Bulletin – April 2025 Australian Economy How Useful are Leading Labour Market Indicators at Forecasting the Unemployment Rate?
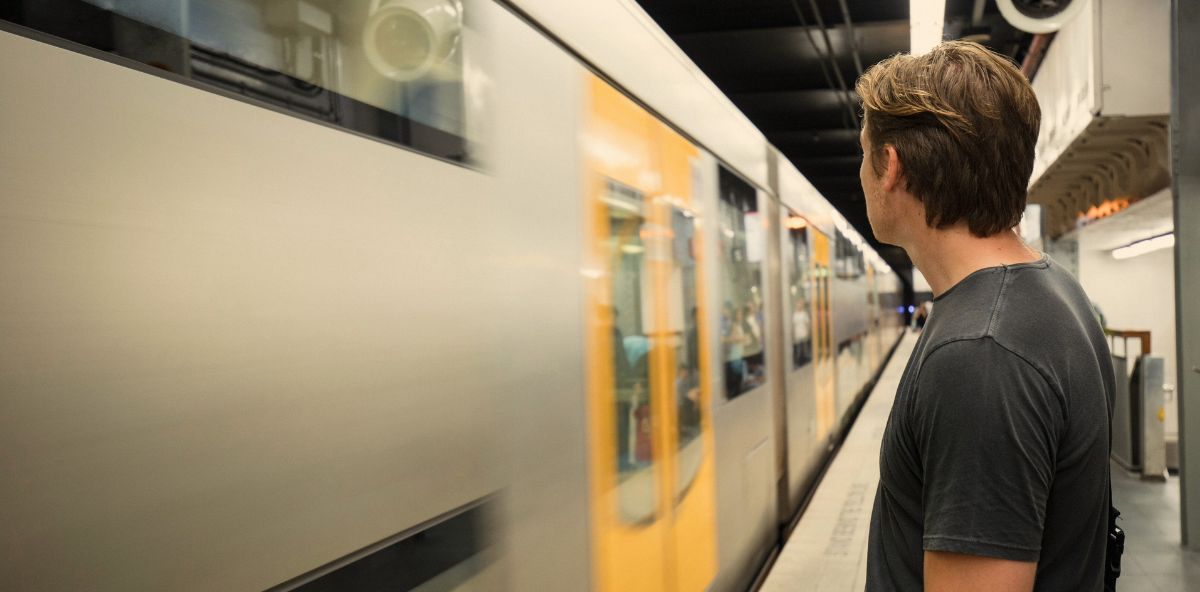
Abstract
The RBA draws on a wide range of information to form our assessment of current labour market conditions and our outlook for the labour market. One of the key labour market indicators that the RBA monitors and forecasts is the unemployment rate. This article considers whether information contained in indicators that are typically viewed as signalling a change in conditions before it becomes apparent in the official labour market statistics – referred to here as leading indicators – are helpful in forecasting the unemployment rate. It finds that information contained in measures of unmet demand, such as job advertisements and vacancies, and consumers expectations for unemployment are useful in informing the RBAs near-term forecasts for the unemployment rate. Models containing these leading indicators can complement our existing framework for forecasting the unemployment rate, which also considers information such as developments in economic activity, insights from firms in the RBAs liaison program and the experience of economies overseas.
Introduction
The unemployment rate plays an important role in Australian monetary policy, as it is relevant for both sides of the RBAs dual mandate. It is a key metric in our assessment of full employment, and forecasts for the unemployment rate are an important input into the inflation forecasts. That said, the unemployment rate is only one of many indicators that the RBA monitors when evaluating labour market conditions. In practice, our view of the labour market is formed by considering a number of other indicators (Ballantyne, Sharma and Taylor 2024). This includes indicators of labour demand that are typically considered to lead changes in aggregate employment and unemployment statistics (referred to here as leading indicators), flows of workers between states of the labour market (i.e. employment, unemployment and outside the labour force), broader measures of labour underutilisation and measures of capacity utilisation.
