Bulletin – December 2020 Australian Economy Long-term Unemployment in Australia
- Download 628KB
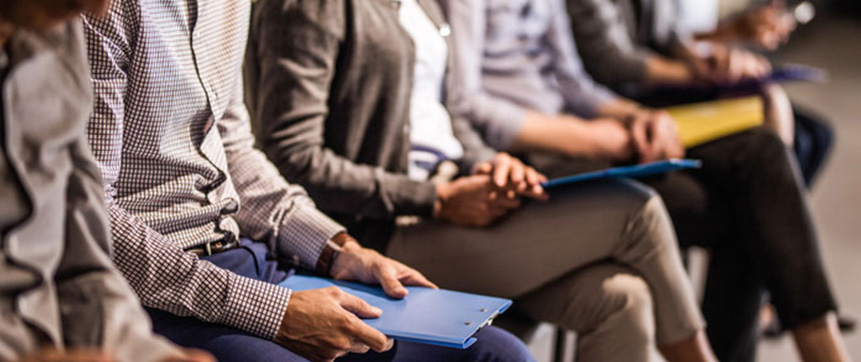
Abstract
Are your future employment prospects affected by past periods of unemployment? And does it matter how long you were unemployed? The average duration of unemployment has increased steadily over the 2010s. At the same time, the rate at which unemployed people are able to find a job has slowed. Long-term unemployed people are more likely to be older and male and have lower levels of formal education than those who have been unemployed for a shorter period. We use micro-level labour market data to show that future employment prospects are closely tied to the duration of unemployment: people who are unemployed for longer are less likely to find a job. We also find some evidence that an extended period of unemployment can harm people's employment chances for a long time afterwards.
Introduction and motivation
The COVID-19 pandemic has resulted in a substantial increase in labour market underutilisation, with more people wanting a job, or to work more hours, than employers need. While the economic outlook is highly uncertain, it is likely that the unemployment rate will remain elevated for a number of years. As such, some unemployed people are facing the prospect of a prolonged period of unemployment.
There are many social and economic consequences of long-term unemployment. The longer a person is unemployed, the harder it may be for them to find a job. This could be because they lose skills and networks, there is a stigma associated with being long-term unemployed, or because people become discouraged and leave the labour force. Long periods of unemployment are associated with lower incomes and financial stress. They can also be debilitating for the individuals, families and communities that are affected. For the economy as a whole, long-term unemployment reduces the effective pool of workers and increases the cost of welfare support.
In this article, we provide an overview of the trends in long-term unemployment in Australia over the past 30 years, and the distinguishing characteristics of the long-term unemployed. We then look at how the transition out of unemployment differs for people depending on how long they have been unemployed. While our analysis largely pre-dates the effects on the labour market from the pandemic, our results are a useful starting point for policymakers considering ways to reduce the chances that Australians face a prolonged bout of unemployment.
Trends in long-term unemployment
Long-term unemployment is defined here as being without paid work, and/or have been looking for work, for a year or more. The long-term unemployment rate, which has been relatively stable at around 1¼ per cent over the past 5 years, generally follows the overall unemployment rate with a lag (Graph 1). Following the 1990s recession, the long-term unemployment rate reached around 4 per cent, before steadily moving lower to bottom out at ½ per cent in late 2008 during the mining boom. At this time, the average duration of unemployment reached a low of 7 months.
In the following decade, leading up to the pandemic, the long-term unemployment rate increased, and the average duration of unemployment also rose to be close to one year (Graph 2). Currently around one in every 5 unemployed people have been unemployed for more than a year, an increase from around one in every 8 a decade ago. The share of very long-term unemployed people increased over this period: around 10 per cent of unemployed people have been unemployed for 2 or more years. The increase in the average duration of unemployment over the 2010s reflects a slowing in the rate at which unemployed people either gain employment or leave the labour force (with flows into unemployment more stable until recently). Average duration has fallen in 2020 because many people lost their jobs when the pandemic first broke out.
Who are the long-term unemployed?
