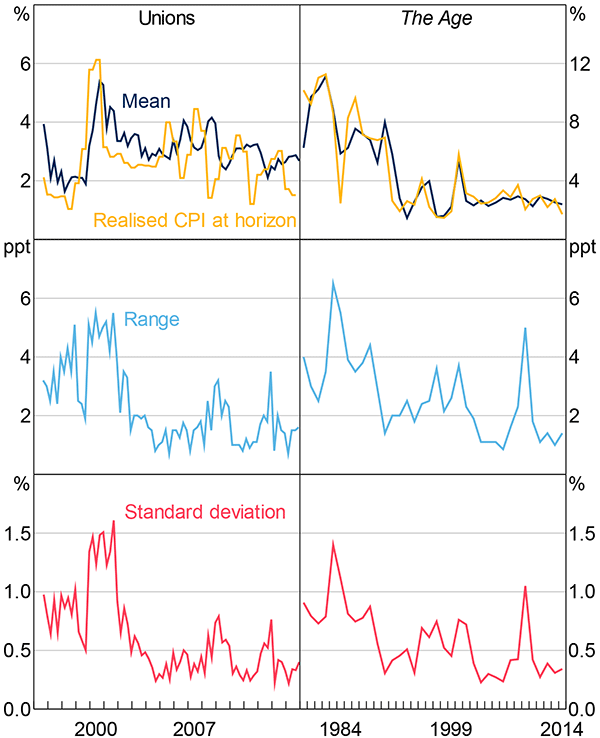
Note: End-of-year survey data from The Age reported
Sources: ABS; The Age; Authors' calculations; Workplace Research Centre
The first notable feature of the data is a decline in the level of disagreement for each of the surveys during the 1990s, which supports the idea that inflation expectations have become better anchored. A reduction in disagreement is most evident in The Age survey, the only measure for which individual response data is available for the 1980s. The standard deviation of responses to The Age survey averaged around 0.9 per cent over the 1980s, compared to 0.4 per cent over the past decade. Some decline in the average level of disagreement is also apparent for the Consensus Economics measure, with the standard deviation of responses averaging around 0.6 per cent in the early 1990s – when inflation targeting was in its infancy – and around 0.2–0.3 per cent more recently.
Second, there is clear time variation in disagreement for each of the survey measures. This variation is also somewhat common to the different measures; the degree of co-movement in disagreement between the series is reported in Table 3, which reports correlations for the quarterly Consensus Economics (end-of-quarter monthly survey), RBA and union survey measures (The Age survey is not available on a quarterly basis). The presence of co-movement suggests that the variation in disagreement through time is not just noise, but is economically meaningful.[3] In terms of the models discussed in Section 2, the mere existence of disagreement is inconsistent with the FIRE model, and time variation in disagreement is superficially inconsistent with the baseline noisy-information model, which does not generate variation in disagreement absent changes in the signal-to-noise ratio of economic data available to forecasters.
Consensus Economics | RBA survey | Unions: year-ahead | Unions: medium-term | |
---|---|---|---|---|
Consensus Economics | 1 | |||
RBA survey | 0.39 | 1 | ||
Unions: year-ahead | 0.37 | 0.31 | 1 | |
Unions: medium-term | 0.37 | 0.20 | 0.81 | 1 |
Notes: Contemporaneous correlations using standard deviations of year-ahead inflation forecasts for the period 1996–2014; we do not report a correlation for The Age survey measure here because it is not available quarterly |
Third, co-movement of disagreement is also very strong between the short- and medium-term measures of inflation expectations in the union survey. If expectations are rational and well-anchored, transitory deviations in short-term expectations should have little effect on medium- and long-term expectations, resulting in a low correlation between the measures. In contrast, the strong correlation between disagreement in short- and medium-term expectations provides some evidence against well-anchored inflation expectations. In addition, there is a strong correlation between short- and medium-term expectations at the individual union level, indicating that when short-term expectations are revised there is a flow-on effect to medium-term expectations. This suggests that it is reasonable to make inference about the anchoring of inflation expectations from a dataset principally limited to short-term measures. Interestingly, disagreement among union officials has almost always been higher for medium-term than short-term inflation expectations (Figure 3).
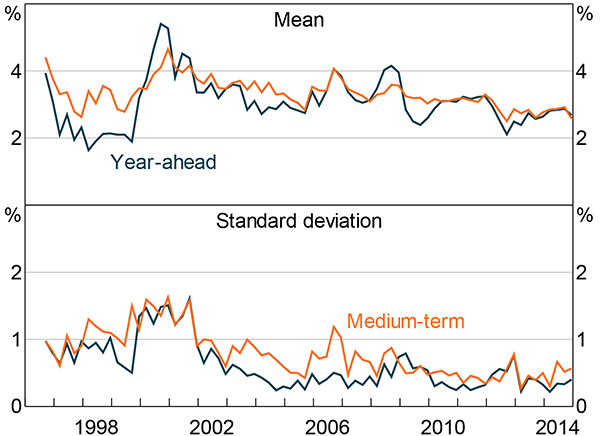
Sources: Authors' calculations; Workplace Research Centre
As discussed in the introduction, one justification for relatively low inflation targets is that low levels of inflation are likely to be associated with low levels of uncertainty about inflation. Providing some support for this argument, there is tentative graphical evidence of a relationship between mean inflation expectations and disagreement (which is formally tested in Section 5.2). In the mid 1990s, and in the period leading up to the global financial crisis, realised and expected inflation was at an elevated level and so was disagreement. Co-movement between disagreement and inflation expectations is consistent with the sticky-information model, because only a fraction of agents revise their forecasts each period, and periods of elevated inflation are likely to be periods in which those updating their forecasts make relatively large changes.
The introduction of the goods and services tax (GST) in July 2000 is an interesting episode in light of the sticky-information model. For the union survey measure, there was a pronounced rise in disagreement when the GST was introduced. Despite respondents being explicitly asked whether their expectations incorporated the effects of the GST, several respondents did not incorporate the policy change into their expectations during this period, clearly showing inattentiveness. In addition, the range of estimates of the size of the ‘GST effect’ spanned around 5 percentage points, indicating genuine disagreement. For the other survey measures, there is no notable spike in disagreement around the time the GST was introduced, indicating that respondents to those surveys were attentive to the effect of the GST on CPI inflation and in broad agreement about its effect on the price level.
4.2 Consumer Survey
4.2.1 Raw data
As mentioned earlier, the distribution of consumer inflation expectations exhibits characteristics that show a marked departure from those of professional forecasters. First, the distribution of consumer inflation expectations contains many more extreme values than the distribution of CPI inflation outcomes suggests is plausible. Responses of 50 and 100 per cent are given in most months the survey is conducted; well above the maximum observed CPI outcome of around 6 per cent since inflation targeting was introduced in 1993.[4] Thus, the distribution of consumer inflation expectations includes extreme values that cannot be explained by informed processing of available information. Extreme positive responses are more common than extreme negative responses, giving the distribution a positive skew. Second, and unlike for the professional survey measures, bunching of survey responses occurs at multiples of five. These two features of the data can be seen in Figure 4, which shows a histogram of responses to the consumer survey, pooled over the 1995–2014 period.
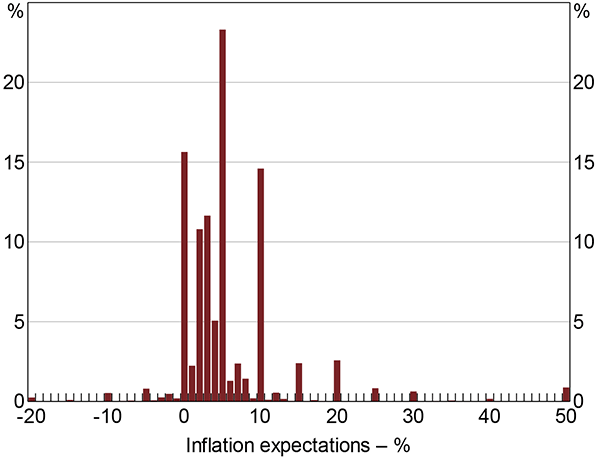
Note: Responses beyond axis limits attributed to bins at −20 and 50 per cent
Sources: Authors' calculations; Melbourne Institute of Applied Economic and Social Research
Measures of disagreement such as the standard deviation are sensitive to extreme observations. At the same time, extreme responses are likely to have little information content about ‘true’ community views, and will instead reflect other factors (Ranyard et al 2008; Bruine de Bruin et al 2010b).[5] For instance, it is possible that some respondents do not fully understand the concept of a percentage change or are not well informed about inflation developments. This could lead to responses that are selected at random, possibly at key round numbers, resulting in more instances of extreme observations.
