Bulletin – September 2019 Payments Survival Analysis and the Life of Australian Banknotes
- Download 1MB
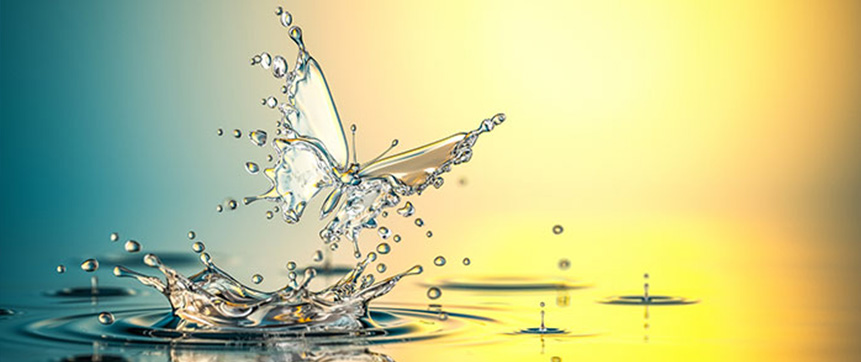
Abstract
The Reserve Bank is in the process of replacing Australia’s first full series of polymer banknotes – the ‘New Note Series’ – with the upgraded ‘Next Generation Banknote’ series. This presents a good opportunity to review our experience with polymer banknotes, and in particular, examine how they have worn in practice. To do so, I extend an existing survival modelling approach to estimate how long a given polymer banknote from the ‘New Note Series’ might be expected to last when in use by the general public. I find that $5 and $10 banknotes have tended to last for around 5 years on average, while $20 and $50 banknotes have lasted for 10 and 15 years on average, respectively. I have not modelled $100 banknotes as they are overwhelmingly used for store-of-value purposes and so do not tend to wear out.
Introduction
In this article I estimate how long a typical Australian polymer banknote from the ‘New Note Series’ (NNS) lasts. In particular, I estimate a survival model of banknote life based on Rush (2015) which incorporates factors that can affect the life of a banknote, including significant economic events and changes to the Reserve Bank's policies around banknotes. Given certain modelling assumptions, the output of this model gives a complete description of the lifecycle of a banknote, including the distribution of outcomes. I briefly compare the results with alternative and simpler, turnover-based ways to estimate banknote life.
The survival function
The life and death of a banknote is analogous to the life and death of a biological organism. From the day it is issued, a banknote is subject to a set of random mechanical hazards. Banknotes presenting with staples, stains, holes or tears, to name a few, will be deemed unfit and destroyed by the Bank. But those that evade the many mechanical hazards that exist in circulation may have a long life. For these banknotes, the end of their useful life can occur either through an accumulation of inkwear, or because the series they belong to is eventually retired.
Survival models are often used by medical researchers, biologists and engineers to describe the probability of some event, like death or a product failure, occurring after a point in time. In banknote life, the event of interest is the destruction of an unfit banknote, which occurs when the banknote is returned to the Bank as unfit. For T the time of destruction, the survival function as a function of t is defined as:
A related concept is the death function, or the lifetime distribution function as it is known in the survival literature. The lifetime distribution function represents the probability a banknote will be destroyed by time t, and in fact is simply the cumulative distribution function (CDF) from statistics:
Differentiating with respect to t gives the instantaneous destruction rate (the probability density function or PDF from statistics), which is also known as the Event Density function :
Scaling the destruction rate by the proportion of surviving banknotes gives the hazard rate. The hazard rate represents the proportion of banknotes that are expected to be destroyed at time t, conditional on their survival up to that point:
Graph 1 shows the predicted survival curve and its associated destruction rate and hazard rate for a single issuance of $5 banknotes, based on historical issuance and destruction data. Half of the issued banknotes are expected to be destroyed within about 6 years, with the destruction rate reaching its peak shortly thereafter. The flattening of the tail of the survival curve beyond this point indicates that a relatively small number of banknotes will last longer, although a sharp increase in the hazard rate shows that the probability of death increases rapidly from here, perhaps reflecting accumulated inkwear for the few surviving banknotes.
The Joint Survival Function
In survival modelling, researchers typically have the ability to observe the life and death of individual subjects, as well as their individual characteristics, and can use these data in estimating a survival function that describes the population. Unfortunately, no such individual-level data exists for Australian banknotes at the moment, although this may change in the future as serial number tracking in banknote processing machines becomes more widespread.
