RDP 2013-01: Currency Demand during the Global Financial Crisis: Evidence from Australia 4. Modelling Currency Demand in Australia
January 2013 – ISSN 1320-7229 (Print), ISSN 1448-5109 (Online)
- Download the Paper 634KB
In this section, we estimate how much of the increase in currency demand in late 2008 can be explained by traditional factors (i.e. interest rates and income movements). A large unexplained rise in currency holdings in this period would be consistent with an increase in precautionary demand. We consider banknote demand split by denomination, as well as total currency on issue, to help identify transactional demand compared to store-of-value or precautionary demand. To achieve these aims, we draw upon three strands of the literature: traditional models, transaction cost models and financial crisis models.
4.1 Traditional Models
Most money demand models include income and interest rates as explanatory variables. Income is positively related to money demand because demand for transaction balances increases in line with income. Interest rates are negatively related to money demand because a higher interest rate increases the opportunity cost of holding money. Early Australian money demand models following this literature include Cohen and Norton (1969) and Stevens, Thorp and Anderson (1987).
de Brouwer, Ng and Subbaraman (1993) explore the sensitivity of Australian money demand relationships to specification changes across various definitions of monetary aggregates, economic activity and interest rates. They find the real long-run demand for currency to be a function of real income and an opportunity cost variable. However, they find the existence of cointegrating relationships to be highly dependent on the definitions of variables.
Lim (1995) establishes a cointegrating relationship between M1, income, the rate of return on money and the rate of return on other assets using an error correction model estimated on Australian data.[12] In contrast, Felmingham and Zhang (2001) only find a cointegrating relationship between a broad definition of Australian money, GDP, interest rate spreads and inflation.
In our model, we use currency holdings of the non-bank sector to represent currency demand, as this series captures the behaviour of the public.[13] We also consider total banknotes on issue because these data allow for separate modelling of demand for different denominations.[14] The changes to banknote distribution in 2001 discussed in Section 3.2 mean that there is a level shift in the total banknotes-on-issue series. We control for this shift using a dummy variable. The changes to distribution arrangements also resulted in a transitory spike in banknote holdings for the three quarters after the change, so we include temporary dummy variables in the affected quarters.
To capture the opportunity cost of holding currency, we include a composite interest rate for retail deposits. This rate is a weighted average of at-call savings deposit rates and term deposit rates.[15] We use nominal GDP as our income variable, although the results are robust to other measures.
4.2 Transaction Cost Models
We also consider models that explicitly include transaction costs. Models that omit these influences may be misspecified and thus have biased estimates of the effect of interest rates on currency holdings. This method is particularly useful when modelling the determinants of high- and low-denomination currency demand separately because the effects of transaction costs on demand are likely to be different for different denominations.
Baumol (1952) and Tobin (1956) develop inventory models of the demand for currency, in which consumers take into account the cost incurred per withdrawal (including the opportunity cost of time and effort). If withdrawing currency becomes less costly, consumers will withdraw less currency more often and thus hold less cash on average. This idea has been incorporated into empirical models by including the number of ATMs and EFTPOS terminals into money demand models (Drehmann, Goodhart and Krueger 2002; Amromin and Chakrovorti 2009). They find a weak relationship between these transaction cost variables and currency demand.
To capture transaction cost effects, we include the number of ATMs, the number of EFTPOS terminals and the number of bank branches per capita. Over the past two decades, ATM and EFTPOS terminal numbers have increased substantially while bank branches per capita have declined (Figure 10). More of these currency access points make it easier to obtain currency, which would tend to decrease the average holdings of currency by the public. EFTPOS terminals also allow substitution from cash to debit cards. Working in the other direction, however, ATM providers demand more banknotes to stock the extra machines.