Clustering at round numbers makes the distribution appear more discrete than continuous, with some large jumps in expectations between observations. However, assuming some respondents recognise their imprecision and thus round their expectations, these discrete responses can be thought of as representing a range of expectations centred on the particular number rather than a precise expectation. We would ideally like to observe a distribution that gives similar weight to observations around the cluster as the raw data, but that smooths out the distribution in between clusters.
In summary, some observations reflect accurate reporting of individuals' expectations of inflation, some are a discrete rounding of these expectations, while others are likely to represent noise with little information content. In order to ensure our analysis is robust, we use a structural framework to filter out noise and address bunching at round numbers.
4.2.2 Filtering the consumer inflation expectations data
Our approach imposes a categorical structure on the raw data, and then uses an ordered probit model to fit a normal distribution to the data. That estimated normal distribution provides the mean and standard deviation of inflation expectations, used in subsequent sections of the paper. The approach taken resembles that used by Bayer and Juessen (2015), who undertake a two-step analysis of ordinal data on self-reported wellbeing.
We believe this parametric approach is superior to the use of simple robust statistics, such as the median and interquartile range, as measures of the central tendency and disagreement in inflation expectations. While both the median and interquartile range measures impose less structure than our method, and are robust to extreme observations, they do not deal well with the clustering of expectations at round numbers.[6]
The disproportionately large share of responses equal to a multiple of five indicates that these observations likely reflect ‘rounded’ expectations. We allow for this by assuming that observations equal to a multiple of five reflect latent expectations in a small range above and below each multiple of five. For example, we assume the mass of reported inflation expectations at 5 per cent represents latent signals of future inflation over the interval 2.5 per cent to 7.5 per cent.[7] In contrast, there is little evidence of bunching at other points in the distribution, and we consider reported inflation expectations not equal to a multiple of five as ‘precise’.
The categorical structure we impose on the data before fitting an ordered probit model takes account of the distinction between rounded and precise observations. Because there are few observations not equal to a multiple of five outside the 0 to 10 range, we collapse all observations outside this range to the nearest multiple of five, limiting the number of categories for parsimony; we also fit only one category in the 6 to 9 range, centred at 7.5, owing to the few precise observations within this range. Lastly, we collapse observations within the range 32.5 to 100 (the maximum recorded value) and −12.5 to −50 (the minimum recorded value) into single bins.[8]
Table 4 reports the full categorical structure imposed on the raw inflation expectations data. The first column j reports the midpoint of each category (with the exception of the first and last category); the second column indicates whether the category represents a precise or rounded observation; the third column indicates the range over which latent signals for category j are assumed to be drawn; and the final two columns report the number and share of observations in each category for the period 1995–2014.[9] Note that the range for the rounded observations overlap the range for the precise observations within the 0 to 10 range.
Midpoint: j | Type | Latent range | Number of observations | Share of observations |
---|---|---|---|---|
−15 | Rounded | −50 (min):−12.5 | 731 | 0.3 |
−10 | Rounded | −12.5:−7.5 | 1,470 | 0.6 |
−5 | Rounded | −7.5:−2.5 | 2,964 | 1.1 |
0 | Rounded | −2.5:2.5 | 41,352 | 15.7 |
1 | Precise | 0.5:1.5 | 5,463 | 2.1 |
2 | Precise | 1.5:2.5 | 26,881 | 10.2 |
3 | Precise | 2.5:3.5 | 30,888 | 11.8 |
4 | Precise | 3.5:4.5 | 14,085 | 5.4 |
5 | Rounded | 2.5:7.5 | 61,245 | 23.3 |
7.5 | Precise | 5.5:9.5 | 15,156 | 5.8 |
10 | Rounded | 7.5:12.5 | 41,087 | 15.6 |
15 | Rounded | 12.5:17.5 | 7,321 | 2.8 |
20 | Rounded | 17.5:22.5 | 7,092 | 2.7 |
25 | Rounded | 22.5:27.5 | 2,299 | 0.9 |
30 | Rounded | 27.5:32.5 | 1,674 | 0.6 |
35 | Rounded | 32.5:100 (max) | 3,213 | 1.2 |
The categorical structure just described allows for the bunching of observations at round numbers, but does not by itself adequately reduce the influence of extreme responses. To allow for extreme responses, we augment the ordered probit model with a uniform distribution from which noisy observations are assumed to be drawn. We estimate the relative weight on the ‘noise’ distribution and the latent underlying normal distribution from which ‘true’ expectations are assumed to be drawn.
Formally, our method proceeds as follows. First, we assign the raw data to the categorical bins
listed in Table 4; we use the notation to refer to the
categorised inflation expectation of observation i. Second, we specify the
distributions from which ‘true’ and ‘noisy’ observations are assumed to
be drawn: ‘true’ observations are drawn from a normal distribution with mean
μ and standard deviation σ, and ‘noisy’ observations are
uniformly distributed over the range −50 to 100. The parameter ρ, which we
estimate, represents the proportion of true observations in each category. These assumptions are
summarised by Equations (1)–(3):
Third, we estimate the parameters ρ, μ, and σ by numerically maximising the log-likelihood function for cross-sections of reported inflation expectations.[10] Conditional on these parameters, the likelihood that a response to the inflation expectations survey lies within category j is given by:
where Φ is the cumulative standard normal distribution and UBj and LBj are the upper and lower bounds of category j, as detailed in Table 4. Figure 5 shows the categorical data and fitted distributions for inflations expectations data pooled over the 1995–2014 period.
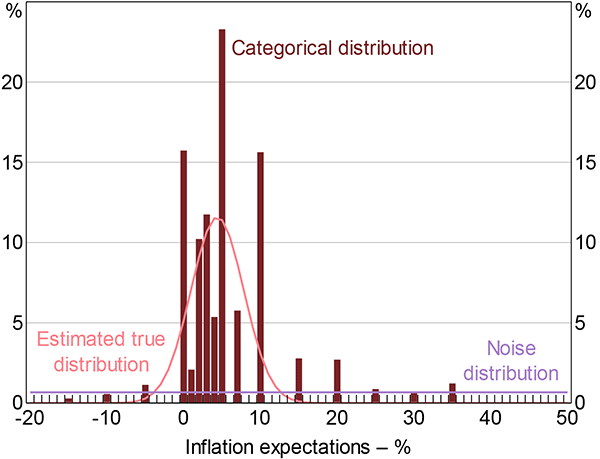
Sources: Authors' calculations; Melbourne Institute of Applied Economic and Social Research
4.2.3 Filtered data
The model's estimated parameters are shown in Figure 6, at a monthly frequency. Over time, about 5 to 10 per cent of the distribution is classified as ‘noise’, and so in effect purged when estimating the underlying parameters of the data. As a result, the true underlying distribution is estimated to be tighter as the extreme values no longer distort the results.
