RDP 2014-12: A State-space Approach to Australian GDP Measurement 5. Comparison with GDP(A)
October 2014 – ISSN 1320-7229 (Print), ISSN 1448-5109 (Online)
- Download the Paper 904KB
It is natural to compare the performance of our models against GDP(A). We first describe the statistical properties of our GDP measures, and we then examine whether our GDP measures are better able to explain and forecast unemployment and inflation.
5.1 How Volatile is GDP Growth?
Visual inspection suggested that our measures of GDP growth smooth out some of the volatility in the published ABS series. A statistical analysis of the alternative GDP measures confirms this conjecture.
Table 5 compares moments of the published GDP series to those of our models. The mean of our models is similar to those of the published series. However, other moments of the distributions differ. All three of our constructed measures are considerably less volatile than the ABS series, with the standard deviation of GDP growth being around one-third lower in our series than in GDP(A).
Parameter | ABS series | GDP(M) series | ||||||
---|---|---|---|---|---|---|---|---|
GDP(A) | GDP(E) | GDP(I) | GDP(P) | Model 1 | Model 2 | Model 3 | ||
Moments | ||||||||
Mean | 0.79 | 0.80 | 0.78 | 0.80 | 0.79 | 0.79 | 0.79 | |
σ | 0.76 | 0.93 | 1.03 | 0.84 | 0.57 | 0.56 | 0.49 | |
ρ1 | 0.21 | −0.03 | −0.19 | 0.31 | 0.47 | 0.79 | 0.68 | |
Results from an AR(1) regression | ||||||||
RSE | 0.74 | 0.93 | 1.02 | 0.80 | 0.51 | 0.34 | 0.36 | |
R2 | 0.04 | 0.00 | 0.04 | 0.10 | 0.22 | 0.63 | 0.47 | |
Notes: The sample period is 1980:Q1–2013:Q2; model-based statistics are for the posterior median estimate of true GDP; σ = standard deviation, ρ1 is the first-order correlation coefficient, RSE = residual standard error from a fitted AR(1) model |
Our measures of GDP growth are also more persistent, with the correlation coefficients on our measures of GDP growth far larger than on the ABS series. As a consequence, our measures of GDP growth are also more predictable; an estimated AR(1) model of our constructed GDP series produces a far closer fit than it does for standard measures of GDP.
5.2 Real-time Performance
In order to produce timely estimates, statistical agencies publish GDP before all information sources are available. They then revise these preliminary estimates as more information comes to light.[16] Bishop et al (2013) find that initial estimates of Australian GDP often differ substantially from later, more informed estimates. Knowing this, users may prefer measures that are less subject to revision, as long as those measures are close approximations to ‘true’ output growth.
We use real-time estimates of GDP(E), GDP(I) and GDP(P) to construct a history of real-time model estimates from 2001:Q1 to 2013:Q2.[17] We evaluate the real-time performance of our models using two common metrics: ‘mean absolute revision’ and ‘mean revision’. Mean absolute revision measures the average size of revisions regardless of sign. Mean revision is the average of revisions and can be interpreted as a tendency for GDP to be revised in a particular direction, that is, whether it is biased. Table 6 presents these statistics for GDP(A) and our models over the period 2001:Q1 to 2009:Q3. Final GDP is defined as the estimate after four years, consistent with Bishop et al (2013).
Measure | GDP(A) | Model 1 | Model 2 | Model 3 |
---|---|---|---|---|
Mean absolute revision | 0.29 | 0.20 | 0.19 | 0.18 |
Mean revision | 0.13 | 0.11 | 0.10 | 0.05 |
Notes: The sample period is 2001:Q1–2009:Q3; revisions are calculated as the difference between each measure's growth estimate after four years and its initial growth estimate |
Our models are more reliable than GDP(A) in real time, with the mean absolute revisions statistically smaller than for GDP(A) at the 5 per cent level. These differences are economically meaningful as well; revisions to our models are around a third smaller than revisions to GDP(A). Consistent with Bishop et al (2013), over this sample period there has been a slight upward tendency to revisions, and this is evident across the measures, although slightly less so for the model estimates.
In addition, our models' GDP growth estimates are also easier to forecast in real time. The root mean squared errors for out-of-sample AR(1) forecasts are 0.39 percentage points for Model 1 and 0.35 percentage points for Models 2 and 3, compared with 0.52 percentage points for GDP(A). This suggests that contemporaneous estimates from our models may provide a better indication of future outturns than GDP(A).
Table 7 shows that our models also converge to their final values more quickly than GDP(A). The performance of Model 1 is particularly noteworthy as this model is highly correlated with GDP(A).
Measure | GDP(A) | Model 1 | Model 2 | Model 3 |
---|---|---|---|---|
Initial | 0.29 | 0.20 | 0.19 | 0.18 |
One year | 0.25 | 0.17 | 0.16 | 0.11 |
Two years | 0.22 | 0.15 | 0.12 | 0.08 |
Three years | 0.17 | 0.10 | 0.09 | 0.06 |
Notes: The sample period is 2001:Q1–2009:Q3; errors are calculated as the difference between each measure's growth estimate after four years and its growth estimate at the specified horizon |
5.3 Explaining Macroeonomic Relationships
In this section we examine whether our measures of GDP display a closer relationship with unemployment and inflation than GDP(A).
