Bulletin – December 2022 Financial Stability New Measures of Financial Stress from Non-traditional Data
- Download 826KB
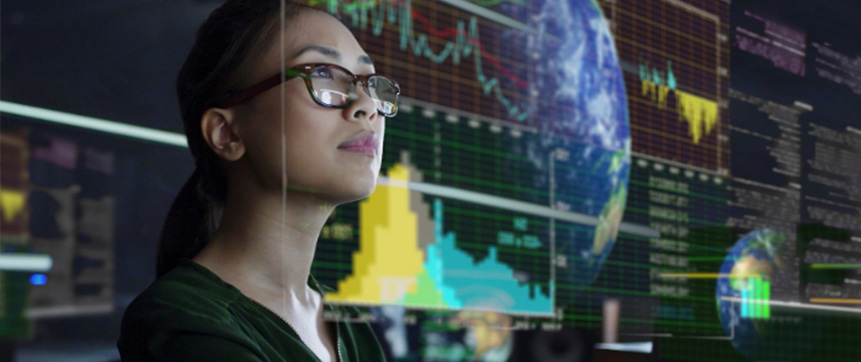
Abstract
Household and business financial stress has significant implications for financial stability and monetary policy. However, high-frequency and timely indicators of emerging signs of financial stress are not readily available. To address this information gap, the Reserve Bank has developed novel measures of financial stress based on news, search and social media data. This article describes these new metrics and how they can capture meaningful changes in financial conditions and, in some cases, predict traditional measures of financial stress, such as loan arrears. Going forward, these indices will continue to be monitored for early signs of financial difficulties.
Introduction
Not having enough money to meet basic needs or uphold financial commitments has a major impact on peoples wellbeing. The effect of financial stress can also spill over from individual households and businesses to the broader economy – and, by extension, financial stability. Financially stressed or constrained households are more likely to curb consumption in response to unexpected reductions in income or wealth, while businesses that are under financial strain may cut back on investment and employment.[1] These responses can amplify the effect of an initial shock, leading to deeper and more pronounced downturns. In extreme cases, financial stress can lead to sizable defaults on loans
Financial stress falls on a spectrum, ranging in severity from general concerns about the availability of money to difficulty paying for essential items to insolvency and default (Figure 1).[2] Households may fall into financial stress after experiencing a loss of income due to unemployment or illness, and are particularly vulnerable if they hold few liquid assets relative to debt (Wang 2022). Characteristics that may make a business more vulnerable to distress include high leverage, significant debt-servicing burden, low profitability and limited liquidity.
Reliable data on severe financial stress are provided by indicators such as non-performing loans, insolvencies, property repossessions, business administrations and court actions against companies. However, these measures are backward-looking and capture rare events that occur late in an episode of financial difficulty. Household financial stress is also measured in surveys, including the Household, Income and Labour Dynamics in Australia (HILDA) Survey and the Survey of Income and Housing. These surveys track whether households have experienced problems such as struggling to pay bills on time, needing to ask family or friends for financial help, and an inability to make rental or mortgage payments (Breunig and McKibbin 2011). While these measurements are rich in information and provide insight on the full spectrum of financial stress and the extent to which it is experienced by different groups of the population, they are neither timely nor frequent. The lack of timely data on mild-to-moderate financial stress makes it difficult to identify emerging risks.
In response to this data gap, the Bank has constructed timely, high-frequency indicators of financial stress among households and businesses, using information from Google Trends, news data and Twitter. There is a growing body of research on the benefits of using such alternative data sources to complement official statistical measures. Baker et al (2016; 2021) have measured economic uncertainty using Twitter and news data, and Google Trends has been used by Preis, Moat and Stanley (2013) to quantify trading behaviour and by Austin et al (2021) to measure economic activity.
These non-traditional data sources are generated as a side effect of some other activity, rather than being carefully designed and collected for statistical purposes to measure a given economic concept. As a result, they often reflect a somewhat biased sample of the population and are not guaranteed to accurately track the underlying concept of interest. For example, people aged over 50 years old are significantly less likely to use Twitter than younger people, so an index based on Twitter might not capture changes in the levels of financial stress experienced by older people.[3] It is therefore important that these indicators are used in conjunction with more traditional indicators and interpreted with subject matter expertise. That said, these indicators can provide timely – even real-time – insight into what is going on across broad sections of the economy, which is critical for providing early-warning indicators of potential problems. To our knowledge, this is the first time globally these non-traditional data sources have been used to construct indicators of financial stress.