Changes in labour market conditions are typically first observable in various other indicators before they become apparent in the unemployment rate.1 For example, firms may respond to a pick-up in economic growth by increasing their demand for labour, which may cause them to post new job vacancies or recruit more intensely for existing vacancies, or increase the hours worked by their current workforce. Consumers may also react to improvements in economic conditions by becoming more optimistic about future labour market conditions and revising down their expectations for unemployment. We consider these indicators as having leading properties. Over time, as firms increase their rate of hiring, we may observe a greater flow of people into employment from unemployment or outside the labour force. When these flows become sufficiently large in magnitude, we are likely to observe a more evident decline in the unemployment rate and higher rates of employment growth.
This article considers the leading indicators the RBA currently monitors and then explores how useful they are at forecasting the unemployment rate. When forming forecasts for the unemployment rate, we also make use of both a suite of Okuns law models that uses information on economic growth and growth in real unit labour costs to predict the unemployment rate (Lancaster and Tulip 2015; Ballantyne et al 2019), as well as more qualitative information (such as from the RBAs liaison program). Further, we are developing a forecasting model that takes signal from labour market flows into and out of unemployment. These are all used to put together the unemployment rate forecasts that are published in the RBAs quarterly Statement on Monetary Policy.
What are the leading labour market indicators that the RBA monitors?
The RBA monitors a number of leading indicators including job advertisements and vacancies, consumers expectations for unemployment over the year ahead and firms hiring intentions. We look at a range of series because each indicator offers a partial view of the labour market and has its own limitations.
Job advertisements and vacancies capture firms unmet demand for labour and are indicative of the degree of tightness that exists in the labour market (Graph 1). When labour demand is stronger than supply, vacancies tend to rise because firms not only tend to post more vacancies but are also more likely to find it difficult to find workers to fill those vacancies. By contrast, those searching for work can find a job more readily in these circumstances and this will reduce the unemployment rate. Together, this leads to the observed negative correlation between the job vacancy and unemployment rates known as the Beveridge Curve (Graph 2).2 This relationship has historically been non-linear. When the labour market tightens, vacancies remain open for longer as firms find it increasingly more challenging to hire workers and fill vacancies, which then results in vacancies rising with only a small corresponding decline in unemployment.
Measures of job vacancies and advertisements can reflect either the stock of vacant positions or the flow of new job postings. The Australian Bureau of Statistics (ABS) measures the stock of job vacancies every three months, which captures all jobs that are ready to be filled immediately, are open to external applicants and for which some form of recruitment action (such as advertising) has been taken by the employer.3 This is therefore a broader measure of aggregate labour demand than other stock-based measures such as the ANZ–Indeed measure of job advertisements; for instance, the latter currently only captures online job postings on the Indeed and Workforce Australia websites, while the ABS measure would also capture recruitment through other channels such as jobs advertised on social media. However, the ANZ–Indeed job advertisement series, which is available monthly, is a timelier and higher frequency indicator compared with the ABS vacancies series.
We also monitor indicators that capture the flow of new online advertisements, such as the Jobs and Skills Australia (JSA) Internet Vacancy Index.4 This measure captures job advertisements newly lodged on the SEEK, CareerOne and Workforce Australia platforms. Flow-based measures of job advertisements can move ahead of stock-based measures and therefore signal a change in labour market conditions earlier. Moreover, they are more sensitive to recent changes in firms demand for labour because, unlike stock-based measures, they are not affected by withdrawals or the filling of longstanding vacancies. However, the JSA Internet Vacancy Index has the disadvantage of having a comparatively shorter back history (which begins in 2006).
The Westpac–Melbourne Institute (WMI) measure of consumers unemployment expectations provides an alternative view on the outlook for the unemployment rate (Graph 3). Unlike measures of job vacancies and advertisements, the WMI indicator does not directly capture information on employers hiring decisions but instead considers how households perceive future labour market conditions. A decline in the index suggests that more consumers expect that unemployment will decline over the year ahead.