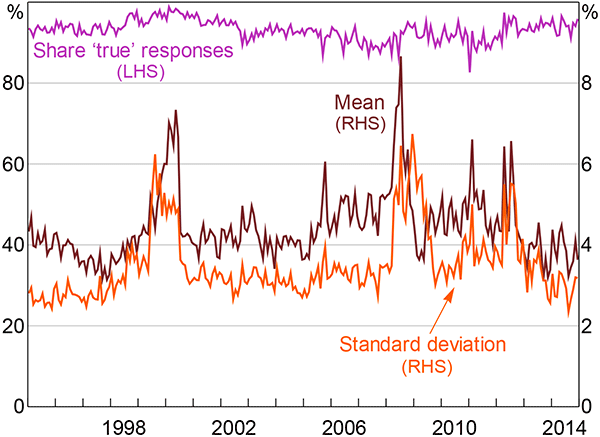
Nevertheless, given that the standard deviation is between 2 and 7 per cent over time, underlying disagreement among consumers is still substantially larger than disagreement among professional forecasters. Previous work has documented a similar pattern internationally (Ranyard et al 2008; Armantier et al 2013).
Compared with the raw data, the estimated underlying level of disagreement is much more stable and does not show a trend incline over the early and mid 2000s (Figure 7). In part, this is due to an increase in the weight on the noise distribution over this period, indicating that a greater fraction of people began expecting extremely high levels of inflation. However, most of the difference is due to extreme responses becoming more extreme. This can be inferred from there being little change over the early 2000s in the standard deviation of inflation expectations after removing the highest and lowest 15 per cent of observations each month, as shown by the trimmed distribution in Figure 7.
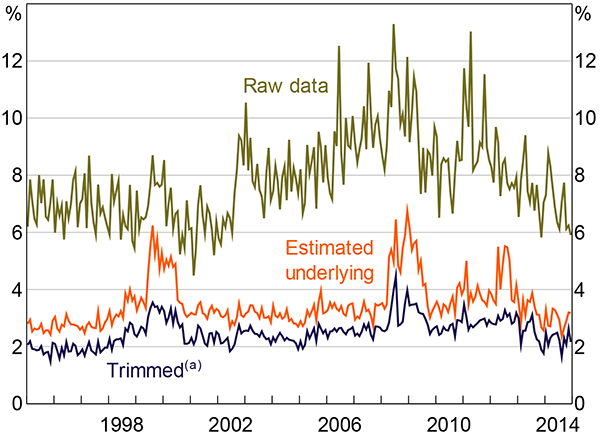
Note: (a) Based on a 30 per cent trimmed distribution
Sources: Authors' calculations; Melbourne Institute of Applied Economic and Social Research
Unlike the professional forecasts, underlying disagreement in consumer inflation expectations exhibits no trend decline over the inflation-targeting period. This could provide less compelling support for increased anchoring; however, this may simply be a result of the short sample of micro data available. In fact, if the positive correlation between the mean and standard deviation seen in Figure 6 (and formally tested in Section 5.2) holds through the history of the sample, disagreement among consumers may have fallen in the early 1990s together with the fall in mean inflation expectations (Figure 8). Unfortunately, micro data prior to 1995 are unavailable to test this possibility.
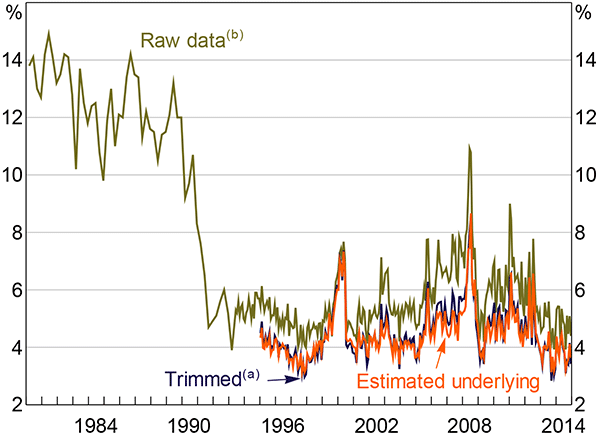
Notes:
(a) Based on a 30 per cent trimmed distribution
(b) A series break occurs prior to January 1995 due to a change in methodology
Sources: Authors' calculations; Melbourne Institute of Applied Economic and Social Research
5. Time-series Variation in Disagreement
For each survey measure, there is substantial variation over time in the level of disagreement. In this section, we assess two main aspects of this time-series variation:
- First, the extent to which it can be explained by macroeconomic news surprises. Examining the response of disagreement to economic news provides a means to discriminate between the different models of expectations formation outlined in Section 2. As indicated by Table 1, the noisy-information model and the disagreement-about-means model do not predict a response of disagreement to macroeconomic news surprises. In contrast, the sticky-information model predicts disagreement to increase in response to macroeconomic news, because only a fraction of agents are attentive to news each period. (In the absence of further shocks, disagreement is predicted to then decrease over time.)
- Second, we formally examine whether disagreement is related to mean inflation expectations or realised inflation. This is more directly related to inflation anchoring; if episodes of increased disagreement are also associated with inflation outcomes that are (temporarily) outside of the target, or with higher mean expectations, then this suggests that disagreement might be indicative of weaker anchoring. However, as discussed earlier, the absence of information on long term expectations means we cannot rule out that consumers' long-term inflation expectations remain unchanged.
5.1 Response of Disagreement to Macroeconomic News
We construct a time series of ‘macroeconomic surprises’ for each of: real GDP growth, the unemployment rate, trimmed mean inflation, CPI inflation, fuel price inflation, import price inflation, and the Australian dollar trade-weighted index.[11] These variables are chosen because they are typically used in inflation forecast models. While surprise changes in these variables are likely to lead to a revision in mean inflation expectations, their impact on forecast disagreement is less clear.
We proxy the surprise component of quarterly changes in macroeconomic variables by the residual of a univariate AR(4) model. Autoregressive model forecasts are a difficult benchmark to beat, so the autoregressive model residuals are likely to be a good measure of the surprise component of macroeconomic news releases. To facilitate comparison across variables, we scale the residuals of each series by their standard deviation.
To estimate the response of disagreement to macroeconomic news surprises, we run the following regression for each series of macroeconomic news surprises:
where σt,t+4 is disagreement in year-ahead forecasts and ωj,t is the estimated surprise component of the change in variable j.[12] We do not conduct this analysis with survey data from The Age, as the survey is run infrequently, restricting our ability to make inferences from the results. For the consumer survey, we use the standard deviation of the ‘underlying distribution’ estimated above. The estimated relationships between the macroeconomic shocks and forecast disagreement, γj,i, are plotted in Figure 9. Coefficients different from zero at the 5 per cent level of significance are denoted with a dot.[13]
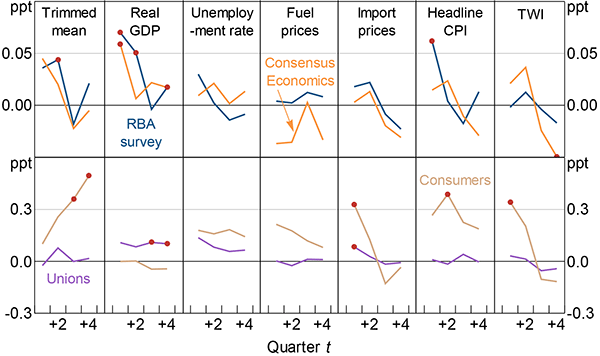
Notes: Lines depict the estimated impact of a one standard deviation surprise change in each macroeconomic variable on disagreement in inflation forecasts (as measured by the standard deviation of survey responses); dots indicate that the relationship between the macroeconomic surprise and inflation forecast disagreement is statistically different from zero at the 5 per cent level
The results provide mixed support for the notion that surprise changes in macroeconomic variables increase forecast disagreement. With the exception of the consumer survey, there is statistically significant evidence that surprise changes in real GDP are associated with an increase in disagreement about future inflation: a one standard deviation surprise in quarterly real GDP growth causes a persistent 0.1 percentage point increase in forecast disagreement among unions, and a smaller but still notable effect among respondents to the RBA and Consensus Economics surveys.
However, disagreement in the consumer survey does tend to increase in response to all the other macroeconomic surprises included here. In particular, the results suggest surprises to trimmed mean inflation are associated with a persistent and statistically significant increase in disagreement among consumer survey respondents. There is also some evidence of a positive impact of trimmed mean inflation surprises on disagreement among professional forecasters and unions.