Measuring stress with Google Trends, news and tweets
Our analysis of non-traditional data for monitoring financial stress is inductive. That is, we start with the data first and then explore its usefulness in providing indicators for financial stress. Three non-traditional data sources were selected to provide complimentary insights into financial stress:
- News data (Dow Jones Factiva Archive) – these data aggregate information from a wide range of sources, from expert views and opinions to personal stories of financial difficulty. The news reflects what news agencies believe is of interest to readers, which is likely to capture both the general level of concern about financial conditions in the population as well as what is politically topical and key events happening overseas.
- Social media data (Twitter) – these data provide a more direct view of what individuals, including small business owners, are concerned about, and also feeds off political and media discussion. For instance, on Twitter it is common to see users publicly tweet about examples of financial difficulties they or their friends are struggling with as it relates to broader issues being covered in the news media. Comments on events overseas are also quite common.
- Search data (Google Trends) – these data provide some insight into the private concerns of individuals, as captured by the searches they make for financial information and assistance.
All three sources are likely to provide a mixture of backward- and forward-looking views of stress. Tweets and news stories discuss both events in the past and concerns for the future. Google searches may be driven by interest in past events or by individual concerns around current and future financial stress.
This article first outlines how the Bank constructs indicators of financial stress from each of these data sources before considering what these indicators show.
Google Trends
Google Trends is a public interface for exploring the number of Google searches for specific terms or topics relative to the total volume of searches. The Banks Financial Stress Index is based on the volume of Google searches for a set of keywords, phrases and topics that people may search for when their household or business is experiencing financial stress. For example, the index includes searches such as defer my utility bill as well as searches for cash assistance and loan support. The index also incorporates topics identified by Google as relevant to financial stress keywords. (A topic is a group of search terms determined by Google to belong to the same concept; including these allows us to capture queries that relate to financial stress but do not contain the specific keywords we have specified.)
Our financial stress indices for households and businesses are constructed in three steps:
- We define a list of queries, defined by keywords or topics that are related to financial stress. See Appendix A for the full list of queries.
- For a given query, we extract its daily relative search volume compared to every other query from 2004 onwards. This requires the chaining together of multiple overlapping data requests because the Google Trends interface limits comparisons of search volumes to a maximum of five queries and only provides daily data for up to nine months. Details of this chaining process and how we normalise the results to obtain comparative relative volumes across all queries is provided in Appendix B.
- The overall financial stress indicator is constructed by summing the relative search volumes for each term so that queries with the highest relative volume contribute the most to the overall index.
For households, the index is mostly driven by concepts related to debt, followed by personal loans and bankruptcy; for businesses, the main components are related to cash-flow, liquidation and business support (Graph 1).
Movements in the business index are driven almost entirely by the experiences of small and medium-sized businesses (SMEs), given they comprise almost all businesses in Australia and so dominate search activity; furthermore, larger listed businesses are unlikely to turn to Google when experiencing financial problems as they have other resources to draw on. The indexs focus on SMEs is one of its key features as timely, high-frequency data on financial stress for smaller businesses is otherwise not readily available.
News
Our news-based indicators of financial stress are constructed using the Dow Jones Factiva Database, which is a large, international news database containing the full-text of news articles from over 30,000 sources, going back to the late 1980s. We extract all articles published in Australia that are categorised as economic news, resulting in a dataset of around 600,000 unique articles (Graph 2).
We quantify financial stress from the content of these articles by computing the net sentiment of relevant articles over time. For household financial stress, articles are selected as relevant if the article summary contains a word indicating it is about households (e.g. households, families, borrowers) or a financial commitment that could be a source of financial stress (e.g. mortgage, rent, electricity). For business financial stress, articles are included if the summary contains a word indicating it is about businesses; we attempt to filter out articles about overseas business activity.
The sentiment of each selected article is estimated using the Loughran-McDonald dictionary, which is a set of keywords tagged as positive or negative, developed specifically for financial texts using company performance filings (Loughran and McDonald 2011). The Financial Stress Index is then constructed by computing the number of positive keywords minus the number of negative keywords, divided by the total number of words in the selected articles for each month and quarter. Graph 3 shows that the keywords playing the largest role in determining sentiment are similar for those articles about businesses and those about households.