Finally, we also consider information on employment intentions from the NAB business survey and the RBAs liaison program (Graph 4). These measures are forward looking and capture changes in firms hiring plans, with a strengthening in firm employment intentions potentially signalling that the unemployment rate could decline going forward. However, these indicators tend to better reflect developments in the market sector – that is, all industries except the health care, education and public administration industries, which are typically less sensitive to the business cycle. Thus, they likely provide an imperfect view of overall demand for labour (which would also capture demand from the non-market sector). Furthermore, some of these measures have relatively short back histories compared with indicators such as stock-based measures of job vacancies and advertisements and the WMI measure of unemployment expectations. They would therefore be less able to capture the underlying dynamics of the labour market during different business cycles over time.
Exploring the relationship between leading indicators and the unemployment rate
Movements in leading indicators can reflect momentum in the labour market and signal how the unemployment rate may evolve in the period ahead. To better understand how leading indicators and the unemployment rate co-move, I examined the correlation between the percentage change in the unemployment rate and the change in the relevant leading indicator. Specifically, I investigated the correlation between the percentage change in the unemployment rate and the percentage change in job vacancies and advertisements (as ratios to the labour force); this is so I could better account for the non-linear relationship between the unemployment rate and these leading indicators (described above). For all other leading indicators, I considered the correlation between the percentage point change in the indicators and the percentage change in the unemployment rate.
Given that no indicator provides a holistic view of the labour market, I also considered drawing together information contained in multiple leading indicators into a single metric called the summary leading indicator. In this article, I focus on a summary leading indicator constructed using information on the ABS measure of vacancies, ANZ–Indeed job advertisements and the WMI measure of unemployment expectations. These series have the longest back histories and therefore can offer more information about how the labour market behaves during different economic cycles.5 The summary leading indicator is calculated as a simple average of the standardised percentage changes in vacancies and job advertisements (as ratios to the labour force) and the percentage point change in the unemployment expectations index. It has historically co-moved and led movements in the unemployment rate (Graph 5).
The strongest correlations between these labour market indicators and the unemployment rate tend to be contemporaneous or leading by one quarter (Table 1). For all indicators, the strongest leading correlation occurs between changes in the indicator one quarter ago and changes in the unemployment rate today. However, the correlations at a lead length of two quarters are also reasonably strong for some indicators. The correlations suggest that the leading indicators may be useful for near-term forecasts of the unemployment rate.
Indicator leads changes in the unemployment rate | Indicator lags changes in the unemployment rate | ||||
---|---|---|---|---|---|
Quarters | 2 | 1 | 0 | 1 | 2 |
Full sample(b) | |||||
ABS vacancies | −0.39 | −0.40 | −0.47 | −0.10 | 0.00 |
ANZ–Indeed job advertisements | −0.44 | −0.57 | −0.69 | −0.22 | −0.08 |
JSA job advertisements | −0.38 | −0.53 | −0.64 | 0.07 | 0.07 |
WMI unemployment expectations | 0.21 | 0.30 | 0.18 | −0.11 | −0.24 |
NAB employment intentions | −0.31 | −0.50 | −0.14 | 0.23 | 0.32 |
Liaison employment intentions | −0.45 | −0.60 | −0.34 | 0.15 | 0.28 |
Summary leading indicator | −0.45 | −0.56 | −0.59 | −0.09 | 0.07 |
(a) I tested the correlation between the percentage change in the unemployment rate
and the percentage change in ABS vacancies, ANZ–Indeed job advertisements and JSA job
advertisements (as shares of the labour force), the percentage point change in the
WMI unemployment expectations measure and the NAB and liaison measures of employment
intentions, and the summary leading indicator (without any further
transformations). Bolded numbers refer to the strongest correlation coefficients for each indicator. Sources: ABS; ANZ–Indeed; Jobs and Skills Australia; NAB; RBA; Westpac–Melbourne Institute. |
Evaluating the forecasting performance of potential leading indicator models
To assess how useful leading indicators are at forecasting the unemployment rate, I assessed the forecasting performance of models containing these leading indicators. Each model includes up to two lagged values of a particular indicator given the results from the correlation analysis above. I conducted an out-of-sample forecasting exercise, which involves estimating a model using a subset of the available data, generating forecasts with the estimated model and then comparing those forecasts to the realised outcomes. This approach better captures how well the model would have performed in practice as it attempts to replicate the conditions and information a forecaster would have had at the time they needed to construct their forecasts.
