Bulletin – April 2025 Australian Economy Monetary Policy Transmission through the Lens of the RBAs Models
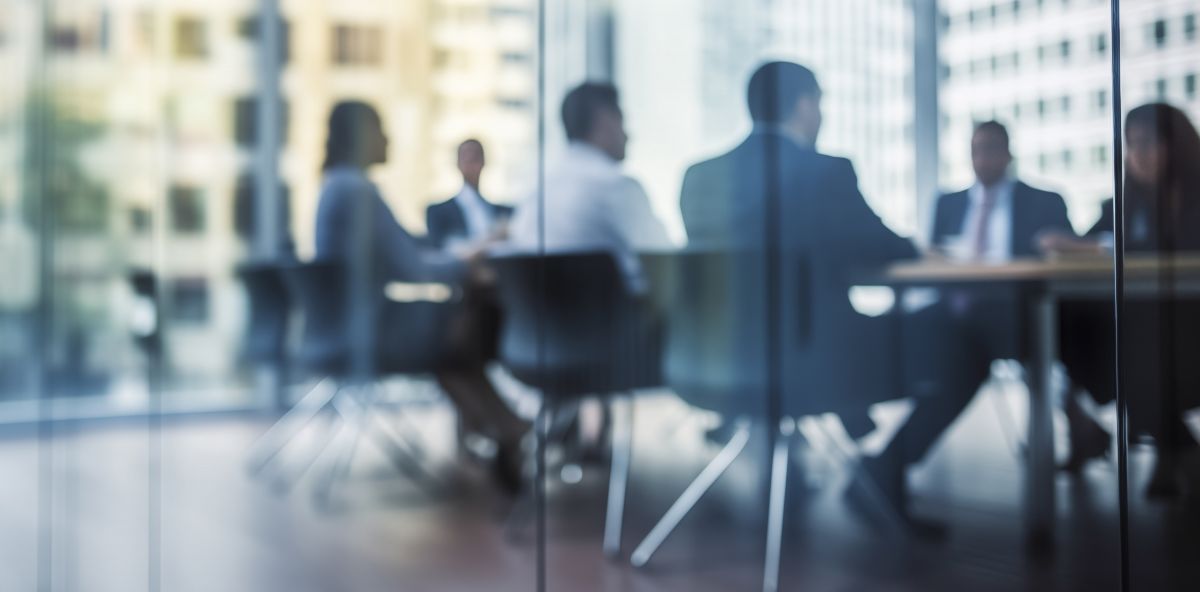
Abstract
Understanding how changes in the cash rate affect economic activity and inflation – so-called monetary policy transmission – is important for the RBA in pursuing its objectives of price stability and full employment. This article explains how the RBA uses its core models of the Australian economy to estimate the overall effects of policy, explore the different channels through which monetary policy transmits, and consider the economic outlook under alternative paths for monetary policy. The findings highlight that: the peak effect of policy is likely to occur after around one to two years; the exchange rate acts as an important transmission channel for policy; housing is a sensitive part of economic activity; and although individual households cashflow can be sensitive to changes in the cash rate, in aggregate it plays a smaller role in transmission.
Introduction
The new Monetary Policy Board (and previously the Reserve Bank Board) sets monetary policy to achieve low and stable inflation and full employment. In doing so, the Board considers information from the RBA staff about current and future economic conditions, along with analysis about how different policy options might affect the economy. For the RBA staff to provide this advice, it is important to understand how much a given change in interest rates will influence economic activity and inflation. It is also important for the RBA to understand the various channels through which monetary policy transmits to economic outcomes, how important each is, and what parts of the economy they affect. This allows the RBA to better monitor and communicate the effects of policy on peoples lives, and to assess whether these effects could change over time as the economy changes.
An RBA Explainer describes monetary policy transmission as having two stages:
- changes in monetary policy affect interest rates in the economy
- changes in interest rates affect economic activity and inflation (RBA 2025).
The RBA uses various models to understand the transmission of policy. This is because different models make different assumptions about how the economy works, which lead to different conclusions about the effects of policy on economic activity and inflation. This diversity supports more robust policymaking.