A limitation of the dictionary approach is that each word is associated with a single sentiment, regardless of the context in which it occurs. For example, the word high is labelled as positive, so the sentence high unemployment rates are affecting family cash flows would be flagged as positive even though high unemployment clearly has a negative connotation. To address this issue, we identified words that frequently occur together with sentiment keywords and manually labelled the sentiment of those pairs. This means that terms such as high unemployment get their own entry in the dictionary and are tagged with the appropriate sentiment. See Appendix C for more details.
We construct measures of financial stress based on Twitter data by tracking the proportion of all tweets from Australian users that contain keywords suggesting financial difficulties. The queries are constructed to capture both the relevant topic and negative sentiments. We do so by counting all tweets that contain pair-wise combinations of words related to household or business debt with associated negative connotations. For example, the tweet feeling overwhelmed by my mortgage would be counted in our indicator. See Appendix D for the full list of topic words and qualifiers.
The stress indicator is then a time series of these tweets as a share of the total number of tweets, which can be aggregated to a desired frequency. Tweet counts matching a given query can be obtained in real-time via Twitters Application Programming Interface (API), allowing us to construct daily indicators with no lag. Sufficient volumes of tweets to produce indices are available from 2016 onwards.
Measuring household financial stress
Evaluating the quality of our indicators is challenging due to the absence of existing, high-frequency measures of mild-to-moderate financial stress to compare them against. This notwithstanding, Graph 4 plots our new measures of household financial stress against the mortgage arrears rate.
Our benchmark, the arrears rate, has been gradually increasing since 2007.[4] This trend is broadly reflected by our indicators, except for the large spike in the news-based indicator associated with the global financial crisis. Both the arrears rate and our new measures increased sharply early in the COVID-19 pandemic, before falling below pre-pandemic levels as the federal and state governments introduced a wide range of support measures, including increased welfare benefits, pandemic leave payments, temporary loan deferrals, eviction moratoriums and wage subsidies (JobKeeper). The overall correlation to the arrears rate is around 0.8 for both the Google Trends and Twitter indices; however, it is very low for the news-based index, which was heavily influenced by the global financial crisis that drove up arrears rates in other economies while remaining low in Australia.
All three indices – particularly the more zeitgeist-driven news and Twitter indices – have risen over 2022, despite limited signs in official data of a pick-up in financial stress across Australian households as a whole. This may reflect that the new indicators capture early-stage financial stress and that the impact of the combination of higher interest rates and inflation varies significantly across households. It could also be driven by anticipation of future financial stress based on overseas news and events.
To more rigorously examine the relationship between Google Trends, Twitter and the arrears rate, we ran Granger causality tests.[5] We found that the Google Trends index Granger-causes the arrears rate, while the arrears rate does not Granger-cause the Google Trends index. Although the simple correlation between the Twitter index and the arrears rate is promising, a sufficient volume of Twitter data to produce the index is only available from 2016, which provides insufficient statistical power to confirm the form of the relationship with arrears. We did not test Granger causality for the news indicator because, due to its sensitivity to overseas events, its correlation with arrears is too low to be directly useful for forecasting. These results suggest that the Google Trend and Twitter indices could help provide an early-warning indicator of the overall level of household financial stress that is relevant both for financial stability and in anticipating how households may respond to income shocks.
By contrast, measures of financial stress from the annual HILDA Survey show no clear association with the arrears rate or our non-traditional metrics (Graph 5). While the self-assessed HILDA measures of financial stress vary meaningfully between households at a given point in time – for example, households that report higher self-assessed levels of financial stress also tend to have lower liquidity buffers and higher debt servicing ratios – they exhibit little variation over time from 2005 onwards.
Measuring business financial stress
In regard to business financial stress, our non-traditional indicators are less consistent with each other than they are for households (Graph 6). This is because they are capturing different segments of the population of Australian businesses. News articles tend to report on large (often listed) companies, including multinationals, and discuss business conditions in aggregate. On the other hand, the Google Trends index captures concerns among smaller businesses. Finally, in the sample of tweets that contribute to our business-stress indicator, there is a mix of anecdotes on the struggles involved in operating a business along with commentary on general market and economic conditions.