The increase in the average duration of unemployment over the 2010s has been fairly broad based across groups (in terms of sex, age, level of education and migrant status). The average duration of unemployment tends to be higher among men, older people and those without a university degree (Graph 3). However, looking at the average duration of unemployment for different groups cannot by itself tell us who the long-term unemployed are.
We use data from the Household, Income and Labour Dynamics in Australia (HILDA) survey to look at the general characteristics of long-term unemployed people, and compare these characteristics to those of the rest of the labour force. HILDA is a rich data source that contains many household and individual characteristics unavailable elsewhere, including household finances. Compared with the pool of employed or short-term unemployed people, the long-term unemployed are more likely to be male, much less likely to have completed year 12 or tertiary education, and have significantly less household net wealth and disposable income (Table 1).[1] The long-term unemployed are also more likely to report experiencing hardship due to a shortage of money.
Long-term unemployed(a) | Short-term unemployed | Employed | |
---|---|---|---|
Age (mean) | 37 | 31 | 39 |
Male (%) | 61 | 51 | 53 |
Completed year 12 (%) | 57 | 61 | 79 |
University degree (%) | 11 | 15 | 30 |
Born in Australia (%) | 67 | 72 | 74 |
Non-English-speaking background (%) | 23 | 18 | 14 |
Household net wealth (mean, $'000 in 2018 dollars) | 423 | 492 | 920 |
Household annual disposable income (mean, $'000 in 2018 dollars) | 75 | 88 | 115 |
Within the last year due to a shortage of money: | |||
Went without meals (%) | 14 | 11 | 3 |
Was unable to heat home (%) | 9 | 6 | 2 |
Asked for financial help from friends or family (%) | 29 | 29 | 12 |
Asked for help from welfare/community organisations (%) | 15 | 13 | 2 |
(a) The long-term unemployed are those unemployed for one year or more Source: HILDA Survey Release 18.0 |
The long-term unemployed are more likely to have previously worked in the agriculture, manufacturing and retail industries relative to short-term unemployed and employed workers. They are also more likely to have previously held jobs in lower-skill occupations. Long-term unemployment is more common in regional areas than in capital cities.
Flows into and out of long-term unemployment
The Australian labour market is quite dynamic, with many people flowing into and out of employment, unemployment and the labour force each month. While the bulk of people who are either employed or outside the labour force remain in their current ‘state’ each month, on average over the past 30 years around 23 per cent of unemployed people transition into employment and a further 21 per cent leave the labour market each month. This means that a little more than half of the unemployed pool remain unemployed from month to month.
To examine how the transition rates out of unemployment differ based on how long someone has been unemployed, we make use of person-level longitudinal Labour Force Survey (LLFS) data.[2] Long-term unemployed people are, on average, less than half as likely to gain employment within a month as someone in short-term unemployment (Graph 4). The chances that a short-term unemployed worker finds a new job depends on economic conditions, with the job-finding rate declining during economic slowdowns and rising steadily during economic expansions. By contrast, the job-finding rate for the long-term unemployed is less sensitive to these cycles and so they may benefit less from favourable labour market conditions. Overall, the rate at which long-term unemployed workers find employment has fallen over the past decade. Possible explanations could be compositional changes in the pool of unemployed workers, changes in economic conditions, or changes in government policy. For example, any changes to the generosity of government income support for unemployed workers may change how intensely someone searches for work. Since unemployment benefits have been declining as a ratio of average wages for some time, incentives to search for work should have – if anything – increased.
The long-term unemployed are more than twice as likely to leave the labour market as find employment in a given month. This is consistent with longer-term unemployed people becoming discouraged from searching for work. Other potential reasons for exiting the labour force include reaching retirement age, illness, or caring responsibilities. There has also been a notable decline in the rate at which long-term unemployed people leave the labour force over the past decade. However, job search requirements to access JobSeeker have generally tightened over the past decade and are unlikely to be responsible for the decline in the rate at which long-term unemployed people leave the labour force.[3]
After controlling for other characteristics, female long-term unemployed are less likely than males to find a new job but more likely to leave the labour force.[4] Older long-term unemployed people are also less likely to find a new job. Unsurprisingly, the likelihood of leaving the labour force is higher for older long-term unemployed people.