In this article, we first start by providing an overview of a few models that the RBA considers, and their predictions of how changes in monetary policy affect inflation and economic activity. We then focus on the RBAs two main macroeconomic models – its macroeconometric model MARTIN, and its dynamic stochastic general equilibrium (DSGE) model DINGO.1 These models assume that the first stage of transmission from the cash rate into short-term interest rates faced by households and firms is roughly one-to-one, and so we focus on the second stage of transmission in this article, highlighting which channels they suggest are more and less prominent. We then conclude by showing how these models can be used to assess alternative paths for monetary policy and what they would mean for the economy. This can help the Board to consider what different policy choices might imply for the outlook.
Consistent with previous work, we find that different models have somewhat different predictions for the overall effects of policy, but that the effects on GDP and inflation tend to peak around one to two years after policy changes. We also show that across both MARTIN and DINGO, the exchange rate channel tends to be very important – especially for inflation – while the cash-flow channel is less important in aggregate, despite it having an obvious effect on individual households through changes in mortgage repayments.
How much does monetary policy affect the economy?
There are a range of ways to model the economy and they all make different assumptions about how the economy works and how we can learn about economic relationships from data. Broadly speaking, models vary in how much they rely on economic theory or simply take the observed historical relationships between variables as given. On one end of the spectrum are models known as vector autoregressions (VARs), such as those used in Beckers (2020) and Read (2023).2 These models primarily aim to capture observed relationships in the data and make fewer and/or weaker assumptions about how the economy works. They are very flexible and data-driven, but may not provide much insight into the underlying structure of how monetary policy transmits to the economy. On the other hand, DSGE models rely heavily on economic theory to specify how individual people and businesses make decisions that determine overall economic activity. These models tend to assume people think about both the future and the past when making these choices. Somewhere between these two are semi-structural models like the RBAs MARTIN.3 These models draw on theory to specify economic relationships that are assumed to hold in the long run, while being more flexible in capturing patterns in the data in the short run. Semi-structural models can capture whether people make decisions based on the past or the future, but they tend to capture decision-making at the aggregate level, rather than embedding it in the model using economic theory of individual agents, as in DSGE models. All of these classes of models are commonly used in central banks around the world, and are often used to complement each other for different purposes.
Different model structures produce different predictions for how much the economy responds to changes in monetary policy. This is commonly illustrated by the impulse response function (IRF) of a model, which shows the response of economic variables over time to a change in the cash rate. Graph 1 and Graph 2 show the responses of real GDP and year-ended inflation to a 100 basis point increase in the cash rate across a range of models. We use three different RBA models that span the different classes, and include an external benchmark labelled Murphy.4 We can see that most models estimate the peak impact of policy occurs after around one to two years. But the estimates of the peak effect range from ¼ to 1 per cent for GDP, and ⅛ to ½ percentage points for inflation. Since these results come from models that are estimated from the data, there is also uncertainty around each models IRF that is not shown on the graphs. The considerable differences in responses across models emphasises the importance of maintaining and using a suite of models when deliberating the effect of a change in monetary policy.
In trying to understand some of these differences, it is important to note that in DINGO people tend to make decisions based on what they expect to happen in the future, whereas in MARTIN expectations are not modelled in such an explicit way.5 So changes in the cash rate pass through to the economy quicker in DINGO and this is likely because people react to the fact that rates will be higher for a period into the future – the entire future path of interest rates matters. But the path of interest rates matters even beyond the role of expectations; each model predicts that the cash rate evolves differently following the initial increase.6 This can be seen in Graph 3, which shows the path of the cash rate in the years after the initial increase in monetary policy. Some models assume rates return to normal slowly, while others assume a much quicker return.
These dynamics play an important role in the response of the economy to monetary policy in the models, which can make it hard to interpret differences in the overall effects. For example, on face value it looks like MARTIN implies a relatively small effect of policy on inflation outcomes. But this largely reflects the fact that MARTIN predicts the cash rate would fall more quickly and even drop below its original level before stabilising. A way to resolve this issue is to conduct policy simulations using the same path for policy (rather than the same initial increase). This is how we conduct policy simulations at the RBA – we provide an example in the section below on What does this mean for monetary policy?.