Despite these differences, the indices capture some meaningful changes in financial conditions. The news indicator shows a significant spike in financial stress associated with the financial crisis. This is also reflected in sharply rising business arrears rates. However, news interest in the crisis dissipated rapidly, returning to pre-financial crisis levels by 2010, whereas the arrears rate was slower to recover. Both the news and Google Trends indices show spikes in stress associated with the first major COVID-19-related lockdown in Australia in 2020, when many businesses faced enormous disruptions to their trading. Likewise, Google Trends data shows searches around business financial stress peaked again during the second lockdown. By contrast, changes in financial conditions facing businesses during the pandemic were not evident in other (late-stage) indicators of financial stress, such as business insolvencies. This is because of the significant policy support measures provided to businesses during this period, including income support, loan deferrals and temporary insolvency relief.
Another way of quantifying whether these indicators capture meaningful changes in financial conditions is to test what weight they receive if incorporated in an overall financial conditions index (FCI) for Australia. The FCI we use here is a summary measure of financial conditions that includes a broad range of indicators, including survey measures of stress as well as variables capturing interest rates and spreads, credit and money, asset prices, debt burdens, the banking sector and financial market measures of risk (Hartigan and Wright 2021). When incorporated in the FCI, the news-based indicator of business financial stress is ranked as one of the top contributors out of 76 other variables. This tells us that this measure is quite effective at capturing overall variation in financial conditions. The relevance of the business indicator can be seen directly by looking at its correspondence with the overall FCI for Australia (Graph 7). We only focus on the news-based indicator here, as the FCI model requires a longer time series than is available from Google Trends or Twitter.
Conclusion
The introduction of new high-frequency measures of household and business financial stress based on news, search and social media data is intended to complement existing, more reliable indicators by potentially providing an early warning of emerging financial stress. The Banks new Google- and Twitter-based measures of household stress are strongly associated with, and for Google Trends lead, the household arrears rate. On the business front, the new metrics capture the early-stage financial stress triggered by the COVID-19 pandemic even though, due to policy support, this stress did not ultimately flow through to increases in measures of severe financial stress such as the arrears rate. This suggests these metrics could help fill the data gap on early-stage financial stress experienced by unlisted businesses.
This is the first attempt to build financial stability indicators using non-traditional data sources. We hope to stimulate interest for further explorations of the topic. These indices will continue to be refined and modified as needed for the Banks policy publications over time, including the Financial Stability Review (RBA 2022). This includes disaggregating the indices to the state level, where possible, as well as improving the predictive capacity by pooling data across countries.
Appendix A: Terms used to construct Google Trends indicators
Query name | Query details (actual query submitted to the Google Trends API) |
---|---|
Topic:Arrears | /m/079k8t |
Topic:Bankruptcy | /m/01hhz |
Topic:Debt | /m/013y7y |
Topic:Debt collection | /m/05csgb |
Topic:Debt consolidation | /m/01nny6 |
Topic:Debt relief | /m/018ct4 |
Topic:Eviction | /m/02my10 |
Topic:Food bank | /m/059plx |
Topic:Foodbank | /g/11csq7t78m |
Topic:Foreclosure | /m/02tp2m |
Topic:Payday loan | /m/02ynk0 |
Topic:Personal loan | /g/121bdfn8 |
bankruptcy | bankrupt OR bankruptcy OR bankruptcies |
bill-assistance | (bills AND support) OR (bills AND assistance) OR (bills AND help) OR (bill AND support) OR (bill AND assistance) OR (bill AND help) |
bill-problems | (bills AND hardship) OR (bills AND behind) OR (bills AND defer) OR (bill AND hardship) OR (bill AND behind) OR (bill AND defer) |
cash-assistance | (cash AND assistance) OR (cash AND loan) OR (cash AND emergency) OR (cash AND help) |
credit-problems | (credit AND default) OR (credit AND behind) OR (credit AND problems) OR (credit AND bad) |
debt | debt |
debt-assistance | (debt AND assistance) OR (debt AND support) OR (debt AND counselling) OR (debt AND relief) |
debt-problems | (debt AND problems) OR (debt AND bad) OR (debt AND default) OR (debt AND behind) OR (debt AND defer) |
electricity-assistance | (electricity AND support) OR (electricity AND assistance) OR (electricity AND help) OR (electricity AND relief) |