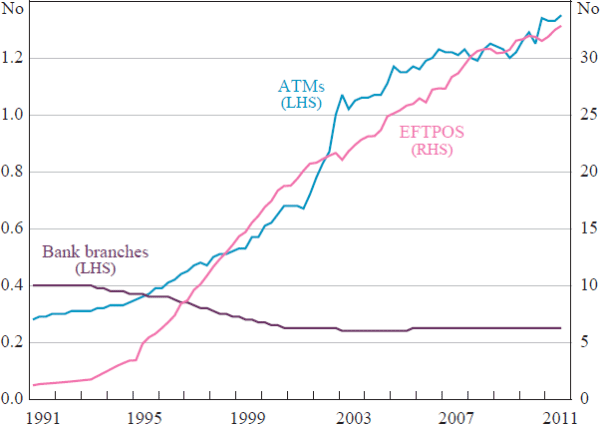
Sources: ABS; Australian Payments Clearing Association
Judson and Porter (2004) find a positive relationship between the share of small businesses and banknote demand across all denominations. Amromin and Chakrovorti (2009) include the ratio of self-employment to total employment in the economy to measure the number of small businesses and they find it to be positively related to demand. They suggest two reasons for this relationship. First, small businesses are less likely to have EFTPOS terminals. Second, small businesses are more likely to undertake the quick low-value transactions that are the most likely to be conducted using cash (see also Emery, West and Massey 2007). We include the ratio of self-employment to total employment to capture this effect.
4.3 Financial Crisis Models
There is some literature on currency demand during financial crises. Bjørnland (2003) finds that currency demand increased substantially during the Venezuelan banking crisis of the mid 1990s, but returned to trend in the long run. Miyagawa and Morita (2009) find a cointegrating relationship between money demand, interest rates, GDP and a ‘financial anxiety’ variable that holds through the financial crises in Finland and Japan during the early 1990s. The financial anxiety variable – derived from business survey data – accounts for a strong rise in money demand, which the authors attribute to a rise in precautionary balances in those countries during these crises. Khamis and Leone (1999) use Mexican data to find a stable cointegrating relationship between real currency balances, real private consumption expenditure and an interest rate, even during the financial crisis in the 1990s.
Few papers have examined the stability of money demand over the most recent financial crisis. Beyer (2009) uses euro area data and finds a stable cointegrating relationship within a vector error correction model that includes M3 over a sample that includes the financial crisis. The inclusion of a wealth variable is crucial to this stability.
We attempt to capture the effects of the global financial crisis (GFC) on currency demand in three ways. First, we add dummy variables for the three quarters from December 2008 to June 2009 to the baseline model. Second, we introduce confidence, financial market and wealth variables to our model. Finally, we examine whether these wealth variables retain any explanatory power in the presence of dummy variables. We would expect rises in the stock of currency to be associated with declines in confidence and wealth variables, and rises in financial volatility variables. In particular, we look at the NAB business confidence survey, the Westpac-Melbourne Institute consumer sentiment survey, stock market volatility and household wealth. These variables were all affected in a highly correlated fashion during the global financial crisis, but none can be considered to directly measure households' confidence in the banking system. As a result, at best we can only proxy for the precautionary demand motive.
4.4 Estimating Error Correction Models
Following de Brouwer et al (1993) we model currency demand in an error correction framework to exploit the possible cointegration between currency holdings, GDP and interest rates. We also include ATMs, EFTPOS terminals, bank branches per capita and the ratio of self-employment to total employment in the long-run relationship to form a general error correction model:[16]
where ct is the currency stock in period t, β is a vector of long-run parameters, Xt is a vector of variables including nominal GDP, the deposit interest rate, ATMs per capita, EFTPOS terminals per capita, bank branches per capita, the ratio of self-employment to total employment and β0 is a constant. All variables except the interest rate are in logarithms. The speed of adjustment parameter is λ, and the parameters for the dynamic terms are δi and γj. The residual is εt. We estimate the model using quarterly data from March 1993.
4.4.1 Non-bank sector currency holdings results
We start with currency holdings of the non-bank sector as the dependent variable. All variables from the cointegrating vector in Equation (1) are significant so were retained, except the self-employment variable because its sign was negative (which goes against its theoretical rationale).[17] Among the dynamic terms, only the first lag of changes in the currency stock is significant so it is the only dynamic term retained.