Decomposing the channels, according to MARTIN and DINGO
RBA (2025) presents a common way of decomposing the transmission of monetary policy into four key channels:
- The exchange rate channel: an increase in interest rates increases the return on Australian assets, and so foreign demand for the Australian dollar to buy them, pushing up the exchange rate. A higher exchange rate means imported goods are cheaper, weighing on inflation. It also makes imports more competitive, and exports less competitive, leading to lower net exports and weaker growth.
- Asset prices and wealth: an increase in interest rates tends to weigh on asset prices. This means that people and businesses will have less equity to use to borrow, and household wealth will decline, which may make them less willing to spend or invest. As a result, lower demand weighs on economic activity.
- Savings and investment: an increase in interest rates raises the return people earn on their savings and increases the cost of borrowing. As such they will tend to save more, and invest and consume less.
- Cash flow channel: higher interest rates mean borrowers pay more to service their debt, and savers earn more on their deposits. The two effects partially offset, but household debt exceeds deposits so on net higher interest rates reduce household cashflow; the resulting decrease in demand is amplified by different spending behaviour across borrowers and savers.
However, there are other ways to think about the transmission of policy to the economy.7 RBA (2025) uses the same channel framework as Atkin and La Cava (2017), whereas Kent (2023) identifies a fifth channel – the credit channel – that emphasises how changes in monetary policy can affect the supply of credit provided by the financial sector. While taxonomies differ, we proceed with the four channels discussed in this article, since they are better suited to the models we are considering.8
As discussed above, we can use the MARTIN and DINGO models to help quantify the channels through which monetary policy transmits to the economy. Nevertheless, such an exercise is challenging, in large part because the transmission channels often overlap or interact. For example, if rates rise asset prices will tend to fall, decreasing household spending via a wealth effect. At the same time, higher interest rates also make households less credit worthy – directly due to higher repayments and indirectly due to lower asset prices reducing net worth of some households – limiting their access to credit and further lowering their consumption. In this case, it is not obvious by how much the asset price channel or the credit channel decreased household consumption. These difficulties are reinforced by second round effects, where the overall weaker economy leads to lower incomes, and so consumption. Although identifying specific channels is challenging, models can still be used to get some sense of which mechanisms might be most important for the transmission of monetary policy.
One simple way to understand the transmission channels is to look at how the expenditure components of GDP evolve after a change in monetary policy (Graph 4 for MARTINs decomposition; Graph 5 for DINGOs).9 This exercise shows that net trade explains most of the decline in GDP over the first year following an increase in the cash rate in MARTIN, which suggests that the exchange rate channel is likely to be important. Imports are predicted to rise in the short term (weighing on GDP) due to a strong response to lower import prices. By contrast, imports fall following an increase in interest rates in DINGO (supporting GDP) as weaker overall demand more than offsets the fact that imports are now cheaper. This exercise shows a clear tension between the models, and highlights the benefits of using a suite of models with different assumptions in trying to get a range of predictions and support robust policymaking.10
In both models, household consumption responds slowly, but accounts for a sizeable share of the change in GDP and shows quite a persistent decline. The strong but delayed response suggests that indirect channels may play an important role in the consumption effect, rather than a change in household cash flows that affects consumption relatively quickly (La Cava, Kaplan and Hughson 2016). The asset prices and wealth channel is likely to be important, where the higher cash rate flows through to household balance sheets via falling housing and equity prices, which decreases net worth and consumption. Dwelling investment and business investment also play an important role in both models, with the former being relatively more important in MARTIN. The strong response of investment suggests that the savings and investment channel is important; however, the asset price and wealth channel may also play a role here, particularly if dwelling investment responds to a fall in house prices.
While this simple exercise reveals several important features about the Australian economy, an alternative is to try to directly isolate each of the channels in the models. To do this, we turn off the response of certain variables in the models to approximately isolate one channel at a time.11 We discuss the results for each channel below, but our key takeaways are:12
- According to MARTIN, the cash flow channel plays a relatively small role in how monetary policy affects the economy (Graph 6; Graph 7). This is consistent with the small initial decline in consumption noted above.
- Instead, the exchange rate is an important channel of monetary policy, particularly for inflation (Graph 7). This is consistent with the strong trade response discussed above.
