RDP 2012-06: The Impact of Payment System Design on Tiering Incentives 5. The Impact of Tiering on Liquidity Usage
October 2012 – ISSN 1320-7229 (Print), ISSN 1448-5109 (Online)
- Download the Paper 862KB
5.1 Estimates of Liquidity Savings
We present simulation results for the changes in liquidity use due to increased tiering in the four system designs. Our hypothesis is that the liquidity benefits from tiering decrease as more liquidity-saving features are added to the RTGS system. A decomposition of liquidity savings into the two sources identified in the literature, namely liquidity pooling and payments internalisation, is contained in Appendix B.
5.1.1 Cumulative tiering
We first look at the case where individual institutions are tiered cumulatively, from smallest to largest, according to their share of the total value of payments. Figure 1 shows liquidity usage over the range from no tiering to tiering all candidate institutions. For all scenarios in this case, the pure RTGS system is the most liquidity intensive and the RITS replica the least intensive. Of the two other system designs, the bilateral-offset system clearly uses less liquidity for the first 33 tiering scenarios. For subsequent scenarios, however, the presence of bilateral offset has almost no effect. This may be due to the increasing concentration of the system; Ercevik and Jackson (2009) report the intuitive finding that liquidity recycling increases with system concentration, thus the need for bilateral offset decreases. The share of the total value of payments settled by bilateral offset falls from 28 per cent when there is no tiering to 13 per cent when all candidate institutions are tiered.
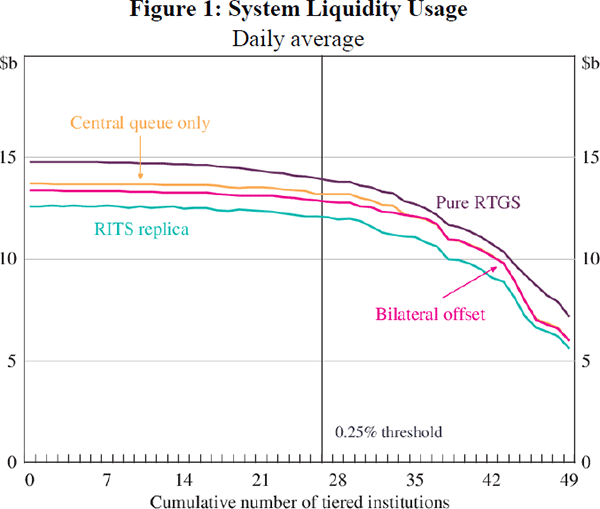
In line with our hypothesis, liquidity-saving mechanisms typically reduce the liquidity benefits from tiering. Average daily liquidity usage falls by $7.0 billion when all candidates are tiered in the RITS replica system, compared with larger decreases of: $7.4 billion in the system with bilateral offset; $7.6 billion in the pure RTGS system; and $7.7 billion in the system with a central queue only. However, there is some variance in the results, with this ranking not holding at all increments of the cumulative tiering.[16]
5.1.2 Tiering individual participants
We now look at the case where individual participants are tiered in isolation. Again, the pure RTGS system is the most liquidity intensive and the RITS replica the least intensive for all scenarios. Average daily system liquidity usage falls by $137 million on average when a single institution is tiered in the RITS replica system, compared with larger decreases of: $143 million in the system with a central queue only; $151 million in the pure RTGS system; and $155 million in the system with bilateral offset. While liquidity savings are lowest in the RITS replica system as expected under our hypothesis, the fact that savings are highest in the bilateral-offset system is not consistent with our hypothesis. Again, there is variance in the results, with this ranking not necessarily holding for each individual institution tiered.
5.1.3 Network effects
It is possible that the low level of tiering observed currently in RITS might be because the benefits of tiering are dependent on the size of the tiered network. For instance, the proportion of payments that can be internalised for a given tiering candidate will tend to increase the larger is the tiered network being joined. If these network effects are large, then multiple equilibria including both high and low degrees of tiering would be conceivable (with the latter a result of very few institutions considering it worthwhile to tier as long as very few other institutions are already tiered).
By comparing the liquidity savings in the cumulative and individual tiering scenarios, we find some evidence for this possibility. When the smallest 30 institutions are tiered in the RITS replica system, average daily liquidity usage declines by around $721 million; whereas when each of the smallest 30 institutions are tiered individually, the summed marginal changes imply a decrease in average daily liquidity usage of around $485 million. That is, the network effect leads to an additional $236 million in total liquidity savings. However, this estimate of the size of the network effects varies; if we considered tiering 35 institutions, for instance, then network effects would appear to imply only $171 million of total liquidity savings. In addition, on average an institution in the smallest 30 sends and receives just 8 per cent of the total value of its payments to and from other institutions in the smallest 30, suggesting that the scope for network effects in this group is limited.
Footnote
For the systems with credit limits, we find that the marginal change in liquidity usage from tiering an additional institution is often not significantly different from zero at the 10 per cent level for approximately the smallest 30 institutions. [16]