electricity-problems | (electricity AND hardship) OR (electricity AND late) OR (electricity AND behind) OR (electricity AND defer) |
eviction | eviction NOT brother NOT boss NOT factor |
financial-assistance | (financial AND help) OR (financial AND assistance) OR (financial AND support) OR (financial AND counselling) |
financial-problems | (financial AND problems) OR (financial AND difficulty) OR (financial AND hardship) OR (financial AND stress) |
foodbank | foodbank OR (food AND bank) |
loan-assistance | (loan AND support) OR (loan AND assistance) OR (loan AND relief) |
loan-problems | (loan AND default) OR (loan AND behind) OR (loan AND bad) OR (loan AND defer) |
mortgage-assistance | (mortgage AND help) OR (mortgage AND support) OR (mortgage AND assistance) OR (mortgage AND relief) |
mortgage-problems | (mortgage AND default) OR (mortgage AND behind) OR (mortgage AND defer) OR (mortgage AND stress) |
payment-assistance | (payment AND plan) OR (payment AND defer) OR (payment AND assistance) OR (payment AND relief) |
rent-assistance | (rent AND help) OR (rent AND support) OR (rent AND assistance) OR (rent AND relief) |
rent-problems | (rent AND problems) OR (rent AND bad) OR (rent AND behind) OR (rent AND arrears) OR (rent AND stress) |
Source: Google; RBA |
Query name | Query details (actual query submitted to the Google Trends API) |
---|---|
Topic:Cash flow | /m/0f29w |
Topic:Debt consolidation | /m/01nny6 |
Topic:Insolvency | /m/04tmq2 |
Topic:Liquidation | /m/02ql2v |
business-restructure | business AND restructure |
business-shutdown | (business AND shut) OR (business AND shutdown) OR (business AND close) OR (business AND liquidate) |
business-support | (business AND hardship) OR (business AND grant) OR (business AND support) OR (business AND assistance) |
closing-down | closing down |
insolvent | insolvency OR insolvent OR insolvent OR insolvency |
layoff | layoff OR retrench |
liquidate | liquidation OR liquidate |
voluntary-administration | voluntary AND administration |
Source: Google; RBA |
Appendix B: Normalising Google Trends results
The frequency at which Google Trends provides data depends on the total length of the period searched (Table B.1). A given query can contain up to five keywords or topics, allowing the comparison of the relative volume of these queries over time. To obtain high-frequency data back to 2004 over a large number of keywords and topics, we run multiple overlapping queries – both over time and search terms. We then normalise the results to make the data comparable across all queries and time periods by scaling the raw results such that the mean values of the overlapping results match.
Let and be the relative search volumes of a query on day for overlapping time periods and , with days in common . Compute and . Normalise relative to by letting .
Relative search volumes are returned as an integer between zero and 100. The discretization can lead to significant clipping of low-volume search terms if they are queried relative to a high-volume term. To mitigate this issue, we run an initial set of queries with the terms grouped into random, overlapping sets of five to get an overall estimate of the relative volume of the queries. We then sort them into new groups based on approximate relative volume and rerun the queries to get a more fine-grained estimate.
Total length of query period | Frequency of returned data |
---|---|
< 7 days | hourly |
7 days to < 9 months | daily |
9 months to < 5 years | weekly |
5 years + | monthly |
Sources: Google; RBA |
Appendix C: Extending the Loughran-McDonald dictionary
Dictionary-based approaches to sentiment classification are simple to apply and interpret. However, they do not take into account the context in which a word appears. Machine-learning-based sentiment classification addresses this issue but is computationally expensive and more difficult to interpret. To mitigate for the limitations of the dictionary approach, we identify words that frequently occur in conjunction with a sentiment word from the Loughran-McDonald dictionary and manually label the sentiment of the resulting phrases. We also collect any phrases where the phrase sentiment differs to the underlying word sentiment – for example, high is positive but high-inflation is negative – and add these phrases to the dictionary.
To identify phrases, we estimate an approximate conditional probability ratio:
Where:
- is the total number of words in the news corpus
- is the number of times word a occurs
- is the number of times word b occurs
- is the number of times word a occurs followed by word b.
Large scores indicate that observing word a makes it significantly more likely that the next word will be b than if words were randomly selected based on their overall frequency.
A computer-readable list of all the phrases we have extracted with their sentiment labels is available upon request.