What affects the duration of unemployment?
Intuitively, any characteristic or feature that affects the rate at which people leave unemployment can have a large cumulative effect on the likelihood that they stay unemployed over a given period of time. It may be the case that the overall increase in the average duration of unemployment in the 2010s simply reflects compositional changes in the labour force. To see if this is the case, we can use so-called survival modelling techniques to estimate the rate at which people leave unemployment while taking into account some of their personal characteristics.[5] We again make use of LLFS microdata for this exercise. We are able to construct a large dataset of individual unemployment spells, spanning the period from the mid 1980s to 2020. While we cannot follow a long-term unemployed person for their entire period of unemployment, the LLFS data provide us with the date they become unemployed and the date they either find a job or leave the labour force, or remain unemployed, in the months surveyed.
The left panel of Graph 5 shows the likelihood someone (after adjusting for their personal characteristics) remains unemployed at a given time into their unemployment spell, given that they had been unemployed until then.[6] The curves in the left panel of Graph 5 flatten quickly, indicating that the chance of someone leaving unemployment tends to be quite low after being unemployed for a year or so. The curve has shifted slightly since the 2000s, suggesting that, even holding the composition of the unemployed pool constant, there is a higher chance someone will remain unemployed in the first year of unemployment in the 2010s compared with the 2000s.
We are also interested in how an unemployment spell ends, as whether someone finds a job or instead leaves the labour market can lead to very different long-term outcomes for them. The middle and right panels of Graph 5 show that relative to the 2000s, the likelihood for the unemployed to find a job has fallen in the 2010s, at least in the first few years of an unemployment spell. At the same time, the very long-term unemployed have become more likely to leave the labour force.[7]
Even after taking other characteristics into account, ageing – at least until retirement age – is associated with a lower probability of exiting unemployment into a job (see Table A3 for the full estimation results). Interestingly, time away from work – including both time unemployed and time out of the labour force – seems to have an effect over and above the duration of unemployment. In particular, being a former worker, defined by the Australian Bureau of Statistics (ABS) as someone that last worked 2 or more years ago, further decreases the chance of exiting a spell of unemployment by around 65 per cent compared with someone who has worked recently; the result is highly statistically significant (Graph 6, left panel).[8] The effects are large and, because they accumulate over the length of an unemployment spell, can have a sizeable effect on the long-term unemployed (Graph 6, right panel). Of course, these are not necessarily causal effects from time away from work. It may also be the case that these workers are different in some other way for which we do not account.
Some consequences of long-term unemployment
We have shown that the probability of exiting unemployment declines the longer someone is unemployed, and that this is driven by a lower probability of finding work. One possible explanation for these differences is that the length of time someone is unemployed in itself lowers the likelihood of being employed. This is a specific form of ‘scarring’ from unemployment.[9] There are several explanations for this phenomenon in the literature, including skill loss during periods away from work, discrimination against the long-term unemployed by employers, or because the unemployed put less effort into searching for a job as they become discouraged.[10] Scarring may prolong unemployment, lead to lower wages on re-employment, and/or increase the probability of experiencing unemployment again.[11]
Another possible explanation for the link between longer periods of unemployment and poor labour market outcomes is inherent differences (e.g. innate ability and skills) between people who are unemployed for short periods and those unemployed for longer periods. For instance, the long-term unemployed report facing different barriers to finding work than the short-term unemployed. The long-term unemployed are much more likely to report a physical disability or ill health as being their main difficulty in finding work. They are also more likely to report that employers consider them too old. Conversely, the short-term unemployed are more likely to report there being too many applicants, or that they lacked relevant skills.
If, after accounting for differences across people, we find evidence of a causal relationship between the duration of unemployment and future job and wage prospects, then policies aimed at reducing unemployment duration may lower the overall loss to society from unemployment.
Is there any evidence of scarring from the duration of unemployment?