To overcome this, Rush (2015) proposed constructing a joint survival function that relies on aggregate issuance and destructions data, where the total quantity of surviving banknotes in existence is modelled as the sum of surviving banknotes from each issuance. Rush took advantage of the fact that every banknote is printed using the same method on the same materials, and assumed that each of the individual banknote survival functions were identical. Only the age of each issuance and the prevailing economic conditions change. This means that a single set of parameters are sufficient to describe the life-cycle of every banknote, regardless of when it was issued. Graph 2 illustrates the concept, where each of the grey lines represents a single issuance and its decay over time. The blue line is the sum of the survival functions, which is also the expected quantity of outstanding banknotes in existence, measured in millions of pieces.
Because issuances vary in size, the expected total quantity of outstanding banknotes, is equal to the sum of each issuances' survival function, , multiplied by the quantity of such banknotes issued, . Here is a vector of common parameters that must be estimated and is the age of the nth issuance at time t:
Abstracting from lost banknotes, the actual quantity of outstanding banknotes, , can be calculated from the Bank's aggregate data as the sum of total issuances less total destructions:
To estimate a joint survival function that best captures the actual life of banknotes, the objective is then to estimate parameters that minimise the difference between the expectation and the observed quantity of outstanding banknotes:
Activity Time
In the natural world, lifetimes are measured in years. This is also typically how most people view the age of a banknote. But banknotes do not age per se, they wear. Both mechanical and inkwear hazards are a function of the number of transactions a banknote undergoes throughout its life. This means, for example, that banknotes used primarily as a store of value will last many years longer than those that are transacted often.
We deviate from Rush's methodology by replacing chronological time with activity time – a quasi-time dimension that speeds up or slows down in response to macroeconomic conditions or changes in the currency issuer's banknote processing policy. The idea that time can run fast or slow is not new, and there is a large literature on asset pricing based on activity time and ‘subordinator’ models.[1] We use an additive method to construct the activity time, which ensures that time cannot run backwards and so constrains the survival function to be monotonically decreasing. Besides ensuring internal consistency, the method also adds ‘memory’ to the model, in the sense that if time ‘ran slowly’ for a period of a banknote's life, it is not forced to jump-back into line with chronological time once that period passes.
To be precise, for representing activity time, a matrix of explanatory variables (such as the velocity of cash, and control variables for Y2K and the GFC; see Appendix A for a complete list), and is a vector of parameters estimated within the model, we define activity time as:
Choosing a Distribution
A survival function can in principal take almost any shape as long as it starts at one and is non-increasing. Banknotes are exposed to two types of hazards: the first is mechanical damage which occurs randomly; and the second is inkwear, which accumulates with use. If mechanical hazards were the only cause of death, then one might expect the hazard rate to be constant, since the chance of a banknote becoming unfit would be the same no matter how old a banknote was. If inkwear were the primary cause, then one might expect banknotes to have similar lives and ‘die’ once they had been used in their allotted number of transactions. Lost, hoarded, or relatively unused banknotes further complicate the analysis. Rush takes a novel approach by separating out such banknotes from circulation. They are then locked away safely until the end of the study period. We allow for the probability that individual banknotes can change states. That is, banknotes can be temporarily hoarded before re-entering the cash economy at a later date. Nonetheless, lost, hoarded, or less frequently used banknotes are accommodated by allowing for a fat-tailed survival distribution.
The above considerations suggest that any candidate distribution will require a significant degree of flexibility to allow for the full range of possibilities. We use the Generalised Gamma (GG) distribution, which is the general form of a family of distributions that include the Exponential, Weibull, Log Normal and Gamma.[2] More importantly, the GG includes all four of the most common types of hazard function used in survival modelling: monotonically increasing and decreasing, as well as ‘U’ and arc-shaped hazards (Cox et al 2007).
Results
Graph 3 shows the model's overall fit for the $5 to $50 NNS denominations. Because relatively few $100 NNS banknotes have been returned to the Bank for processing, their expected life-span far exceeds the available data. For this reason, $100 banknotes have been excluded from the analysis.
The blue shading on Graph 3 highlights targeted banknote quality programs and other denomination specific factors, while grey shading indicates significant events that are expected to affect all denominations to some extent, like the GFC (see Appendix A for more information). Most highlighted episodes are associated with some increase in the public's demand for banknotes and/or a policy change from the Bank that tends to increase banknote life. For example, demand for banknotes significantly increased ahead of Y2K, which resulted in each individual banknote being used less and so higher banknote life, despite an overall increase in transaction demand.