Focusing initially on the pre-GFC sample, the coefficients on GDP and the interest rate are significant and have the expected signs (Table 2). The elasticity on GDP is not significantly different from unity, suggesting that GDP growth is met with commensurate growth in the currency stock in the long run. The semi-elasticity of the currency stock with respect to the deposit rate is −1.3 per cent. This implies that a permanent 100 basis point decrease in the deposit rate is associated with a $520 million long-run increase in the currency stock, based on the level in mid 2008. The magnitude of the semi-elasticity is broadly consistent with that estimated in de Brouwer et al (1993).
1993:Q1–2008:Q2 | 1993:Q1–2011:Q4 | ||
---|---|---|---|
Without GFC dummies | With GFC dummies | ||
Speed of adjustment (λ) | −0.23*** | −0.26*** | −0.23*** |
GDPt − 1 (β1) | 1.07*** | 1.02*** | 1.00*** |
DepositRatet − 1 (β2) | −0.013* | −0.005 | −0.011*** |
ATMt − 1 (β3) | −0.16** | −0.12*** | −0.12*** |
EFTPOSt − 1 (β4) | −0.06*** | −0.04*** | −0.06*** |
BankBranchest − 1 (β5) | −0.59*** | −0.50*** | −0.55*** |
Constant (β0) | 4.93** | 6.52*** | 6.39*** |
Δct − 1 (δ1) | −0.30** | 0.15 | −0.17 |
Dummy variables | |||
GFC (2008:Q4) | 0.044*** | ||
GFC (2009:Q1) | 0.026*** | ||
GFC (2009:Q2) | 0.015** | ||
Adjusted R2 | 0.41 | 0.31 | 0.63 |
Standard error | 0.0046 | 0.0070 | 0.0048 |
LM(5) test(a) | 0.20 | 0.31 | 0.23 |
Chow test (mid sample)(a) | 0.04 | 0.26 | 0.30 |
Notes: ***, ** and * indicate significance at the 1, 5 and 10 per cent level,
respectively (a) p-value of F-statistic reported |
The negative coefficients on ATMs, EFTPOS terminals and bank branches are in keeping with theory. That is, by making it easier to access cash (or pay without cash), these variables are inversely related to currency demand. To give some scale to the magnitudes of the coefficients of these variables, a 10 per cent increase in ATMs and EFTPOS terminals per capita is estimated to reduce currency demand by 1.6 and 0.6 per cent respectively. A 1 per cent reduction in bank branches per capita is estimated to increase currency holdings by 0.59 per cent.[18]
Overall, non-bank currency holdings grew by 6 per cent per year on average over the period. Nominal GDP was the main driver, accounting for around 7 per cent average annual growth. The net effect of trends in ATMs, EFTPOS terminals and bank branches was negative and in the order of an average reduction of 1 per cent per year in currency holdings.
Extending the sample to include the surge in currency during the global financial crisis (but with no dummy variables) leads to some changes in the coefficients. In particular, the interest rate becomes insignificant and the lagged dependent variable changes sign and becomes insignificant. With the inclusion of GFC dummy variables, the coefficient on the deposit rate estimated over the full sample is not significantly different to the pre-GFC sample estimation. The coefficient on lagged currency changes switches back to its original sign, but remains insignificant. No other coefficient estimates are significantly different in the two samples. A Chow breakpoint test at December 2008 rejects the null hypothesis of parameter stability, which is consistent with unusual currency demand behaviour from that point.
We can use the coefficients estimated in the pre-GFC sample to generate estimates of the effects of the changes in interest rates on currency demand. The fall in the deposit rate between the September quarter 2008 and the June quarter 2009 of 343 basis points implies a long-run increase of $1.8 billion in currency demand. However, the short-run effects are much smaller. The estimated response of currency demand to the changing deposit rate in the late 2008–early 2009 period is around $500 million, only a small fraction of the overall rise (Figure 11).