We find some tentative evidence that the duration of unemployment leads to scarring. To do this, we look at the difference in employment rates for individuals before and after unemployment spells of varying duration.[12] Underlying this approach is the idea that any inherent differences in job-finding rates between people who become short- and long-term unemployed should be evident both before and after an unemployment spell. If the likelihood of finding a job after an unemployment spell falls relative to before, then there is evidence of scarring from experiencing a period of unemployment. If groups who are unemployed for longer experience the largest falls in employment probabilities, then this would suggest the detrimental effects of unemployment increase as unemployment duration increases. That is, there should be larger differences in the likelihood of being employed between the long- and short-term unemployed after their unemployment spells, relative to before.
Even after accounting for education, experience and economic conditions, we see systematic differences in employment rates in the years before an unemployment spell for those that are long-term unemployed compared with the short-term unemployed (left panel of Graph 7).[13] This is due to inherent differences across these groups. Those who go on to be long-term unemployed are less likely to have been employed 5 years before the spell than those who become short-term unemployed.
For those who experienced longer unemployment spells, there is a clear decrease in employment probabilities 5 years after they are observed to be unemployed. For those unemployed for shorter durations, however, there is no obvious decline in employment probabilities over the same timeframe. This difference between the long- and short-term unemployed is suggestive of a causal relationship between the duration of unemployment and future labour market outcomes, and provides evidence of scarring that increases with employment duration. This is consistent with Abraham et al (2016), who find a causal relationship between the duration of unemployment and future unemployment for US workers, but in contrast to Doiron and Gorgens (2008), who find no such relationship for young, low-skilled Australian workers from unemployment duration alone (while finding evidence for an effect from the number of unemployment spells).
We used the same methodology to see if the duration of unemployment affects someone's eventual labour income (Graph 8). To remove the effect of being unemployed on earnings, we limit our focus to only those people who regain employment. It is clear that the long-term unemployed fare significantly worse than the short-term unemployed after regaining work, even after accounting for age and experience – this is shown by the gap in wages between the long- and short-term unemployed in the right panel of Graph 8. However, the fact that similar differences in wages between the long- and short-term unemployed are also present before observed unemployment means there is no clear evidence of scarring from the duration of unemployment on wages, at least among workers who re-enter employment. Our findings therefore suggest that any persistent income losses from long-term unemployment may arise mainly from a lower probability of finding a job, rather than lower wages upon re-employment. This finding is somewhat different from the international literature that does find a negative effect of unemployment duration on wages; these international studies, however, tend to use large administrative datasets that are not yet widely available for researchers here.[14] Administrative data would allow us to follow individual workers – including details on their employment history and earnings – over a longer period of time. This would give us a clearer picture of the consequences of long-term unemployment in Australia, and provide a more appropriate comparison with the international literature.
Conclusion
The probability of gaining employment is lower for people who have had longer unemployment spells. We find some evidence of scarring from unemployment, with long-term unemployed people experiencing worse employment outcomes relative to those who were unemployed for a shorter period. In Australia and abroad, the adverse effects on individuals, society and the economy as a whole from prolonged spells of unemployment will be important considerations in the setting of public policy following the COVID-19 shock.