* NNS represents the introduction of NNS banknotes; Recolour is a change in colour to the $5 banknotes; FED represents the issuance of a special edition 'Federation $5' banknote; VCH relates to a change in distribution policy; Quality 1 and Quality 2 are various banknote cleansing programs. See Appendix A for more detail.
The fit of the survival model improves with time, particularly post 2006 when there were fewer banknote cleansing programs and other such distortions. The model performs particularly well on the $50 denomination. Australian $50 banknotes are thought to be primarily used as a store of value, while lower denominations are used more for transactions.[3] High levels of hoarding will tend to smooth out the effect of economic events on banknote life, given that the relatively low share of banknotes that are actively circulating are the only ones affected. The accumulated effects of transitory events are also less relevant to high-value banknotes that have a long life-span, since a smaller proportion of their life is affected. This, and the fact there were no cleansing programs aimed at the $50, probably explains why that denomination is the easiest to model.
Parameter estimates are presented in Table 1. The first three parameters, and , determine the fit of the underlying GG survival function, while the remaining parameters define the activity time process, where positive values serve to speed up time and so reduce banknote life, and negative values slow time and so extend life. It's worth noting that the model is non-linear and subject to a scaling parameter ( ), so the coefficients cannot be compared directly between denominations. A description of each variable can be found in Appendix A.
$5 | $10 | $20 | $50 | ||||||||
---|---|---|---|---|---|---|---|---|---|---|---|
Variable | Estimate | Sig. | Estimate | Sig. | Estimate | Sig. | Estimate | Sig. | |||
Survival curve parameters | |||||||||||
(location) | 5.00 | *** | 4.83 | *** | 5.23 | *** | 6.61 | *** | |||
(scale) | 0.19 | *** | 0.60 | *** | 0.06 | *** | 0.07 | *** | |||
(shape) | 1.89 | *** | 1.47 | *** | 19.55 | *** | 11.87 | *** | |||
Activity time parameters – environmental | |||||||||||
Velocity | 13.29 | *** | 11.32 | *** | 15.64 | *** | 55.77 | *** | |||
Velocity * t | −0.04 | *** | −0.03 | *** | −0.06 | *** | −0.12 | *** | |||
Y2K | −2.19 | *** | −1.65 | *** | −2.03 | *** | −1.19 | *** | |||
GFC | −0.03 | −0.15 | * | −0.63 | *** | 0.18 | *** | ||||
Surplus Fit Holdings | 10.82 | *** | −7.33 | *** | −8.97 | *** | −1.55 | *** | |||
Activity time parameters – banknote policy changes | |||||||||||
NNS | 0.65 | *** | 0.38 | *** | 0.56 | *** | 0.72 | *** | |||
Federation $5 | −1.14 | *** | |||||||||
Recolour $5 | 1.81 | *** | |||||||||
Quality program | 0.19 | *** | 0.40 | *** | 0.73 | *** | |||||
Quality program 2 | −0.49 | *** | 0.32 | *** | |||||||
VCH Distribution policy | −0.96 | ** | −0.37 | −1.59 | *** | 0.43 | ** | ||||
NGB | 0.82 | *** | 1.43 | *** | |||||||
MAPE (per cent) | 2.3 | 1.7 | 2.0 | 0.8 | |||||||
RMSE (million pieces) | 3.1 | 2.0 | 3.5 | 3.1 | |||||||
Degrees of freedom | 303 | 288 | 277 | 268 | |||||||
***, **, and * denote statistical significance at the 1, 5, and 10 per cent levels, respectively. |
The signs of the coefficients for variables related to the prevailing economic environment are broadly in line with what one might expect. Banknotes age more quickly when their velocity throughout the cash economy increases, but this effect has decreased over time. Y2K and the GFC are generally thought to have led to temporary increases in precautionary banknote demand, increasing banknote life. Banknote life also tends to increase with the Bank's ‘surplus fit holdings’ (these are used but still-fit banknotes that are stored in the Bank's vaults) as one would expect, since banknotes held in storage do not really wear.[4]
The appropriate signs for the variables related to changes in banknote policies are less obvious though. Banknote quality programs, for example, which seek to replace a significant share of outstanding worn banknotes with freshly printed ones, initially slow the destruction rate as new banknotes are issued in preparation for the program. The effect is then reversed once commercial banks start returning their stock of old banknotes for processing.