There is less evidence of a relationship between the surprise component of other macroeconomic releases and forecast disagreement. In terms of the models discussed in Section 2, the variation in forecast disagreement in response to some surprises provides tentative evidence against the baseline noisy-information model. The noisy-information model can explain disagreement among forecasters, but it does not predict a response of disagreement to macroeconomic news surprises. While the rise in disagreement in response to real GDP growth surprises is consistent with the sticky-information model, the absence of a response of most other variables is seemingly inconsistent with the model – disagreement is predicted to rise in response to a macroeconomic surprise in any variable that affects inflation forecasts.
The rise in disagreement in response to real GDP growth surprises could reflect forecasters having different beliefs about the slope of the Phillips curve, and so updating their inflation forecasts by varying degrees in response to changes in real GDP growth. Consistent with this, there is some evidence that surprise changes in unemployment raise disagreement, although we cannot reject there being no response.
5.2 The Relationship between Disagreement and Inflation
In Section 4 we observed some suggestive visual evidence of a positive relationship between inflation and disagreement in inflation expectations. For the United States, Mankiw et al (2004) find strong evidence of such a relationship, which they argue is consistent with the staggered adjustment sticky-information model of expectations formation. But the sticky-information model is symmetric with respect to increases and decreases in inflation, suggesting that disagreement should be high whether inflation rises above or falls below the midpoint of the RBA's inflation target band.
To test these possibilities formally, we regress the measures of inflation forecast disagreement on the level of headline CPI inflation and deviations of CPI inflation from the midpoint of the target band:
where πt is year-ended CPI inflation at time t. The results, reported in Table 5, suggest that there is no relationship between inflation forecast disagreement and the level of year-ended CPI inflation. The absence of a response contrasts with Mankiw et al (2004), and may reflect the fact that the sample period used for the United States included a shift in the mean of inflation, following the Volcker disinflation, and our sample period mostly includes only the recent low-inflation period.
Consensus Economics | RBA survey | Unions: year-ahead | Unions: medium-term | Consumers | |
---|---|---|---|---|---|
β1: relationship with the level of CPI inflation | 0.005 (0.013) |
−0.008 (0.013) |
0.006 (0.009) |
−0.021 (0.015) |
0.006 (0.058) |
β2: relationship with the absolute deviation of CPI inflation from target | 0.035** (0.017) |
0.040* (0.021) |
0.082** (0.034) |
0.091*** (0.027) |
0.072 (0.063) |
Sample period | 1992–2014 | 1994–2014 | 1997–2014 | 1997–2014 | 1996–2014 |
Notes: Regressions include a constant term, four autoregressive terms, and seasonal dummies for the Consensus Economics data; sample periods differ owing to data availability; for the RBA survey, missing observations prior to 2002 are set equal to the average of adjacent quarters; ***, ** and * denote statistical significance at the 1, 5 and 10 per cent levels, respectively; Newey-West standard errors in parentheses |
However, we find that disagreement does tend to be relatively high when year-ended CPI inflation deviates from the middle of the RBA's inflation target, except for the consumer survey measure. For the Consensus Economics and RBA survey measures, a 1 percentage point deviation in year-ended CPI inflation from the midpoint of the target is estimated to raise forecast disagreement by about 0.04 percentage points, and by about 0.1 percentage point for the year-ahead and medium-term union measures. While a rise in disagreement when current inflation deviates from 2½ per cent is consistent with the sticky-information model, it is also consistent with imperfect anchoring of inflation expectations, although such inference is necessarily very tentative because our data reflect short-horizon inflation expectations.
We have tested for a relationship between disagreement and realised inflation, but the sticky-information model also predicts a relationship between disagreement and mean inflation expectations. Under this model, any news that causes attentive agents to revise their expectations will also increase disagreement. For the consumer measure, Figure 6 provides strong graphical evidence of co-movement between disagreement and mean inflation expectations. We test for such a relationship in each survey measure by respecifying Equation (6) as follows:
where is mean inflation expectations in period t,
is
mean inflation expectations over the full sample period for each measure of inflation
expectations, and all other variables are defined as before.
For the professional economist survey measures, the relationship between disagreement and expected inflation (Table 6) is qualitatively similar to the relationship between disagreement and CPI inflation. For the union officials and consumer survey measures there is evidence of a significant positive relationship between disagreement and mean inflation expectations.
Consensus Economics | RBA survey | Unions: year-ahead | Unions: medium-term | Consumers | |
---|---|---|---|---|---|
γ1: relationship with mean inflation expectations | 0.034 (0.021) |
0.002 (0.018) |
0.077** (0.034) |
0.142 (0.091) |
0.368*** (0.090) |
γ2: relationship with the absolute deviation of mean inflation expectations from series average | 0.094*** (0.035) |
0.032 (0.024) |
0.185** (0.086) |
0.120 (0.103) |
0.083 (0.128) |
Sample period | 1992–2014 | 1994–2014 | 1997–2014 | 1997–2014 | 1996–2014 |
Notes: Regressions include a constant term, four autoregressive terms, and seasonal dummies for the Consensus Economics data; sample periods differ owing to data availability; for the RBA survey, missing observations prior to 2002 are set equal to the average of adjacent quarters; ***, ** and * denote statistical significance at the 1, 5 and 10 per cent levels, respectively; Newey-West standard errors in parentheses |
For the consumer measure in particular, the relationship is statistically and economically significant, with each percentage point increase in inflation expectations associated with a one-third of a percentage point increase in disagreement. If this relationship holds in the period before the sample of unit record data starts, it suggests that disagreement in consumer inflation expectations fell sharply over 1990–91, alongside the mean and median expectations (for which data are available). This could explain the absence of a trend decline in disagreement for this measure, noted in Section 4.2.[14]
6. Are Consumer Expectations More Weakly Anchored?
There remains the question as to why disagreement in consumer inflation expectations is so much larger than among other agents. Two main explanations have been proposed (Cavallo, Cruces and Perez-Truglia 2014):
- Rational inattention: Consumers are typically not professional forecasters, so their ability and incentive to collect and process information relevant to inflation are lower; this is consistent with rational inattention (Carroll 2003; Mankiw et al 2004). However, rational inattention appears insufficient to explain the extent of disagreement among consumers. Models such as that proposed by Carroll (2003) cannot explain the magnitude of disagreement observed for consumers, nor can the sticky-information model (which also has inattentiveness at its heart). For example, these models cannot explain why a large portion of individuals persistently forecast inflation outside the range of historical experience. In addition, inattentiveness might actually suggest a narrow distribution, if many responses are always equal to the inflation target.
- Individual experience: Alternatively, individuals may use different information sets based on their personal experience, and place excess weight on some items relative to others.[15] Moreover, there are varying degrees of financial literacy among consumers, and some groups may be more prone to biases than others. Individuals may also base expectations on their lifetime average experience of inflation. As a result, disagreement may reflect different beliefs about long-run inflation, consistent with a lower degree of anchoring among households than professional forecasters.[16]
In this section, we document evidence that individual household experience explains at least part of the wide disagreement in consumer inflation expectations. There are long-run differences in consumer expectations by demographic group. These appear to be at least partly related to personal inflation experience. While these results explain only a relatively small part of overall disagreement, they are consistent with consumer disagreement being at least partly explained by ‘differences in means’.[17]
We also find that aggregate consumer inflation expectations over-react to salient prices, particularly petrol. This suggests that consumers' expectations are backward looking and so somewhat non-rational.[18] It also refutes the rational inattention explanation for consumer disagreement, which would suggest that consumers under-react to new information. One consequence of this result is that movements in relative prices in the CPI basket might see some consumers' expectations become less anchored, even where aggregate inflation outcomes are consistent with the target.
6.1 Disagreement by Demographic Groups
If consumers have different beliefs about long-run inflation, we should expect to see individuals or groups that have persistently high or low inflation expectations. Ideally, we might test this at the individual consumer level; however, the Melbourne Institute data are not panel in nature, as a new sample of individuals is surveyed each month. Accordingly, we look at persistent differences in expectations across observable demographic characteristics to identify long-run differences in mean expectations.