Appendix A
Long-term unemployed(a) | Short-term unemployed | Employed | |
---|---|---|---|
Married (%) | 38 | 34 | 64 |
Has resident children (%) | 31 | 27 | 44 |
Aboriginal and/or Torres Strait Islander (%) | 7 | 6 | 1 |
Self-assessed poor health (%) | 1 | 2 | 1 |
Inner regional (%) | 19 | 18 | 17 |
Outer regional/remote (%) | 12 | 9 | 10 |
Years of work experience (mean) | 15 | 12 | 20 |
Reservation wage (mean, $) | 20.9 | 21.8 | - |
Preferred hours at reservation wage (mean) | 32.3 | 29.4 | - |
Tenure with last employer (mean, years) | 2.9 | 1.8 | - |
SEIFA decile of relative socio-economic advantage (median) | 3 | 5 | 6 |
Occupational status (last job if not employed) (mean) | 32 | 36 | 50 |
Owns home (%) | 45 | 50 | 67 |
Sold home because of financial difficulties (if owns property and has sold property within the last 4 years) (%) | 35 | 21 | 7 |
Hours searching for a job in last week (if unemployed, mean) | 6.7 | 6.5 | - |
(a) The long-term unemployed are those unemployed for one year or more Source: HILDA Survey Release 18.0 |
Remain unemployed | Exits to employment | Leaving the labour force | |
---|---|---|---|
Sex (base category: male) | |||
Female | –0.065*** | –0.002 | 0.066*** |
Age (base category: 25–34) | |||
15–19 | –0.007 | 0.015*** | –0.008** |
20–24 | 0.012*** | 0.008*** | –0.020*** |
35–44 | 0.001 | –0.007*** | 0.006** |
45–54 | –0.004 | –0.020*** | 0.024*** |
55–59 | –0.018*** | –0.036*** | 0.054*** |
60–64 | –0.083*** | –0.053*** | 0.136*** |
Country of birth (base category: Australia) | |||
Migrant from main English-speaking country(b) | 0.011*** | 0.000 | –0.011*** |
Migrant from elsewhere | –0.007** | –0.011*** | 0.018*** |
Social marital status (base category: not married) | |||
Married | –0.014*** | 0.009*** | 0.006** |
Number of children in household (base category: 0) | |||
1 | –0.021*** | 0.002 | 0.019*** |
2 | –0.030*** | 0.004* | 0.026*** |
3 or more | –0.012** | –0.006** | 0.028*** |
Job search (base category: looked only for full-time work, or for both full- and part-time work) | |||
Looked only for part-time work | –0.187*** | 0.021*** | 0.166*** |
Employment history (base category: recent worker, i.e. last worked less than 2 years ago) | |||
Former worker | 0.005* | –0.039*** | 0.034*** |
Never worked | –0.037*** | –0.027*** | 0.064*** |
State and area dummies | Yes | Yes | Yes |
Number of observations | 184 516 | 184 516 | 184 516 |
Number of transitions | 137 119 | 14 754 | 32 643 |
(a) ***, ** and * denote statistical significance at the 1, 5 and
10 per cent levels; standard errors are clustered at the
respondent level Sources: ABS; authors' calculations |
All exits | Exits to employment | Leaving the labour force | |
---|---|---|---|
Sex (base category: male) | |||
Female | 1.13*** | 1.04*** | 1.25*** |
Age (base category: 25–34) | |||
15–19 | 1.07*** | 0.91*** | 1.32*** |
20–24 | 0.95*** | 0.93*** | 0.99 |
35–44 | 0.96*** | 0.99 | 0.94*** |
45–54 | 0.86*** | 0.84*** | 0.90*** |
55–59 | 0.75*** | 0.64*** | 0.89*** |
60–64 | 0.71*** | 0.48*** | 0.96** |
65 and above | 0.89*** | 0.70*** | 1.06** |
Country of birth (base category: Australia) | |||
Migrant from main English-speaking country(b) | 1.04*** | 1.06*** | 1.01 |
Migrant from elsewhere | 0.89*** | 0.74*** | 1.06*** |
Relationship in household (base category: head of couple family) | |||
Head of lone-parent family | 0.84*** | 0.57*** | 1.21*** |
Children aged 15 and over | 0.94*** | 0.84*** | 1.09** |
Living alone | 0.71*** | 0.61*** | 0.85*** |
Social marital status (base category: not married) | |||
Married | 0.98 | 0.87*** | 1.16*** |
Number of children in household (base category: 0) | |||
1 | 0.99** | 0.93*** | 1.06*** |
2 | 1.03*** | 0.95*** | 1.12*** |
3 or more | 0.99* | 0.83*** | 1.16*** |
Job search (base category: looked only for full-time work, or for both full- and part-time work) | |||
Looked only for part-time work | 1.62*** | 1.08*** | 2.31*** |
Employment history (base category: recent worker, i.e. last worked less than 2 years ago) | |||
Former worker | 0.35*** | 0.17*** | 0.60*** |
Never worked | 0.60*** | 0.38*** | 0.88*** |
Area of state effects? (e.g. Greater Sydney) | Yes | Yes | Yes |
Number of unemployment spells | 424 034 | 424 034 | 424 034 |
Number of exits | 266 896 | 132 690 | 134 206 |
Number of respondents | 368 760 | 368 760 | 368 760 |
(a) Results presented are exponentiated coefficients. ***, ** and * denote statistical