While the overall fit is encouraging, the model needs to capture the entire distribution if it is to provide valid information beyond just simple measures of central tendency. One way to check whether this is the case is to compare the model's predictions to data observed by the Bank in the course of its operations. Graphs 4 and 5 compare predictions of the average age of fit banknotes in circulation and unfit banknotes returned for processing to information taken from the Bank's commercial cash sampling (CCS) program.
In particular, the Bank records the year of manufacture of around 10,000 banknotes that are deposited by retailers each year and then sent on to the Bank for analysis. It is important to note, however, that the year of manufacture says little about when a banknote was actually issued to the public, and so to use these data to estimate how long a particular banknote has been in circulation I need to make assumptions around the timing of issuances.[5]
Overall, Graphs 4 and 5 suggest that the banknote life estimates, while close, are slightly upwardly biased overall. For unfit banknotes, the apparent bias can be explained when we recognise that the model predicts the age of unfit banknotes at the time of processing, whereas the sample data are for banknotes still in circulation which would otherwise have lived longer before they were returned to the Bank. It is less clear why the models appear to be slightly biased in regards to fit banknotes – either the models are somewhat misspecified or the assumptions used to calculate the age of banknotes from the CCS data are somewhat inaccurate. Most likely, both are true to some extent.
Graph 6 shows the average survival curve for each denomination, taken from the time they were first issued until the end of 2018. The dashed line at 50 per cent intersects with the median life for all banknotes in that denomination. Given that our data are largely silent on the destruction date of long-living, high-value denominations, the tail of the $50 distribution in particular, should probably be ignored.[6]
Comparison with Alternative Model Estimates
There are alternative ways to estimate banknote life. The most common method is based purely on the turnover of banknotes, and is simply the number of outstanding banknotes divided by the number of banknotes destroyed in a year. This method provides an estimate of central tendency but says nothing about the distribution of banknote life. The method is also sensitive to various idiosyncratic factors such as banknote demand shocks and changes in banknote processing policies. A slightly more sophisticated method, again based on turnover, is detailed in Feige (1989) and calculated as the average number of outstanding banknotes divided by the average of old banknotes destroyed and new banknotes issued each year (hereafter the ‘Feige’ method). Again, it says nothing about the distribution of banknote life and is still sensitive to various idiosyncratic factors, albeit less so.[7]
These turnover-based methods estimate banknote life using a cross-section of data taken at a particular point in time. In doing so, they implicitly assume that whatever was happening at the time will continue to happen into the future. That is, the prevailing conditions, or the state, are assumed to remain unchanged, and so these methods are referred to as steady-state methods.
Graph 7 compares some steady-state estimates of average banknote life with those from the model. As can be seen, one issue with steady-state methods is that they can be highly volatile. This stems from the fact that the steady-state methods are generally estimated based on a window of a single year of data. The effects from temporary factors are magnified because they are assumed to remain in place for the entire life of the series. Such factors usually have little to do with the structural integrity of the banknote, but are more often the result of the currency issuer's own policies. For example, in 1999 the Bank ceased destroying unfit banknotes to build a contingency stock for Y2K. The effect was to increase the steady-state estimates of banknote life at the time, and then reverse this once the contingency event had passed. In contrast, the policy change had little effect on our estimates of banknote life. More recently, the Bank limited its processing of the remaining stock of $20 NNS banknotes. This was an operational decision to ensure that the current stock of $20s would last until the new NGB series are issued in late 2019. This doubled the estimates of banknote life using the simple turnover-based measure, but had almost no effect on the model-based survival estimates which use all of the available data.
Conclusion
In late 2016, 2017 and 2018 respectively, the Bank released upgraded $5, $10 and $50 NGB banknotes, and the existing stock of old NNS series polymer banknotes were deemed unfit; the new $20 NGB is due to be released in late 2019. This banknote upgrade program, after roughly 25 years using the previous polymer banknote series, provides a good opportunity to evaluate how our banknotes have worn in use. Overall, I find that over their period of use, the average life of old-series polymer banknotes increases as the denomination gets higher: a little over 5 years for the $5 and $10; a little over 10 years for the $20; and around 15 years for the $50. This is consistent with lower-denomination banknotes incurring higher wear through greater use for transactions.