The Melbourne Institute data contain a set of demographic variables including gender, age, education and annual household income.[19] We divide individuals into a relatively small number of groups, which helps provide statistical power to find significant differences in inflation expectations between groups.[20] These demographic characteristics most likely serve as a proxy for unobservable factors, such as differences in financial literacy and inflation experience.
To estimate long-run differences between demographic groups we augment our filtering model of consumer inflation expectations detailed in Section 4.2 with demographic dummy variables. We also allow for differences in estimated disagreement within each demographic group by defining the standard deviation as a function of the same dummy variables. As we are interested in persistent differences, we pool the data from the full sample in order to estimate the average difference between groups. Equation (1) is now replaced by
where ηgroup is a matrix of demographic dummy variables (including a constant) and β and γ are vectors of coefficients.[21] The likelihood function for observation i is given by
The set of parameters that maximise the joint log-likelihood function for the observed survey data are reported in Table 7. The results are highly statistically significant, in large part due to the very large dataset. In addition, Wald tests confirm that the coefficients estimated for each group are statistically different to the other groups in the same demographic category.
Dummy variable | ρ | μ | σ |
---|---|---|---|
Female | 0.801*** | 0.631*** | |
18–34 years | −0.264*** | −0.042 | |
45–64 years | 0.152*** | 0.112*** | |
65+ years | −0.109*** | −0.044 | |
Non-secondary | 0.237*** | 0.264*** | |
Secondary | −0.133*** | −0.029 | |
Tertiary | −0.450*** | −0.336*** | |
Postgraduate | −0.658*** | −0.573*** | |
Under $30,000 | 0.423*** | 0.531*** | |
$81–100,000 | −0.156*** | −0.136*** | |
$100,000+ | −0.312*** | −0.127*** | |
Income refused | 0.049 | 0.206*** | |
Constant | 0.930*** | 4.321*** | 3.233*** |
Number of observations | 262,878 | ||
Notes: Estimates based on full sample 1995–2014; ***, ** and * denote statistical significance at the 1, 5 and 10 per cent levels, respectively |
Many of the estimates suggest differences in long-run means across demographic groups: males, those with higher education levels, and those with higher incomes tend to have lower inflation expectations, on average. However, the results for the age dummies do not show a systematic correlation, in line with the mixed findings in the literature (Bryan and Venkatu 2001; Blanchflower and Mac Coille 2009; Bruine de Bruin et al 2010b; Madeira and Zafar 2015).[22]
Groups that have consistently higher inflation expectations over time also have consistently greater within-group disagreement in expectations. This is in line with the finding in Section 5 that, through time, high expectations have been associated with high levels of disagreement. Rational inattention cannot explain this cross-sectional finding: less attentive groups should have higher disagreement but the same mean over time.
Figure 10 illustrates the evolution of the μ parameter on several key demographic characteristics over time, using a binary split of each demographic category. These differences in expectations by demographic group are quite stable. However, there are some periods during which these differences are smaller or larger; in particular, the higher expectations of older individuals have become statistically insignificant in recent years. There is some tentative evidence that certain groups are more susceptible to macroeconomic shocks. In particular, female respondents show a marked increase in expectations around the time of the global financial crisis. In addition, males and university-educated respondents appear to have had relatively high expectations around the time of the introduction of the GST. However, these two outcomes have opposing implications for interpreting disagreement. The GST episode supports sticky-information models of expectation formation, with males and university-educated consumers showing a greater tendency to update their information sets, whereas the global financial crisis episode implies females were more attentive to macroeconomic developments.
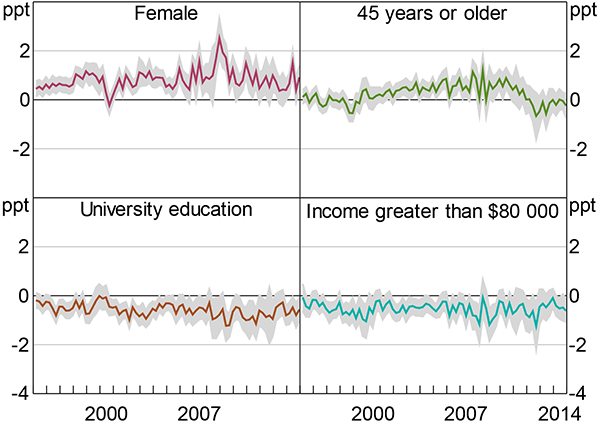
Notes: Base group is male, under 45 years of age, no tertiary education and earns $80,000 or less per annum; shaded regions represent two standard error bands
While the differences between some demographic groups are suggestive of differences in means, they only explain a small part of overall disagreement. Efron's pseudo R-squared statistic for the full sample results indicates the demographic variables only explain around 1.4 per cent of the overall variation in expectations. Thus, there is substantial variation in beliefs about inflation expectations not proxied by demographic variables.
6.2 Does Long-run Disagreement Reflect Actual Inflation Experience?
As noted above, one factor that may be related to persistent differences in inflation expectations are differences in inflation experience. Using data on household consumption from the Household Expenditure Survey (HES), we produce realised inflation series tailored to individual households in order to gauge the extent of disagreement in inflation experience.[23]
Overall, households' expectations are considerably more dispersed than their inflation experience. Across households, inflation expectations are around twice as dispersed as inflation outcomes (Figure 11).[24] However, the level of dispersion in households' realised inflation is markedly higher than the level of disagreement in professional forecasters' expectations, suggesting that differences in individual inflation experience may still explain an important part of the level, if not variation, of disagreement in consumers' expectations.
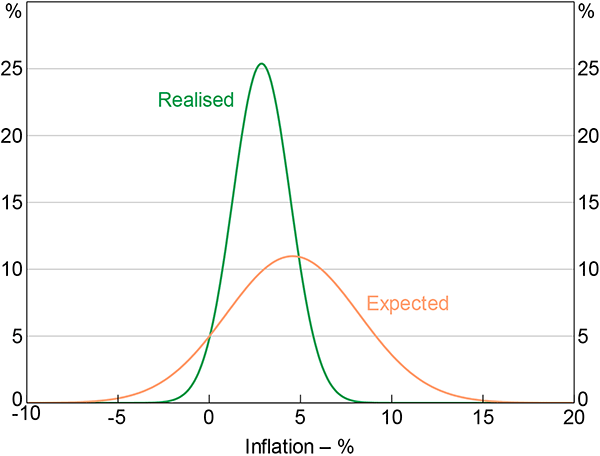
Note: Based on average quarterly mean and standard deviation outcomes for 2003–14 sample period
Sources: ABS; Authors' calculations
There is some evidence that individuals that experience higher inflation also expect higher inflation. While the data available do not link an individual household's expectations and inflation experience, we can again assess this according to demographic characteristics. By income, age and education, demographic groups that have experienced higher inflation outcomes over the past decade have also reported higher inflation expectations on average. Allowing for an approximately 1.5 percentage point upward bias in inflation expectations across all groups, there is an approximately one-for-one relationship between realised and expected inflation expectations by group over the past decade (Figure 12).