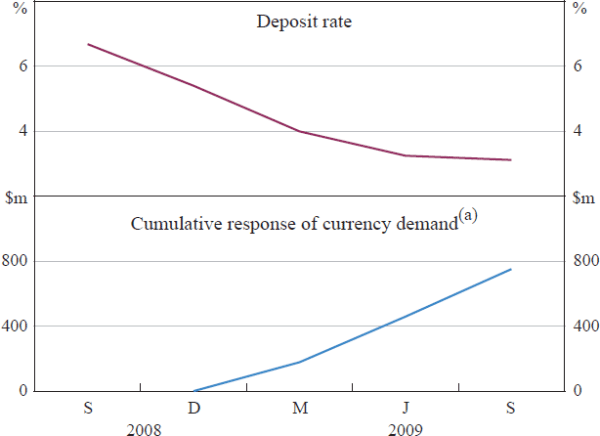
Note: (a) The response is calculated based on coefficients estimated in the error correction model using the 1993:Q1–2008:Q2 sample
Sources: RBA; authors' calculations
The model is less well-suited to generating estimates of the effect of the fiscal stimulus payments on currency demand, although this effect will be captured to some extent through the GDP variable.[19] Overall, the dummy variables suggest that 4.4 percentage points of the 6.5 per cent rise in the December quarter 2008 can be attributed to factors other than interest rates and nominal GDP, and that almost all of the further rises in the March and June quarters of 2009 were unexplained by the model (Table 3).
Δ Non-bank currency % | Attributed to dummy ppts | |
---|---|---|
December 2008 | 6.5 | 4.4 (0.6) |
March 2009 | 2.7 | 2.6 (0.8) |
June 2009 | 1.8 | 1.5 (0.6) |
Total | 11.0 | 8.5 (1.3) |
Note: Standard errors of estimated coefficients are in parentheses |
Around 80 per cent of the rise in currency holdings during the global financial crisis, therefore, cannot be explained by the standard explanatory variables, which is consistent with it being an unusual increase in precautionary demand for currency.[20]
4.4.2 Financial crisis models
We now examine whether confidence and financial variables add explanatory power to the baseline error correction model, and compare them to the GFC dummy variables. The results shown in Table 4 show that a number of these variables, when included individually, improve the explanatory power of the model overall. However, they do not improve the explanatory power of the models in the pre-GFC sample, and the explanatory power of the variables disappears in the presence of the GFC dummy variables.
Financial crisis variables(a) | Sign | Additional R2 | |
---|---|---|---|
GFC dummies included |
|||
GFC dummy variables | + | 0.31 | |
TWI | – | 0.23 | 0.01 |
VIX | + | 0.14 | 0.00 |
ASX volatility | + | 0.12 | 0.00 |
Business confidence | – | 0.09 | 0.00 |
Household wealth(b) | – | 0.05 | 0.00 |
ASX 200(b) | – | 0.04 | 0.01 |
Consumer confidence | – | 0.00 | 0.00 |
Notes: (a) Each included separately in the baseline model shown in column
2 of Table 2 (b) Variables expressed in logarithms |
4.4.3 Estimation of banknotes on issue by denomination
Separate models of currency demand for different denominations allow us to test whether low-denomination banknotes are less sensitive to interest rates, which would be the case if the demand for these banknotes is driven more by transaction needs. We can also examine whether demand for high-denomination banknotes was more sensitive to the concerns that drove precautionary holdings of cash during the global financial crisis. We estimate separate models using low-denomination banknotes (the sum of five and ten dollar banknotes), fifty dollar banknotes and one hundred dollar banknotes.[21] The denomination splits are available only for all banknotes on issue, not just non-bank holdings as above, so we estimate a model of total banknotes on issue as well for comparison.
Table 5 shows the regression results. We removed insignificant variables, leaving us with slightly different models for each independent variable.[22] The specification using total banknotes on issue is similar to the non-bank currency holdings specification in Table 2. The insignificant ATM coefficient could be because the extra currency used by the bank sector to stock ATMs offsets the negative effect of ATMs on non-bank sector currency holdings (as in Table 2). Also, the coefficient on the lagged changes in total banknotes is insignificant.