- The other channels are also important, but are harder to isolate in the models.
Exchange rate channel
For the exchange rate response, the important feature of the change in monetary policy is that it changes the cash rate relative to interest rates in other economies. If other economies were to change monetary policy in tandem with Australia, the observed effect on the exchange rate would be very small. For example, during much of the 2022–2023 tightening cycle, the real trade-weighted index was quite stable because interest rate increases were also occurring across many other countries at the same time. It is important to emphasise that the exchange rate channel was still operating at this time – it is very likely the Australian dollar would have depreciated if the cash rate had remained lower.
At the peak of the GDP response, the exchange rate channel accounts for around one-quarter to two-thirds of the GDP response in DINGO and MARTIN, and around one-third to two-thirds of the response of inflation. While MARTIN and DINGO agree that the exchange rate is an important channel of monetary policy transmission, this exercise shows that it is particularly important in MARTIN.
Asset prices and savings/investment channel
For the asset prices channel and the savings and investment channel, the important feature of the change in monetary policy is that it changes the relative attractiveness of saving versus spending. That is because the interest rate tells us how much savings income we have to forgo in a years time so that we can spend money now. Economists call the decision to change how much we consume or save now versus the future intertemporal substitution. This feature of interest rates provides the underlying structural driver of both the asset prices channel and the savings and investment channel. Households and firms savings and investment decisions are directly affected by interest rates, whereas the asset price channel can be thought of as the indirect effect of these same decisions. That is, asset prices adjust to bring the expected rate of return on the asset into line with interest rates, with further adjustments in decisions resulting from this price response. The indirect effect relies on additional behaviours, such as the tendency for households to consume more when their wealth increases.13
The asset prices channel and savings and investment channel also overlap significantly in MARTIN, and so it is difficult to quantify the channels separately. As such, we group these two channels together. According to MARTIN, the asset prices channel and the savings and investment channel account for about one-quarter of the peak GDP response but account for a larger share later on (Graph 6). As discussed above, these channels are likely to be particularly important for business and dwelling investment, and consumption; however, the role of dwelling investment stands out. An alternative way of isolating the housing price and dwelling investment effect suggests it accounts for a little less than one-quarter of the GDP response in MARTIN, and most of the asset prices channel and the savings and investment channel. The housing sector also notably increases the duration of the GDP response to monetary policy in DINGO (Gibbs, Hambur and Nodari 2018). The smaller response of business investment in MARTIN is consistent with businesses having high and sticky investment hurdle rates (Lane and Rosewall 2015). However, Nolan, Hambur and Vermeulen (2023) find that despite high and sticky hurdle rates, changes in interest rates still affect business investment decisions.
Cash flow and other channels
The cash flow channel captures how changes in interest rates flow through to households interest income and debt repayments. As this is the most obvious effect of monetary policy for households to observe, it often gains considerable public attention. The strength of this channel will be a function of the relative size of households holdings of interest-sensitive assets and debt, and how different these households consumption responses are to a change in their income. So the distribution of assets and liabilities across households with different characteristics is an important factor in the transmission of monetary policy.
However, MARTIN does not directly model the distribution of households and only captures the aggregate effect of changes in interest income and repayments. In addition, it is very difficult to isolate just the cash flow channel in MARTIN since household income is affected by many factors. So we label the rest of the effect left over after accounting for the exchange rate, asset prices and savings/investment channels as the cash flow and other channels.
MARTIN suggests that the cash flow and other channels are small, but occur faster than the savings and investment and the asset prices channels. Most of this channels contribution to GDP comes through consumption, which decreases by about 0.15 per cent. In MARTIN, the decrease in consumption largely reflects lower non-labour income, which is consistent with a fall in interest-sensitive cash flows.
Still, MARTINs estimates of the cash flow channel are broadly similar to other estimates of the size of the cash flow channel. For example, La Cava, Hughson and Kaplan (2016) found that a 100 basis point increase in the cash rate for one year leads to a 0.1–0.2 per cent fall in aggregate household expenditure.14 Additionally, updated RBA staff estimates suggest that the cash flow effect is unlikely to have changed substantially of late (Jennison and Miller 2025). One reason why we might see a small overall effect on the economy is that the cash flow channel works in opposite directions depending on whether the household is a net saver or a borrower. While aggregate cash flows for these two groups could be large, they partially offset each other and so the net economic effect is smaller.