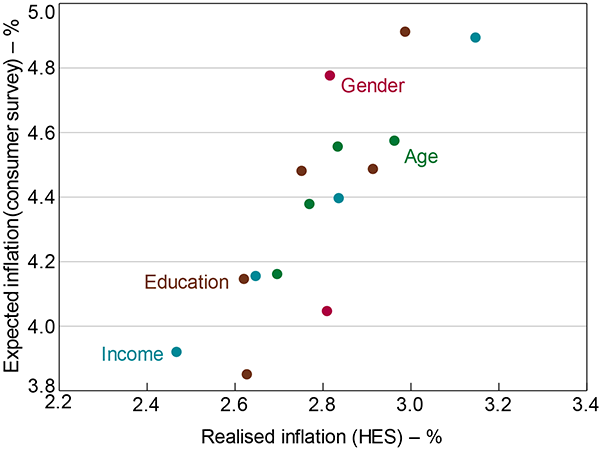
Notes: Averages over 2003–14 sample period; demographic groups split according to footnote 20, with those who refused to report their income omitted
Sources: ABS; Authors' calculations; Melbourne Institute of Applied Economic and Social Research
Again, these differences only account for a very small portion of the overall disagreement in expectations.[25] Rather, most of the variation is within demographic groups. Looking within groups, there is also a clear correlation between disagreement in expectations and dispersion in inflation outcomes (Figure 13).[26]
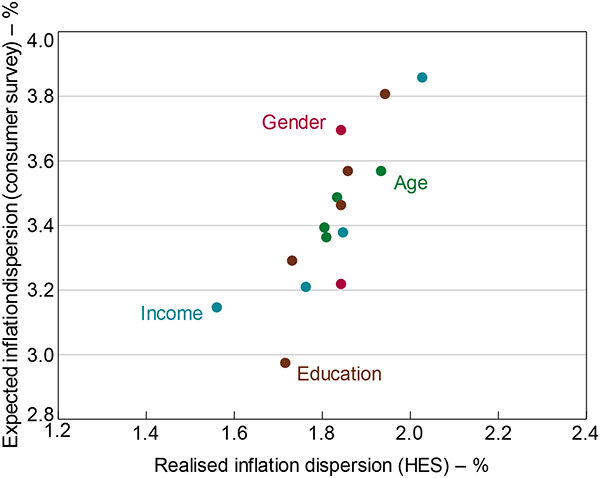
Note: See notes to Figure 12
Sources: ABS; Authors' calculations; Melbourne Institute of Applied Economic and Social Research
In part, the results for the consumer survey are likely to reflect the wording of the question that is posed to consumers. In particular, respondents are asked to think about the ‘prices of things [they] buy’. International work has demonstrated that such question wording can engender responses that are quite different from a question about ‘economy-wide inflation’ (Bruine de Bruin et al 2010a). Preliminary analysis of Australian data suggests that survey wording may have a similar effect, and further study in this area is warranted.[27]
6.3 Response of Consumer Inflation Expectations to Salient Prices
An important implication of individuals relating future inflation to their personal experience is that movements in certain salient prices may have a disproportionate effect on aggregate expectations. Examples of salient prices are petrol prices, utility prices and rents, which tend to be either volatile or move in a discrete fashion. Because changes in petrol prices are essentially unforecastable, a response of consumer inflation expectations to past changes in petrol prices would be suggestive of uninformed behaviour, or beliefs that second-round price effects of petrol price changes are large.
To test for the presence of salient price effects, we regress mean consumer inflation expectations on a range of explanatory variables, including macroeconomic factors (the unemployment rate, the output gap and the level of the cash rate). In addition, lagged movements in three salient prices are included (petrol, housing and rents), as is lagged underlying inflation, so that these salient prices effectively enter the specification as relative prices. A lag of the dependent variable is also included to control for the autoregressive nature of expectations.
The results provide strong evidence that aggregate inflation expectations are positively related to past movements in petrol prices (Table 8). While the size of the coefficient is broadly in line with the weight of petrol in the CPI basket, given that petrol prices are close to a random walk they suggest an uninformed or backward-looking response. Other salient prices are not found to be statistically significant. The sensitivity of inflation expectations to petrol prices but not to most other macroeconomic news (see Section 5) provides evidence against the pure sticky-information model, which supposes time- rather than price-dependent acquisition of information.
(1) | (2) | (3) | (4) | (5) | |
---|---|---|---|---|---|
μt−1 | 0.618*** | 0.595*** | 0.531*** | 0.642*** | 0.593*** |
underlying inflationt−1 | −0.081 | ||||
unemployment ratet−1 | 0.026 | ||||
output gapt−1 | 0.127** | ||||
cash rate | 0.012 | ||||
Δpetrol | 0.039*** | 0.032** | 0.034*** | 0.038*** | 0.034*** |
Δhousing | −0.021 | −0.047 | −0.030 | −0.021 | −0.016 |
Δrents | 0.440* | 0.374* | 0.539** | 0.432 | 0.382 |
![]() |
0.075 | ||||
![]() |
−0.078 | ||||
![]() |
0.164 | ||||
Constant | 1.281** | 1.454 | 1.370** | 1.394** | 1.010* |
Number of observations | 79 | 79 | 72 | 79 | 79 |
R-squared | 0.616 | 0.639 | 0.606 | 0.620 | 0.624 |
Note: ***, ** and * denote statistical significance at the 1, 5 and 10 per cent levels, respectively |
7. Is Disagreement a Proxy for Forecast Uncertainty?
Despite conceptual differences, disagreement among forecasters is often used as a proxy for mean forecast uncertainty (Bloom 2014). In theory, an increase in disagreement can occur when there is no change in uncertainty – if at least some agents change their reported expectation, but all are no less sure about the likely outcome – but in practice forecaster disagreement co-moves with other measures of economic uncertainty (e.g. mentions of economic uncertainty in newspapers).
In this section, we investigate whether disagreement in inflation expectations is related to the magnitude of RBA inflation forecast errors. We assess predictability of the magnitude of RBA forecast errors, rather than the forecast errors in the mean expectation of each survey measure, because these forecasts are of most interest for the setting of monetary policy.
Figure 14 shows a scatterplot of absolute RBA year-ahead CPI inflation forecast errors against disagreement in CPI inflation expectations. For the Consensus Economics and RBA survey measures, there is some evidence that large forecast errors were preceded by a relatively high level of disagreement among survey respondents.
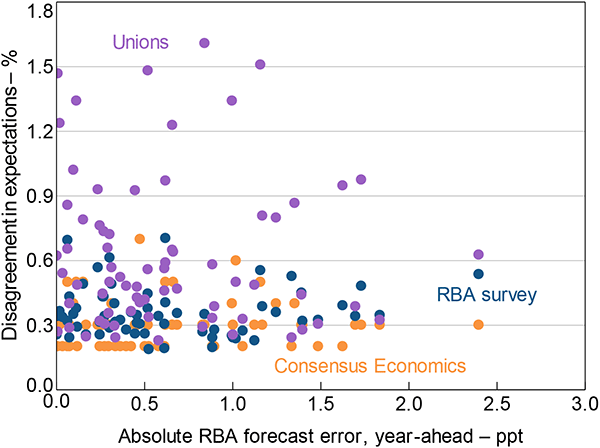
Note: Datum points are quarterly combinations of year-ahead absolute RBA forecast error and survey forecast disagreement lagged one quarter, which matches the RBA forecast error with the survey disagreement available at the time the RBA forecast was made
Sources: Authors' calculations; Consensus Economics; RBA; Workplace Research Centre
We formally test for a relationship between forecast disagreement and the magnitude of RBA CPI inflation forecast errors by estimating the following regression for each inflation survey measure:
where πt + h is CPI inflation over the period
t to t + h, πt + h|t is the
time t RBA forecast, and thus is the magnitude of
the RBA's inflation forecast error. We use the standard deviation of year-ahead inflation
forecasts for each survey measure i as our measure of disagreement: σt:t + 4. The
absolute value of past forecast errors,
, are included as a
control variable.
Consistent with the graphical evidence, the estimated β parameters are positive for the Consensus Economics and RBA survey measures, suggesting some positive relationship between forecast disagreement and the magnitude of RBA forecast errors, but the relationship is not precisely estimated. There is no evidence of a relationship between disagreement and forecast errors for the union and consumer survey measures. Taken together, the regression results reported in Table 9 provide relatively weak evidence that forecast disagreement can be used to predict the magnitude of RBA inflation forecast errors.