Appendix D: Search terms for Twitter indicators
Keyword | Negative connotation | |||
---|---|---|---|---|
Businesses | Households | Government (excluded) | ||
cash flow | loan | government | arrears | strains |
Insolvency | mortgage | morrison | bad | stresses |
bankrupt | credit | treasury | behind | struggle |
balance sheet | debt | leadership | concerns | suffered |
budget | finances | nation | defaults | suffering |
business loan | serviceability | national | deficits | tense |
capital | repayment | public | doubts | tension |
liability | repayments | political | endanger | tepid |
credit risk | interest rate | politics | failed | threats |
profit | interest rates | sovereign | failing | tough |
equity | home loan | failures | troubled | |
asset | home equity | fallen | tumbling | |
subsidy | rent | faltering | turbulent | |
production | headwinds | turmoil | ||
trade | impaired | unable | ||
stock price | impairing | unrest | ||
share price | inability | unstable | ||
business | insolvent | volatile | ||
liquidity | poorer | weakened | ||
market | problems | weakening | ||
business debt | riskier | weaker | ||
company debt | setbacks | weakest | ||
recruiting | severely | worries | ||
profitability | severity | worrying | ||
regulation | shortages | |||
investment | ||||
fixed cost | ||||
sunk cost | ||||
wage | ||||
liquidation |
Endnotes
The authors are from Economic Research Department and would like to thank Cathie Close, Callan Windsor and John Simon for their feedback on this work. [*]
See Johnson, Parker and Souleles (2006); Kaplan, Violante and Weidner (2014); Albuquerque and Green (2022); Murillo, Graham and Harvey (2010); Gómez (2019). [1]
Figure 1 is adapted from Bullock (2018). [2]
Statista Research Department (2022), Twitter usage in Australia in 2018 by generation. [3]
For a discussion on the potential causes of this rise, see Kearns (2019). [4]
We use a VAR model with the BIC criteria to select the number of lags to include and difference the data to deal with any non-stationarity. [5]
References
Albuquerque B and G Green (2022), Financial Concerns and the Marginal Propensity to Consume in COVID Times: Evidence from UK Survey Data, IMF Working Paper No 2022/047.
Austin P, M Marini, A Sanchez, C Simpson-Bell and J Tebrake (2021), Using the Google Places API and Google Trends Data to Develop High Frequency Indicators of Economic Activity, IMF Working Paper No 2021/295.
Baker S, N Bloom and S Davis (2016), Measuring Economic Policy Uncertainty, The Quarterly Journal of Economics, 131(4), pp 1593–1636.
Baker S, N Bloom, S Davis and T Renault (2021), Twitter-derived Measures of Economic Uncertainty, 13 May.
Breunig R and McKibbin R (2011), The Effect of Survey Design on Household Reporting of Financial Difficulty, Journal of the Royal Statistical Society: Series A (Statistics in Society), 174(4), pp 991–1005.
Bullock M (2018), Household Indebtedness and Mortgage Stress, Address to the Responsible Lending and Borrowing Summit, Sydney, 20 February.
Gómez M (2019), Credit Constraints, Firm Investment and Employment: Evidence from Survey Data, Journal of Banking and Finance, 99, pp 121–141.
Hartigan L and M Wright (2021), Financial Conditions and Downside Risk to Economic Activity in Australia, RBA Research Discussion Paper No 2021-03.
Johnson D, J Parker and N Souleles (2006), Household Expenditure and the Income Tax Rebates of 2001, American Economic Review, 96(5), pp 1589–1610.
Kaplan G, G Violante and J Weidner (2014), The Wealthy Hand-to-Mouth, Brookings Papers on Economic Activity, Spring, pp 77–138.
Kearns J (2019), Understanding Rising Housing Loan Arrears, Speech to the 2019 Property Leaders Summit, Canberra, 18 June.
Loughran T and B McDonald (2011), When Is a Liability Not a Liability? Textual Analysis, Dictionaries, and 10‐Ks, The Journal of Finance, 66(1), pp 35–65.
Murillo C, Graham J and Harvey C (2010), The Real Effects of Financial Constraints: Evidence from a Financial Crisis Journal of Financial Economics, 97(3), pp 470–487.
Preis T, H Moat and H Stanley (2013), Quantifying Trading Behaviour in Financial Markets Using Google Trends, Scientific Reports, 3(1), pp 1–6.
RBA (Reserve Bank of Australia) (2022), Graph 2.3, Financial Stability Review, October.
Statista Research Department (2022), Twitter Usage in Australia in 2018 by Generation. Available at <https://www.statista.com/statistics/891903/australia-twitter-usage-share-by-generation>
Wang L (2022), Household Liquidity Buffers and Financial Stress, RBA Bulletin, June.