What does this mean for monetary policy?
How do these results affect the way the RBA staff think about monetary policy? Since the models build in the relationships between important economic variables and the cash rate and how long they take to transmit to the economy, we often use them to examine how different alternative policy settings affect the outlook. This helps the Board evaluate how different policy options fit into their broader monetary policy strategy and communicate how policy settings might need to respond if the economic outlook unfolds differently to the RBAs central forecast (Hunter 2024). This is particularly important given the long and variable lags of monetary policy, which means that policymaking needs to be forward-looking and consider the future path of interest rates, rather than meeting-by-meeting decisions (Hunter 2025).
We provide an illustrative example of this below, which compares how an alternative path of the cash rate affects the outlook for GDP and inflation. The baseline path – called the February market path – represents how financial markets expected the cash rate would evolve as of February 2025 (Graph 8). The RBA uses the prevailing market path to create the forecasts released every quarter in the Statement on Monetary Policy, and so it provides a useful benchmark. An alternative hold path assumes the cash rate remained at 4.35 per cent from the February Board meeting until the end of 2026. An important question for the Board is how this different path for the cash rate might affect the outlook, which can be answered using MARTIN and DINGO.
As published in the February Statement, following the February market path saw GDP grow by about 2¼ per cent on average per year from the beginning of 2025 to the end of 2026. The growth in GDP over the forecasts broadly matched the RBAs assessment of growth in economic capacity. Therefore, the level of demand continued to modestly exceed supply. As a result of the mismatch between demand and supply, inflation was expected to remain above the midpoint of the 2–3 per cent target range at about 2.7 per cent at the end of 2026. However, under the hold path, the models predict GDP would grow by about 1½ per cent on average per year from the start of 2025 to the end of 2026.15 This results in demand and supply being more balanced. As a result, inflation decreases to be around or below the midpoint of the RBAs 2–3 per cent target range by the end of 2026.
The results from the two models under the hold path are moderately different. The lower bound estimates suggest that underlying inflation would fall below the middle of the target band by 2026. However, the undershoot is small, providing some comfort that such a path would not lead to a significant fall in inflation. This highlights how using a range of models can provide a more robust view of different policy alternatives. This illustrative example applies to the February Statement, and so does not incorporate any information received since then about the economic outlook.
Conclusion
Understanding the pass-through of monetary policy to economic activity and inflation is crucial for the RBA in achieving its policy objectives of price stability and full employment. Models enhance our understanding by providing estimates of the aggregate effects of policy, allowing us to explore transmission channels, and allowing us to consider the economic outlook under alternative policy paths. By mapping out various transmission channels, we have highlighted some key considerations for understanding the effects of monetary policy.
The RBAs core models, MARTIN and DINGO, are primarily used for these exercises. The models highlight that the peak effect of policy is likely to occur after around one to two years. They also show that the exchange rate acts as a core transmission channel for policy, while housing is a sensitive part of economic activity. Although the cash flow channel gains a lot of public attention and can have a large effect on individual households, it has a smaller role in aggregate transmission.
While useful for policy purposes, both models have shortcomings and make specific assumptions. At times, the models can provide conflicting predictions. As such, the RBA is continuing to invest in improving these models, as well as exploring new models such as Heterogenous Agent New Keynesian (HANK) models that can better account for the diversity of financial positions across households. This will allow the RBA to continue to build a richer understanding of the effects of monetary policy, and therefore support policymaking.
Appendix A: Tempering monetary policy expectations in DINGO
In the Banks DSGE model, decisions by households and firms depend on not just the current level of the cash rate, but also on what they expect the path of the cash rate to be in the future. Therefore, the effect of a change in the path of the cash rate depends on how much people anticipate this change.
We can use the alternative hold path from Graph 8 as an example. At one extreme, suppose that all households and firms fully anticipate the Board to implement the hold path. This means that changes expected to occur even far in the future, say near the end of 2026, have potentially powerful effects on current decisions (and therefore on output and inflation).16 But this may be an unrealistic assumption: households might be somewhat uncertain about whether the Board would hold rates higher to the end of 2026.