Total | Low-denomination | $50 | $100 | |
---|---|---|---|---|
Speed of adjustment (λ) | −0.28*** | −0.35*** | −0.36*** | −0.10*** |
GDPt − 1 (β1) | 0.88*** | 0.57*** | 0.82*** | 1.14*** |
DepositRatet − 1 (β2) | −0.011*** | −0.010** | −0.017*** | −0.019* |
ATMt − 1 (β3) | – | – | 0.10* | – |
EFTPOSt − 1 (β4) | −0.04** | −0.02** | 0.08*** | −0.22*** |
BankBranchest − 1 (β5) | −0.42*** | – | – | −1.05*** |
Constant (β0) | 9.87*** | 13.87*** | 14.45*** | – |
Δct − 1 (δ1) | – | – | – | 0.56*** |
Dummy variables | ||||
VCH (2003:Q3 onwards) | 0.03** | 0.11*** | 0.06** | −0.13*** |
VCH (2001:Q4) | 0.08*** | 0.12*** | 0.10*** | 0.04*** |
VCH (2002:Q1) | 0.04*** | 0.03*** | 0.06*** | – |
GFC (2008:Q4) | 0.07*** | – | 0.12*** | 0.03*** |
GFC (2009:Q1) | 0.02** | – | 0.03** | – |
GFC (2009:Q2) | – | – | – | – |
Adjusted R2(a) | 0.50 | 0.37 | 0.49 | 0.68 |
Standard error | 0.0076 | 0.0094 | 0.012 | 0.0064 |
LM(5) test(b) | 0.12 | 0.03 | 0.58 | 0.13 |
Chow test (midpoint)(b) | 0.95 | 0.17 | 0.94 | 0.54 |
Notes: ***, ** and * indicate significance at the 1, 5 and 10 per cent level,
respectively; ‘–’ indicates the variable was not significant
and removed from estimation (a) Not including single quarter dummy variables (b) p-value of F-statistic reported |
The estimated interest rate coefficients are broadly consistent with the theory that demand for larger denominations should be relatively more sensitive to deposit rates. The insignificance of the GFC dummy variables in the low-denomination regression confirms that only larger denominations were behaving unusually in this period. The larger coefficients on the GFC dummies for the $50 banknote regression compared to the $100 banknote regression mirrors the larger rise in $50 banknotes seen at the time.
The rise in high denominations (particularly the $100 banknotes) is consistent with some individuals converting their deposits into currency for precautionary purposes as the events of the global financial crisis made them more apprehensive about holding deposits. The rise in deposits overall, however, seems to suggest that others may have increased their holdings of deposits by substituting out of relatively more risky assets such as trusts or mortgaged-backed securities.
Footnotes
In Australia, M1 is the sum of currency plus bank current deposits of the private non-bank sector. [12]
Full details of all data series and sources are given in Appendix A. [13]
Analysing currency on issue, which includes banknotes and coins, makes no difference to the results. [14]
The weighted average deposit rate is highly correlated with the cash rate, especially in the pre-GFC sample. Using the cash rate instead has little effect on most of the modelling results. [15]
The financial crisis variables are omitted at this stage, but are included in Section 4.4.2. [16]
Excluding the self-employment variable has no major bearing on other coefficients. [17]
ATMs and ETPOS terminals per capita increased on average by 9 and 21 per cent per year respectively between 1993 and 2008. Bank branches per capita have decreased by an average of 3 per cent per year over the same period. [18]
The stimulus payments totalled around 1.7 per cent of annual GDP, but this cannot be directly translated to an increase in transactional demand because the payments were temporary and households could choose to save or spend them. [19]
Given that bank deposits also rose, this could be interpreted as a general increase in demand for less risky assets. However, in estimations of a similar model with household deposits as the dependent variable, GFC dummy variables are not significant (compared with the highly significant dummies for non-bank currency holdings in Table 2). This suggests that the rise in currency holdings in late 2008–early 2009 was more unusual than the rise in deposits. [20]
Twenty dollar banknotes are omitted due to their changing role over time. In regressions with twenty dollar banknotes, all explanatory variables except EFTPOS terminals are insignificant. [21]
The coefficient on the dummy variable for Y2K in the December quarter 1999 is insignificant because the effect is muted by averaging across the quarter. [22]