For each potential leading indicator model, I generated a set of forecasts with a model estimated using the initial subset of data.6 I repeated this process, adding an additional quarter of data each time to the subset of data used to estimate the model, until all available data had been used. Finally, I evaluated the forecasting performance of these models. How accurately a model forecasts the unemployment rate is measured using its root mean squared errors (RMSEs), which capture the difference between the forecasts and the realised outcomes.7 Models with lower RMSEs are better at forecasting as their predictions are closer to the realised outcomes on average. I evaluated the performance of the forecasts over the pre-pandemic period to ensure the results were not distorted by pandemic-related volatility.
Comparing the RMSEs of models with different leading indicators can indicate which series are relatively more useful at forecasting the unemployment rate. As I considered multiple models with the same leading indicator but a varying number of lagged terms, I assessed the usefulness of an indicator by calculating the average forecast across models containing that leading indicator and then evaluating the accuracy of this average forecast. While I could have simply chosen the best-performing individual model, it is possible that individual models perform well by chance and hence looking at average performance is likely to offer more robust results. It is also useful to compare the models performance to that of a simple benchmark model, such as an autoregressive (AR) model of the percentage change in the unemployment rate with one lag of itself (an AR(1) model) or a white noise model where the expected percentage change in the unemployment rate is zero. The proposed leading indicator model should outperform the benchmark models if there is additional value from considering the information contained in those leading indicators.
Results
I first considered the indicators with the longest back histories – that is, the ABS measure of vacancies, ANZ–Indeed job advertisements, the WMI measure of unemployment expectations and the summary leading indicator. Of these, the summary leading indicator and ANZ–Indeed job advertisements perform the best; models containing only the summary leading indicator or only ANZ–Indeed job advertisements have lower RMSEs than models containing other leading indicators and the benchmark models (Graph 6). Moreover, this outperformance is statistically significant, on average, over the first six quarters of the forecast period for models containing only the summary leading indicator and over the first three quarters of the forecast period for models with only ANZ–Indeed job advertisements.8 This suggests that we should use these models to inform our near-term forecasts for the unemployment rate, but need to rely on other forecasting models or techniques to inform the unemployment rate forecasts further out.
While models containing only ABS vacancies also significantly outperformed the benchmark models, they performed significantly worse than models with only the summary leading indicator or only ANZ–Indeed job advertisements. Finally, models containing the WMI measure of unemployment expectations alone perform only as well as the benchmark models. That said, information contained in both ABS vacancies and the WMI measure of unemployment expectations will still be captured in models containing the summary leading indicator.