At the other extreme, we can assume that all future changes in the cash rate are completely unanticipated. This means that households and firms would expect the interest rate to revert and keep being surprised that rates had stayed where they were. As a result, households would not adjust their behaviour as much. However, this extreme is also unlikely. If policymakers chose an alternative policy path like the hold path, they would likely seek to communicate it to the public, rather than repeatedly surprising them as they acted that path out.
When modelling the effect of alternative cash rate paths, we assume something in the middle. We assume that, at any point in time, the future shocks generating the constant cash rate path are partly anticipated, with households placing less and less weight on those further into the future. Specifically, we assume that they put less weight and focus on a future shock set to occur in quarters, giving it only a weight of for some discount parameter that is less than one.17 The rationale is that changes in the near future (e.g. next quarter) are likely anticipated by more people than changes in the distant future (e.g. in 10 years).18
We set
Endnotes
Jack Mulqueeney and Alexander Ballantyne are from Economic Analysis Department and Jonathan Hambur is from Economic Research Department. The authors would like to thank Meredith Beechey Österholm, Anthony Brassil, Irene Cam, Matt Read, Callum Ryan, Tim Taylor and Nick West for their comments and contribution to the analysis, and Michaela Haderer and Callum Ryan for their work on tempering the expectations dynamics in the DSGE model (explained in Appendix A). *
MARTIN is detailed in Cusbert and Kendall (2018) and Ballantyne et al (2019). DINGO, or the Dynamic Intertemporal New-Keynesian General-Equilibrium Optimisation model, is detailed in Gibbs, Hambur and Nodari (2018), which builds on Rees, Smith and Hall (2015). 1
A range of papers have used VARs to estimate the effects of monetary policy, often with additional structure intended to aid identification. Read (2023) explores a set of relatively weak assumptions to identify the effects of monetary policy using a structural VAR; the estimates are difficult to compare against the other models for methodological reasons, but the results are consistent with the output response lying towards the upper end of the range of existing estimates. Hartigan and Morley (2020) combine information from a large number of economic variables to estimate a factor-augmented VAR and find that the transmission of monetary policy appears to have changed since the introduction of inflation targeting. Dungey and Pagan (2009) use a structural VAR that also accounts for long-run relationships between economic variables (cointegration) and find that the response to monetary policy is smaller than in a model that does not account for these long-run relationships. 2
These types of models have a long history in Australia and globally and continue to be a focus of active development across academia and policy institutions. Some examples of models that have been used in Australian policy institutions are Treasurys EMMA model (see Bullen et al 2021) and former TRYM model, Outlook Economics AUS-M, and Oxford Economics Global Economic Model. See Pagan (2019) for a full history. 3
For the external benchmark, we use a model built by Chris Murphy (ANU). His model takes a hybrid approach, which means its structure lies somewhere in between MARTIN and DINGO. See Murphy (2020) and Murphy (2024). 4
MARTIN features long-term inflation expectations in the model, which is estimated from a range of financial market and survey data, but changes in interest rates do not affect these expectations in the model. The future path of interest rates is modelled through two- and 10-year government bond yields, which respond to the cash rate contemporaneously. 5
This reflects differences in how the models assume the RBA will respond to changes in economic conditions (i.e. inflation, unemployment and GDP) and the inertia inherent in setting policy, often referred to as the central banks reaction function. 6
The categorisation and emphasis of different channels varies across research on monetary policy transmission. See also Mishkin (1996), Ireland (2006) and Burr and Willems (2024). 7
We choose not to focus on the credit channel because the models used in this article do not have well developed financial sectors. However, Brassil, Major and Rickards (2022) develop a banking-augmented version of MARTIN and show how this affects monetary policy transmission, including showing how the overall size of effects can vary depending on the state of the economy. 8