significance at the 1, 5 and 10 per cent levels; standard
errors are clustered at the respondent level; to estimate the baseline
hazards, monthly intervals were used for the first 3 years of
an employment spell, then every quarter until 5 years after the
start of a spell, and yearly thereafter until 10 years after the
start of a spell; baseline hazards are allowed to vary every decade
Sources: ABS; authors' calculations |
Footnotes
The authors are from the Economic Analysis and Economic Research Departments. This document uses unit record data from the Household, Income and Labour Dynamics in Australia (HILDA) Survey. The unit record data from the HILDA Survey was obtained from the Australian Data Archive, which is hosted by The Australian National University. The HILDA Survey was initiated and is funded by the Australian Government Department of Social Services (DSS) and is managed by the Melbourne Institute of Applied Economic and Social Research (Melbourne Institute). The findings and views based on the data, however, are those of the authors and should not be attributed to the Australian Government, DSS, the Melbourne Institute, the Australian Data Archive or The Australian National University and none of those entities bear any responsibility for the analysis or interpretation of the unit record data from the HILDA Survey provided by the authors. [*]
See Appendix Table A1 for additional summary statistics. Using a probit model we find age, sex and education to be statistically significant in predicting long-term unemployment among the unemployed. The older an individual, the higher the odds of them being unemployed for longer durations. Conversely, having more education is associated with lower odds of becoming long-term unemployed. [1]
The Labour Force Survey follows people over time (every month for up to 8 months). The LLFS data – the longitudinal data from this survey – available to the RBA contain over 27 million responses to the monthly labour force survey from 1982 onwards, and include respondents' employment outcomes in the surveyed months as well as some data on worker characteristics. [2]
The main payment for those over 21 years of age but under pension age is the JobSeeker Payment (formerly known as the Newstart Allowance). During the COVID-19 pandemic, the government temporarily increased unemployment payments and waived some eligibility criteria, as well as job search requirements, for the JobSeeker Payment. [3]
We do this using a multinomial probit model. See Appendix Table A2 for model estimates. [4]
Survival models are typically used to analyse the expected time until some event happens, such as when an unemployment spell ends. Examples from the literature in Australia include Carroll (2006) and Borland and Johnston (2010), who employ survival modelling with HILDA data, while Rotaru (2014) analyses 3 years of the LFS microdata. These papers tend to focus on the individual factors associated with exits from unemployment and not on the estimated baseline rates of exits after accounting for those individual factors. We use a piecewise exponential model to look at how the rate of exit has changed over time. The ability to choose arbitrarily short duration intervals makes this approach very flexible and well suited for estimating the baseline hazard and associated survival curves. [5]
We hold certain characteristics constant over the entire sample period, such as the respondent's age group, sex, area of state, country of birth, household relationship, marital status, number of children, whether they searched exclusively for part-time work, and whether they are a former/recent worker or had never worked before. Other important variables, such as education and last industry of employment, are not included as they are not consistently available in the data. [6]
The exit rates to employment and to outside the labour force do not sum to total exits because the 2 types of exits have been modelled separately. The models also do not account for changes to government policy, which (for instance) may affect workers' incentives to keep searching for a job or leave the labour force. [7]
This is consistent with Kroft et al (2019), who, using Canadian data, find that time away from work matters as much as unemployment duration for the job-finding rate. [8]