I also considered how useful other indicators, such as measures of employment intentions, are at forecasting the unemployment rate. However, I found that models containing employment intentions only do as well as the benchmark models. This may partly reflect the tendency for these measures to capture developments in the market sector while the unemployment rate will also be affected by conditions in the non-market sector. Importantly, while these measures may not appear in the preferred forecasting models based on this out-of-sample forecast evaluation exercise, they still aid our understanding of developments in the labour market and interactions between different segments of the labour market.9
I also tested whether including other economic and labour market indicators in these models can help to improve their forecasting performance. These indicators include: measures of economic activity; survey-based indicators of tightness in the labour market, such as the share of firms reporting labour as a constraint on output and measures of capacity utilisation; and growth in real unit labour costs, which attempts to capture firms decisions to substitute away from labour when it becomes a relatively more expensive input. However, performing a similar forecast evaluation exercise suggests that including these additional variables does not materially improve forecasting performance beyond a model that only contains the leading indicators.10
Conclusion
Leading indicators of labour demand tend to be timely indicators of the labour market that can offer information on how the unemployment rate is likely to evolve going forward. Using an out-of-sample forecast evaluation exercise, I investigated which leading indicator models are relatively better at forecasting the unemployment rate and can outperform simple benchmark models. I found that the preferred leading indicator models contain only a summary leading indicator or only ANZ–Indeed job advertisements. Models with these indicators significantly outperform the benchmark models over the first year or so. Accordingly, we now use models with only a summary leading indicator or only ANZ–Indeed job advertisements to inform our near-term unemployment rate forecasts. That said, when we form our forecasts for the unemployment rate, we also consider other suites of forecasting models as well as qualitative information, such as information from the RBAs liaison program, that may not be well captured in our models.
Endnotes
The author is from Economic Analysis Department. *
For further detail on the underlying dynamics of the labour market, see Hunter (2024). 1
For a more detailed discussion of the relationship between job vacancies and labour market variables, see Edwards and Gustafsson (2013). 2
The ABS Job Vacancies Survey was suspended between May 2008 and November 2009. The analysis presented in this article uses estimates of the number of job vacancies from the Treasury Model of the Australian Economy (TRYM) for this period. 3
The SEEK Employment Index is another flow-based measure of job advertisements. Since information in SEEK job advertisements is also captured in the JSA Internet Vacancy Index, I focus on the JSA measure in this article. 4
I also considered whether a summary leading indicator that averages over a greater number of leading indicators would help forecast the unemployment rate. However, I found that these expanded versions of the summary leading indicator tend to perform about as well as the simpler summary leading indicator (which only captures information in ABS vacancies, ANZ–Indeed job advertisements and WMI unemployment expectations). I therefore focus on the simpler summary leading indicator. 5
Generating forecasts for the unemployment rate requires forecasts for the underlying leading indicator inputs. The forecasts for the latter are constructed using simple autoregressive integrated moving average (ARIMA) models that consider the historical properties of each series. 6
The models aim to produce forecasts for the percentage change in the unemployment rate, which subsequently imply forecasts for the level of the unemployment rate. I then calculated RMSEs by comparing the implied forecast for the level of the unemployment rate to the realised unemployment rate outcomes. 7
I used a Diebold-Mariano test to check whether there were statistically significant differences in forecasting performance between models. Note that there is no significant difference in performance between models with only the summary leading indicator and models with only ANZ–Indeed job advertisements. 8
While I did consider the JSA job advertisements series in a sample starting from 2006, the results are less reliable as the forecast evaluation period is very short as a result. 9
Similarly, including lags of the percentage change in the unemployment rate does not materially improve the forecasting performance of a model with only ANZ–Indeed job advertisements or only the summary leading indicator. 10
References
Ballantyne A, T Cusbert, R Evans, R Guttmann, J Hambur, A Hamilton, E Kendall, R McCririck, G Nodari and D Rees (2019), MARTIN Has Its Place: A Macroeconometric Model of the Australian Economy, RBA Research Discussion Paper No 2019-07.
Ballantyne A, A Sharma and T Taylor (2024), Assessing Full Employment in Australia, RBA Bulletin, April.
Edwards K and L Gustafsson (2013), Indicators of Labour Demand, RBA Bulletin, September.
Hunter S (2024), Understanding the Journey to Full Employment, Keynote Address to the Barrenjoey Economic Forum, Sydney, 11 September.
Lancaster D and P Tulip (2015), Okuns Law and Potential Output, RBA Research Discussion Paper No 2015-14.
Underlying data for selected graphs. Other data may be available upon request via our general enquiry page.