For all following MARTIN and DSGE exercises, we assume public demand does not respond to the increase in the cash rate. 9
The difference is discussed in Ballantyne et al (2019). Some other Australian models also predict that imports fall following an increase in the interest (for a structural VAR example, see Lawson and Rees 2008). The difference between MARTIN and DINGO does not appear to reflect the choice between a DSGE and semi-structural model. For example, ECB-BASE, a semi-structural model of the Euro area, also predicts that imports fall (Angelini et al 2019). 10
This is implemented by forcing the exchange rate to remain at baseline throughout the exercise, while keeping the path of the cash rate the same as in the initial monetary policy shock exercise. In the DSGE we assume people expect the exchange rate to remain unchanged. In MARTIN, we then run a further exercise to further force house prices, dwelling investment, the earnings yield on equities and the business lending rate to remain at baseline to separate the asset prices and savings/investment channel. 11
Note that we only focus on the exchange rate channel for DINGO. This reflects the fact that the other channels are more interlinked and so harder to turn-off individually in DINGO. 12
This behaviour is consistent with the life-cycle theory of consumption (see Ando and Modigliani 1963) and is found as a feature of Australian households in empirical research (see May, Nodari and Rees 2020; Gillitzer and Wang 2016); although, the observed behaviour may also be due to interactions with credit (see Windsor, Jääskelä and Finlay 2015). 13
They construct this estimate using household-level data on interest-sensitive cash flows and estimated marginal propensities to consume. They find the response entirely reflects changes in durables consumption. Non-durables consumption does not respond to changes in interest-sensitive cash flows. 14
The magnitude of the decrease in GDP growth and subsequent fall in inflation depends on the degree to which people expect interest rates to be higher in the future. See Appendix A for more details about how we constructed this range of estimates. 15
This is a well-known feature of full-information rational-expectations models; it is known as the forward guidance puzzle (see e.g. Del Negro, Giannoni and Patterson 2023). 16
This approach is one of several suggested in
Groot et al (2021). The discounting of future shocks by
In addition to the shocks potentially being
anticipated (at least for
Our approach is similar to the modelling approach taken by some other central banks. For example, in an evaluation of policy strategies, Federal Reserve staff discounted expectations terms in their DSGE model by multiplying them by 0.9 or 0.95 (Arias et al 2020). 19
References
Ando A and F Modigliani (1963), The “Life Cycle” Hypothesis of Saving: Aggregate Implications and Tests, American Economic Review, 53(1), pp 55–84.
Angeletos G-M and C Lian (2018), Forward Guidance without Common Knowledge, American Economic Review, 108(9), pp 2477–2512.
Angelini E, N Bokan, K Christoffel, M Ciccarelli and S Zimic (2019), Introducing ECB-BASE: The Blueprint of the New ECB Semi-structural Model for the Euro Area, ECB Working Paper Series No 2315.
Arias J, M Bodenstein, H Chung, T Drautzburg and A Raffo (2020), Alternative Strategies: How Do They Work? How Might They Help?, Federal Reserve Board Finance and Economics Discussion Series No 2020-068.
Atkin T and G La Cava (2017), The Transmission of Monetary Policy: How Does It Work?, RBA Bulletin, September.
Ballantyne A, T Cusbert, R Evans, R Guttmann, J Hambur, A Hamilton, E Kendall, R McCririck, G Nodari and D Rees (2019), MARTIN Has Its Place: A Macroeconometric Model of the Australian Economy, RBA Research Discussion Paper No 2019-07.
Beckers B (2020), Credit Spreads, Monetary Policy and the Price Puzzle, RBA Research Discussion Paper No 2020-01.
Brassil A, M Major and P Rickards (2022), MARTIN Gets a Bank Account: Adding a Banking Sector to the RBAs Macroeconometric Model, RBA Research Discussion Paper No 2022-01.
Bullen J, B Conigrave, A Elderfield, C Karmel, L Lucas, C Murphy, H Ruberl, N Stoney and H Yao (2021), The Treasury Macroeconometric Model of Australia: Modelling Approach, Treasury Paper No 2021-09.
Burr N and T Willems (2024), About a Rate of (General) Interest: How Monetary Policy Transmits, BoE Quarterly Bulletin, July.
Cusbert T and E Kendall (2018), Meet MARTIN, the RBAs New Macroeconomic Model, RBA Bulletin, March.
Del Negro M, MP Giannoni and C Patterson (2023), The Forward Guidance Puzzle, Journal of Political Economy Macroeconomics, 1(1), pp 43–79.