This phenomenon is a component of the broader concept of scarring, which typically includes the longer-term adverse consequences of a downturn on labour market outcomes. In this article we consider scarring only as it relates to the length of an unemployment spell. While not discussed in this article, previous literature on the broader concept of scarring has found that workers graduating in a recession experience persistently lower earnings than otherwise both internationally (Oreopoulos, von Wachter and Heisz 2012) and in Australia (Fontenay et al 2020; Andrews et al 2020). [9]
For a review of the Australian and international literature see Borland (2020). [10]
Our focus in this article has been on some of the economic outcomes from long-term unemployment. There are of course serious effects on physical and mental health from a lengthy unemployment spell; see, for example, Sullivan and von Wachter (2009). [11]
We use the employment share of the population, instead of the labour force, to account for the possibility that scarring may occur through an increase in exits out of the labour force. [12]
We remove the compositional effects from these variables by estimating an equation where employment is a function of education, work experience, and calendar-year indicator variables. Our estimates in Graph 7 can be interpreted as observed employment probabilities, purged of differences in education, experience and macroeconomic conditions. [13]
For instance, Schmieder, von Wachter and Bender (2016) use administrative German data to find that each additional month of non-employment causes average daily wage decline by less than 1 per cent but this effect fades over a few years; Cooper (2014) finds, using US survey data, that the negative effects on earnings persist for many years. Again using US data, Abraham et al (2016) also find an effect on wages, albeit a much smaller one than that on unemployment. By contrast, Arulampalam (2001) finds no evidence in UK survey data that unemployment duration is associated with lower wage outcomes (but the number of unemployment spells does have a negative effect). [14]
References
Abraham K, J Haltiwanger, K Sandusky and J Spletzer (2016), ‘The Consequences of Long-Term Unemployment: Evidence from Linked Survey and Administrative Data’, ILR Review, Cornell University, 72(2), pp 266–299.
Andrews D, N Deutscher, J Hambur and D Hansell (2020), ‘The Career Effects of Labour Market Conditions at Entry’, Treasury Working Paper 2020-01.
Arulampalam W (2001), ‘Is Unemployment Really Scarring? Effects of Unemployment Experiences on Wages’, The Economic Journal, 111(475), pp 585–606.
Borland J (2020), ‘Scarring effects: A review of Australian and international literature’, Australian Journal of Labour Economics, 23(2), pp 174–187.
Borland J and D Johnston (2010), ‘How Does a Worker's Labour Market History Affect Job Duration?’, Melbourne Institute Working Paper 6/10.
Carroll N (2006), ‘Explaining Unemployment Duration in Australia’, Economic Record, 82(258), pp 298–314.
Cooper D (2014), ‘The Effect of Unemployment Duration on Future Earnings and Other Outcomes’, Federal Reserve Bank of Boston Working Papers 13-8.
Doiron D and T Gorgens (2008), ‘State dependence in youth labor market experiences, and the evaluation of policy interventions’, Journal of Econometrics, 145(1-2), pp 81–97.
Fontenay Cd, B Lampe, J Nugent and P Jomini (2020), ‘Climing the jobs ladder slower: Young people in a weak labour market’, Productivity Commission Staff Working Paper.
Kroft K, F Lange, MJ Notowidigdo and M Tudball (2019), ‘Long Time Out: Unemployment and Joblessness in Canada and the United States’, Journal of Labor Economics, 37(S2), pp S355–S397.
Oreopoulos P, T von Wachter and A Heisz (2012), ‘The Short- and Long-Term Career Effects of Graduating in a Recession’, American Economic Journal: Applied Economics, 4(1), pp 1–29.
Rotaru CI (2014), ‘Transitioning Out of Unemployment: Analysis Using the ABS Longitudinal Labour Force Survey File’, Australian Journal of Labour Economics, 17(2), pp 111–137.
Schmieder JF, T von Wachter and S Bender (2016), ‘The Causal Effect of Unemployment Duration on Wages: Evidence from Unemployment Insurance Extensions’, American Economic Review, 106(3), pp 739–777.
Sullivan D and T von Wachter (2009), ‘Job Displacement and Mortality: an Analysis Using Administrative Data’, Quarterly Journal of Economics, 124(3), pp 1265–1306.