RBA forecast error | Consensus Economics | RBA survey | Unions | Consumers |
---|---|---|---|---|
Four-quarters-ahead | 0.326 (0.213) |
0.154 (0.398) |
−0.050 (0.134) |
−0.067 (0.056) |
Five-quarters-ahead | 0.393* (0.231) |
0.626 (0.387) |
−0.060 (0.118) |
−0.117 (0.049) |
Six-quarters-ahead | 0.655 (0.412) |
1.098 (0.453) |
0.077 (0.109) |
−0.150 (0.048) |
Notes: Regressions include a constant term and two autoregressive terms, and are run for the full sample period that the survey data are available; ***, ** and * denote statistical significance at the 1, 5 and 10 per cent levels, respectively; Newey-West standard errors in parentheses |
8. Conclusions
This paper provides some of the first evidence for Australia on the behaviour of disagreement about inflation expectations, contributing to a growing international literature studying forecast disagreement for macroeconomic variables. Our findings have implications for the anchoring of inflation expectations and the manner in which agents form and update their expectations. While the behaviour of disagreement does not fit neatly within any particular model, we do find evidence consistent with information rigidities.
In summary, our key findings are:
- There has been a decline in disagreement in inflation expectations among professional forecasters since the 1980s and early 1990s. This is consistent with inflation expectations having become better anchored at the RBA's inflation target since the adoption of inflation targeting in 1993.
- Disagreement responds little to most macroeconomic news surprises. However, disagreement about inflation over the year ahead does rise in response to deviations in current inflation from the midpoint of the RBA's inflation target. This is consistent with the presence of information rigidities, but also imperfect credibility of the inflation target.
- Consumers' inflation expectations appear more weakly anchored than professionals: disagreement among consumers about inflation expectations is an order of magnitude larger than among professional forecasters, and there is no evidence of a decline in disagreement since 1995.
- There is a strong relationship between disagreement and the mean level of inflation expectations for consumers, but not for professional forecasters. This implies that reductions in consumer inflation expectations prior to 1995 may have been associated with a decline in disagreement.
- There are persistent differences in consumer inflation expectations across demographic groups, which appear partly related to personal inflation experience. However, these differences explain only a small fraction of the overall disagreement in consumer inflation expectations.
- Consumer inflation expectations are sensitive to certain salient prices, such as past changes in petrol prices. This is inconsistent with pure sticky-information-type models of expectations formation, which assume that updating of expectations is time-dependent.
- On a methodological level, we introduce a new technique to remove noise from individual response level consumer inflation expectations data.
- We find only weak evidence that disagreement may be a useful proxy for mean forecast uncertainty. This suggests that there is not meaningful predictable time variation in inflation forecast confidence bands.
Appendix A: Extending the Model of Consumer Inflation Expectations
In Section 4.2 we presented a method for estimating the mean and standard deviation of consumer inflation expectations that is robust to bunching at round numbers and extreme values. The disproportionately large share of observations at certain round numbers, particularly 0, 5 and 10, led us to make a distinction between ‘rounded’ observations occurring at multiples of five (representing inflation expectations in a range around the reported value) and ‘precise’ observations not equal to a multiple of five in the range 0 to 10.
For simplicity, we made the assumption that rounded and precise observations are drawn from the same distribution. However, this need not be the case. Here, we extend the framework in Section 4.2 to allow for different underlying distributions of consumer inflation expectations between respondents reporting an expectation equal to a multiple of five and those not reporting an expectation equal to a multiple of five. Furthermore, we previously assumed that all inflation expectations reports of 0, 5 and 10 were rounded expectations, which introduces some imprecision into our estimates if some reports at 0, 5 and 10 represent precise estimates. We now estimate the fraction of reports that are precise and rounded, which no longer requires us to assume that all observations at 0, 5 and 10 represent rounded observations.
Formally, under this revised methodology, the likelihood that a response to the inflation expectations survey lies within category j is given by:
where μp and σp are the mean and standard deviation of the distribution of precise expectations; μr and σr are the mean and standard deviation of the distribution of rounded expectations; θ is the fraction of precise expectations; 1 − ρ is the fraction of noisy observations; UBj and LBj are the upper and lower bounds of category j, as detailed in Table 4; and Φ is the standard normal cumulative density function. We jointly estimate these parameters for each month's survey data of consumer inflation expectations by numerically maximising the log-likelihood function.
Next, we combine the estimated precise and rounded distributions to form an estimated population distribution of underlying consumer inflation expectations. Assuming independence of the precise and rounded distributions, the combined distribution is normal with mean μ and variance σ2, where
with all parameters as defined earlier.
Figure A1 shows a time series plot of the mean and standard deviation of the combined distribution, together with the equivalent estimates from Section 4.2, using the simpler method outlined in the main text. Given the similarity of the series, we have chosen to conduct our analysis using the results from the simpler of the two methods.
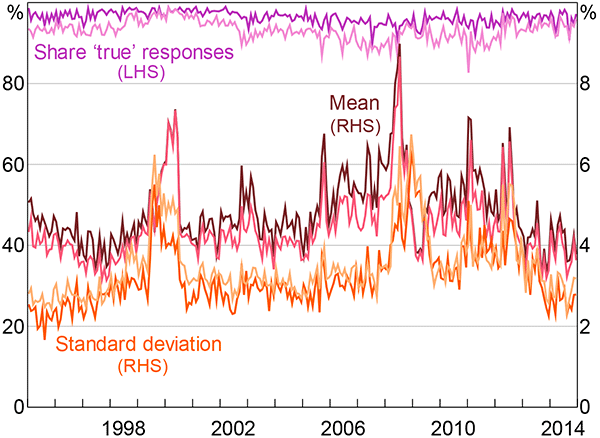
Note: The original estimates from Section 4.2 are shown in lighter colour
References
Andrade P, RK Crump, S Eusepi and E Moench (2014), ‘Fundamental Disagreement’, Federal Reserve Bank of New York Staff Report No 655, rev November.
Armantier O, W Bruine de Bruin, S Potter, G Topa, W van der Klaauw and B Zafar (2013), ‘Measuring Inflations Expectations’, Annual Review of Economics, 5, pp 273–301.
Ball L (1992), ‘Why Does High Inflation Raise Inflation Uncertainty?’, Journal of Monetary Economics, 29(3), pp 371–388.
Bayer C and F Juessen (2015), ‘Happiness and the Persistence of Income Shocks’, American Economic Journal: Macroeconomics, 7(4), pp 160–187.
Blanchflower DG and C Mac Coille (2009), ‘The Formation of Inflation Expectations: An Empirical Analysis for the UK’, Paper presented at the XI Annual Inflation Targeting Seminar of the Banco Central do Brasil, Rio de Janeiro, 14–15 May.
Bloom N (2014), ‘Fluctuations in Uncertainty’, The Journal of Economic Perspectives, 28(2), pp 153–176.
Brischetto A and G de Brouwer (1999), ‘Householders' Inflation Expectations’, RBA Research Discussion Paper No 1999-03.
Bruine de Bruin W, W van der Klaauw, JS Downs, B Fischhoff, G Topa and O Armantier (2010a), ‘The Effect of Question Wording on Reported Expectations and Perceptions of Inflation’, Federal Reserve Bank of New York Staff Report No 443.
Bruine de Bruin W, W van der Klaauw, JS Downs, B Fischhoff, G Topa and O Armantier (2010b), ‘Expectations of Inflation: The Role of Demographic Variables, Expectation Formation, and Financial Literacy’, The Journal of Consumer Affairs, 44(2), pp 381–402.
Bruine de Bruin W, W van der Klaauw and G Topa (2011), ‘Expectations of Inflation: The Biasing Effect of Thoughts about Specific Prices’, Journal of Economic Psychology, 32(5), pp 834–845.
Bryan MF and G Venkatu (2001), ‘The Demographics of Inflation Opinion Surveys’, Federal Reserve Bank of Cleveland Economic Commentary, 15 October.
Capistrán C and M Ramos-Francia (2010), ‘Does Inflation Targeting Affect the Dispersion of Inflation Expectations?’, Journal of Money, Credit and Banking, 42(1), pp 113–134.
Carroll CD (2003), ‘Macroeconomic Expectations of Households and Professional Forecasters’, The Quarterly Journal of Economics, 118(1), pp 269–298.
Cavallo A, G Cruces and R Perez-Truglia (2014), ‘Inflation Expectations, Learning and Supermarket Prices: Evidence from Field Experiments’, NBER Working Paper No 20576.