Dungey M and A Pagan (2009), Extending a SVAR Model of the Australian Economy, Economic Record, 85(268), pp 1–20.
Farhi E and I Werning (2019), Monetary Policy, Bounded Rationality, and Incomplete Markets, American Economic Review, 109(11), pp 3887–3928.
Gabaix X (2020), A Behavioral New Keynesian Model, American Economic Review, 110(8), pp 2271–2327.
García-Schmidt M and M Woodford (2019), Are Low Interest Rates Deflationary? A Paradox of Perfect-Foresight Analysis, American Economic Review, 109(1), pp 86–120.
Gibbs C, J Hambur and G Nodari (2018), DSGE Reno: Adding a Housing Block to a Small Open Economy Model, RBA Research Discussion Paper No 2018-04.
Gillitzer C and JC Wang (2016), Housing Wealth Effects: Cross-sectional Evidence from New Vehicle Registrations, Economic Record, 92(S1), pp 30–51.
Groot O, F Mazelis, R Motto, A Ristiniemi and A Ristiniemi (2021), A Toolkit for Computing Constrained Optimal Policy Projections (COPPs), ECB Working Paper No 2021/2555.
Hartigan L and J Morley (2020), A Factor Model Analysis of the Australian Economy and the Effects of Inflation Targeting, Economic Record, 96(314), pp 271–293.
Hunter S (2024), Shedding Light on Uncertainty: Using Scenarios in Forecasting and Policy, Speech at the University of Adelaide South Australian Centre for Economics Studies (SACES) Lunch, Adelaide, 13 December.
Hunter S (2025), Monetary Policy: Forward Looking and Data Dependent in the Face of Uncertainty, The Australian Financial Review Banking Summit, Sydney, 18 March.
Ireland P (2006), The Monetary Transmission Mechanism, Federal Reserve Bank of Boston Working Paper No 06-1.
Jennison S and M Miller (2025), An Update on the Household Cash-flow Channel of Monetary Policy, RBA Bulletin, January.
Kent C (2023), Channels of Transmission, Address to Bloomberg, Sydney, 11 October.
La Cava G, H Hughson and G Kaplan (2016), The Household Cash Flow Channel of Monetary Policy, RBA Research Discussion Paper No 2016-12.
Lawson J and D Rees (2008), A Sectoral Model of the Australian Economy, RBA Research Discussion Paper No 2008-01.
Lane K and T Rosewall (2015), Firms Investment Decisions and Interest Rates, RBA Bulletin, June.
May D, G Nodari and D Rees (2020), Wealth and Consumption in Australia, Australian Economic Review, 53(1), pp 105–117.
McKay A, E Nakamura and J Steinsson (2016), The Power of Forward Guidance Revisited, American Economic Review, 106(10), pp 3133–3158.
Mishkin F (1996), The Channels of Monetary Transmission: Lessons for Monetary Policy, NBER Working Paper No 5464.
Murphy CW (2020), Decisions in Designing an Australian Macroeconomic Model, Economic Record, 96, pp 252–270.
Murphy CW (2024), An Evaluation of the Macro Policy Response to COVID, ANU Tax and Transfer Policy Institute Working Paper No 11/2024.
Nolan G, J Hambur and P Vermeulen (2023), Does Monetary Policy Affect Non-mining Business Investment in Australia? Evidence from BLADE, RBA Research Discussion Paper No 2023-09.
Pagan A (2019), Australian Macro-Econometric Models and Their Construction – A Short History, CAMA Working Paper No 50/2019.
RBA (Reserve Bank of Australia) (2025), The Transmission of Monetary Policy, Explainer.
Read M (2022), Estimating the Effects of Monetary Policy in Australia Using Sign-restricted Structural Vector Autoregressions, RBA Research Discussion Paper No 2022-09.
Rees D, P Smith and J Hall (2015), A Multi-sector Model of the Australian Economy, RBA Research Discussion Paper No 2015-07.
Windsor C, J Jääskelä and R Finlay (2015), Housing Wealth Effects: Evidence from an Australian Panel, Economica, 82(327), pp 552–577.
Underlying data for selected graphs. Other data may be available upon request via our general enquiry page.