Appendix A
Variable | Description |
---|---|
Velocity | The ratio of monthly ATM withdrawals, by value, to circulating banknotes, by denomination, is used as a proxy for the velocity of cash. |
Velocity * t | The velocity variable times a time trend. |
Y2K | An indicator variable is included for each denomination to allow for the RBA's precautionary measures and the possibility that public demand for banknotes increased around Y2K. The variable is equal to one between August 1999 and July 2000. |
GFC | The GFC may have led to precautionary demand for banknotes or changes in use patterns. An indicator variable equal to one between October 2008 and August 2009 is included for each denomination. |
Surplus Fit Holdings | Banknotes in excess of public demand are sometimes returned to the RBA where they are stored until they are re-issued at a later date. A variable is included that captures the proportion of surviving banknotes that are held at the RBA as surplus fit holdings. |
NNS | An indicator variable is included for each denomination to allow for the possibility that hazard rates can differ when a banknote series is first issued. For the $5, $20 and $50 denominations the indicator variable is equal to one for the first two years. This is extended to 3.5 years for the $10 banknote since there were some minor changes to the ink used. |
Federation $5 | A commemorative $5 banknote celebrating the Centenary of Federation was issued in 2001 and withdrawn the next year. An indicator variable has been included and is equal to one from January 2001 to September 2002. |
Recolour $5 | The first $5 polymer banknotes released in 1992 were considered too pale. A brighter coloured version was subsequently released in 1995. An indicator variable has been included to allow for the possibility that hazard rates were affected during the period from May to December 1995. |
Quality Programs | Indicator variables are used to capture the effect of targeted cleansing programs designed to improve the quality of banknotes in circulation. These were implemented for the $5 denomination in 2011, $10 in 2005 and 2009, and for the $20 banknote in 2006 and 2007. |
VCH Distribution Policy | The RBA changed its cash management practises in 2001, transferring banknote holdings from the RBA to commercial banks. An indicator variable equal to one between November 2001 and April 2002 is included for each denomination to allow for the policy change. |
NGB | Next Generation Banknote series were issued to the public in late 2016, 2017 and 2018 for the $5, $10 and $50 denominations respectively, and the old NNS series declared unfit. An indicator variable equal to one is included from two months prior to NGB's release until the end of the series for each of these denominations. The additional two months allows for the time taken to stock the commercial banks prior to the public release date. |
Footnotes
The author is from Note Issue Department and would like to thank Richard Finlay, Alexandra Rush, Luci Ellis, Tony Richards, Carl Schwartz and Sandra Wilkinson for their help. [*]
See for example Heyde (1999) and Finlay and Seneta (2006) for early examples that seek to model activity time in a realistic way. [1]
The GG distribution has density function: [2]
Finlay, Staib and Wakefield (2018) discuss the drivers of banknote demand in more detail. [3]
The exception here is the $5 denomination, where the relationship inverts. On closer inspection, this particular coefficient probably suffers from omitted variable bias: the Bank generally only holds a small quantity of surplus fit $5 banknotes for around two months each year, immediately after the Christmas period, which is the time when cash demand is at its peak. [4]
Each banknote has its own unique serial number, and in principle this information could also be used to provide a wealth of information about banknote life. While this is likely to become the case in future, it has not been the case in the past: historically, serial numbers have not generally been recorded at the time of banknote issuance, and until recently the technology to capture them at the time of processing was also very expensive or unavailable. [5]
This is also why $100 banknotes were excluded from the analysis; steady state methods suggest that $100 NNS banknotes last around 200 years on average. [6]
See Rush 2015 for more information on these turnover methods. [7]
References
Cox C, H Chu, MF Schneider and A Muñoz (2007), ‘Parametric survival analysis and taxonomy of hazard functions for the generalized gamma distribution’, Statistics in Medicine, 26, pp 4252-4374.
Doyle M-A, C Fisher, E Tellez and A Yadav (2017), ‘How Australians Pay: Evidence from the 2016 Consumer Payments Survey’, RBA Research Discussion Paper No RDP 2017-04.
Feige EL (1989), ‘Currency Velocity and Cash Payments in the U.S. Economy: The Currency Enigma’, Munich Personal RePEc Archive Paper No 13807.
Finlay R and E Seneta (2006), ‘Stationary-Increment Student and Variance-Gamma Processes’, Journal of Applied Probability, 43(2), pp 441–453.
Finlay R, A Staib and M Wakefield (2018), ‘Where’s the Money‽ An Investigation into the Whereabouts and Uses of Australian Banknotes', RBA Research Discussion Paper No 2018-12.
Heyde CC (1999), ‘A risky asset model with strong dependence through fractal activity time’, Journal of Applied Probability, 36(2), pp 1234–1239.
Rush A (2015), ‘The Life of Australian Banknotes’, RBA Research Discussion Paper No 2015-10.