Coibion O and Y Gorodnichenko (2012), ‘What Can Survey Forecasts Tell Us about Information Rigidities?’, Journal of Political Economy, 120(1), pp 116–159.
Friedman M (1977), ‘Nobel Lecture: Inflation and Unemployment’, Journal of Political Economy, 85(3), pp 451–472.
Jacobs D, D Perera and T Williams (2014), ‘Inflation and the Cost of Living’, RBA Bulletin, March, pp 33–46.
Kumar S, H Afrouzi, O Coibion and Y Gorodnichenko (2015), ‘Inflation Targeting Does Not Anchor Inflation Expectations: Evidence from Firms in New Zealand’, Paper presented at the Fall 2015 Brookings Panel on Economic Activity, Washington DC, 10–11 September.
Lucas Jr RE (1972), ‘Expectations and the Neutrality of Money’, Journal of Economic Theory, 4(2), pp 103–124.
Mackowiak B and M Wiederholt (2009), ‘Optimal Sticky Prices under Rational Inattention’, The American Economic Review, 99(3), pp 769–803.
Madeira C and B Zafar (2015), ‘Heterogeneous Inflation Expectations and Learning’, Journal of Money, Credit and Banking, 47(5), pp 867–896.
Malmendier U and S Nagel (2016), ‘Learning from Inflation Experiences’, The Quarterly Journal of Economics, 131(1), pp 53–87.
Mankiw NG and R Reis (2002), ‘Sticky Information versus Sticky Prices: A Proposal to Replace the New Keynesian Phillips Curve’, The Quarterly Journal of Economics, 117(4), pp 1295–1328.
Mankiw NG, R Reis and J Wolfers (2004), ‘Disagreement about Inflation Expectations’, in M Gertler and K Rogoff (eds), NBER Macroeconomics Annual 2003, 18, The MIT Press, Cambridge, pp 209–248.
Pagan A (1984), ‘Econometric Issues in the Analysis of Regressions with Generated Regressors’, International Economic Review, 25(1), pp 221–247.
Patton AJ and A Timmermann (2010), ‘Why Do Forecasters Disagree? Lessons from the Term Structure of Cross-Sectional Dispersion’, Journal of Monetary Economics, 57(7), pp 803–820.
Ranyard R, F Del Missier, N Bonini, D Duxbury and B Summers (2008), ‘Perceptions and Expectations of Price Changes and Inflation: A Review and Conceptual Framework’, Journal of Economic Psychology, 29(4), pp 378–400.
Reis R (2006), ‘Inattentive Producers’, The Review of Economic Studies, 73(3), pp 793–821.
Sims CA (2003), ‘Implications of Rational Inattention’, Journal of Monetary Economics, 50(3), pp 665–690.
Woodford M (2003), ‘Imperfect Common Knowledge and the Effects of Monetary Policy’, in P Aghion, R Frydman, J Stiglitz and M Woodford (eds), Knowledge, Information, and Expectations in Modern Macroeconomics: In Honor of Edmund S. Phelps, Princeton University Press, Princeton, pp 25–58.
Acknowledgements
We would like to thank Andrea Brischetto, James Hansen, Greg Kaplan, Christopher Kent and John Simon for helpful comments. We are grateful to Dilhan Perera for his work on individual inflation experience on which we have drawn.
Footnotes
For the United States, Mankiw, Reis and Wolfers (2004) is a notable exception. More recently, Andrade et al (2014) analyse the term structure of disagreement for inflation, GDP growth, and the federal funds rate. For Australia, Brischetto and de Brouwer (1999) document differences in mean consumer inflation expectations by demographic characteristics among respondents to the Melbourne Institute survey of households' inflation expectations. [1]
From the beginning of the sample until February 1997, the medium-term question referred to inflation ‘through the rest of the 1990s’ and between May 1997 and August 1998, the medium-term question referred to inflation ‘through to 2000’. After August 1998, the question refers to inflation over the next 5 to 10 years. [2]
The correlation between these measures is not primarily due to a common downward trend. For the detrended series, the correlation between the RBA and union survey measures falls to 0.19, but the other pairwise correlations are similar. [3]
The pre-inflation targeting maximum inflation rate was around 25 per cent, during the early 1950s wool boom. [4]
Another possibility is that these extreme values result from data entry errors, which would also act to introduce noise and distort measures of disagreement. [5]
An evaluation of our approach relative to more sophisticated non-parametric methods in the robust statistics literature is beyond the scope of this paper. The validity of the structure we impose depends on the underlying data generating process, which is unknown. [6]
Some fraction of responses equal to a multiple of five may represent ‘precise’ answers. Appendix A outlines a method to estimate this fraction. The estimated parameters of interest are similar using this more complicated method to those using the model outlined here. [7]
We discard twelve observations below −50 per cent and one observation above 100 per cent from the dataset since they are particularly extreme outliers. [8]
The lowest and highest categories are not centred on j, but this has a negligible effect because observations within this range are almost entirely accounted for by the ‘noise’ distribution, as described in what follows. [9]
Observations are weighted by their sampling frequency, as is standard for Melbourne Institute's stratified dataset. [10]
The two CPI series exclude interest charges prior to the September quarter 1998 and are adjusted for the tax changes of 1999–2000. Removing the GST effect is necessary as the policy was anticipated, so it should not be captured as a macroeconomic surprise. [11]
For the regressions with disagreement in Consensus Economics expectations, we also include a set of quarterly dummy variables to remove the mechanical effect of changes in the forecast horizon on uncertainty. [12]
Pagan (1984) shows that under the null hypothesis that γj,i = 0 the standard errors do not need to be adjusted for the use of a generated regressor. [13]
If this is the case, it indicates that consumers were more attentive to the taming of inflation and/or introduction of inflation targeting in the early 1990s compared with professional forecasters and unions. This runs counter to our prior beliefs. [14]
See, for example, Bruine de Bruin, van der Klaauw and Topa (2011), Madeira and Zafar (2015) and Malmendier and Nagel (2016). [15]
Inflation learning may also occur more slowly than for professionals, in which case an individual's expectations may appear backward looking. [16]
It may be the case that some consumers fundamentally do not understand the survey question. [17]
Another possibility is that consumers believe there are large second-round price effects of petrol price changes. [18]
The dataset also includes variables for home ownership, voting intention and location. [19]
These groupings are split along a number of dimensions: male and female; ages 18–34, 35–44, 45–64, and 65 years and over; education level non-secondary, secondary, vocational, tertiary (including diplomas and undergraduate degrees) and postgraduate; incomes $30,000 or under, $31,000–80,000, $81,000–100,000, over $100,000 and those who refused to provide their income. [20]
We use males aged 35–44 years with vocational education and income of $30,000–80,000 as our base group, which is captured by the constant. [21]
This is despite there being a clear difference in raw means across the age groups. This is a result of collinearity between age and income; around half of the respondents aged 65 or older also have an annual income of $30,000 or less. Accordingly, the higher raw average inflation expectation of older respondents is empirically explained by their lower income. [22]
See Jacobs, Perera and Williams (2014). [23]
Moreover, periods of elevated disagreement in inflation expectations do not coincide with periods of increased dispersion in inflation outcomes. [24]
Efron's R-squared, equal to 0.0035, suggests that long-run differences in realised inflation between demographic groups explain little of the variation in inflation expectations. [25]
There are some limitations in reaching this conclusion. In particular, differences in measured inflation experience between groups might partly reflect measurement issues – notably, that the dispersion of inflation outcomes is based upon certain assumptions, and so may under-estimate the full dispersion of household experiences. For example, differences in measured inflation experience between groups does not capture differences in inflation rates for the same good or service within a given city. For more details on the construction of these data, see Jacobs et al (2014). [26]
Preliminary analysis has been conducted using data from a trial survey question asking consumers about expected ‘inflation’ rather than changes in the ‘prices of things you buy’. The ‘inflation’ question elicited fewer extreme responses than the ‘prices of things you buy’ question, and a tighter distribution around the midpoint of the RBA's inflation target. [27]