5.3.1 Unemployment
Macroeconomic theories typically predict a close relationship between output growth and unemployment, a relationship known as ‘Okun's law’. Figure 8 illustrates the Okun's law relationship for Australia for GDP(A) and Models 1 and 2.[18] As theory would suggest, for all three measures, lower output growth is associated with an increase in the unemployment rate. But the relationship between changes in unemployment and changes in GDP appears stronger for Models 1 and 2 than for GDP(A), represented by steeper fitted lines and higher adjusted R2s.
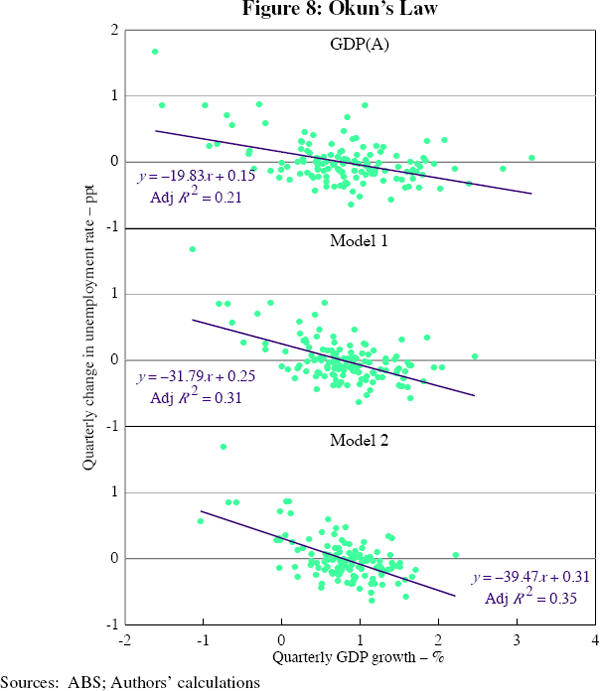
We confirm this result more formally by examining the in-sample fit of our models and GDP(A). To do this, we estimate the specification:
where Δyt−i is the quarterly growth rate of a measure of GDP in quarter t − i. The long-run response of unemployment to changes in output, known as ‘Okun's coefficient’, can be approximated by:
Table 8 presents our results. The estimated coefficients are mostly statistically significant and are of the expected sign. The regressions including Models 1 and 2 appear to fit the data better than those including GDP(A), as shown by the adjusted R2s, although the difference is not statistically significant. The coefficients on the lagged changes in unemployment are smaller in the regressions with our model measures, suggesting that our measures contribute more information than GDP(A) or a random walk. Finally, the coefficients on contemporaneous and lagged values of output growth are larger for our models than for GDP(A), reflected in larger Okun's coefficients. This may indicate attenuation bias in the regressions including GDP(A), caused by the presence of measurement error.
Parameter | GDP(A) | Model 1 | Model 2 |
---|---|---|---|
α | 0.23** | 0.32** | 0.34** |
ΔUt−1 | 0.36** | 0.29** | 0.25** |
Δyt | −0.12** | −0.17** | −0.11* |
Δyt−1 | −0.13** | −0.14** | −0.27** |
Δyt−2 | −0.04 | −0.08* | −0.05 |
Implied Okun's coefficient | −0.45 | −0.56 | −0.59 |
Adj R2 | 0.54 | 0.57 | 0.60 |
Notes: The sample period is 1980:Q4–2013:Q2; ** and * represent significance at the 1 and 5 per cent levels, respectively; the models were estimated using robust (White 1980) standard errors |
Despite the better in-sample fit, we find that our models do not improve real-time forecasting. Table 9 presents root mean squared errors for one-step-ahead forecasts of the unemployment rate, incorporating either GDP(A), Model 1 or Model 2. We find that over the sample period, root mean squared errors were broadly similar across the measures, suggesting that the models are similarly useful at forecasting unemployment.[19]
Measure | GDP(A) | Model 1 | Model 2 |
---|---|---|---|
Root mean squared error | 0.20 | 0.20 | 0.20 |
Mean error | 0.02 | 0.03 | 0.04 |
Note: The sample period is 2001:Q1–2013:Q2 |
5.3.2 Inflation
We now examine whether our model measures of GDP are useful for explaining inflation. We estimate an expectations-augmented mark-up model of inflation, following Norman and Richards (2010). The model includes terms for bond market inflation expectations (E(π)), unit labour costs (ulc), import prices (mp) and the output gap (gap). The dependent variable is the rate of inflation (π). Like Norman and Richards, we choose a polynomial distributed-lag specification as follows:
Our model measures of GDP enter the mark-up model through the output gap, where potential GDP growth is derived using a Hodrick-Prescott (HP) filter.[20]
Table 10 presents the results. Our GDP measures seem to fit about as well as GDP(A). The coefficients on the output gap terms for all of the measures are statistically significant and of a similar magnitude.[21] The models appear to fit the data similarly well, with the adjusted R2 for each model around 0.6. Overall, our GDP measures appear about as useful as GDP(A) in explaining inflation.
Parameter | GDP(A) | Model 1 | Model 2 | Model 3 |
---|---|---|---|---|
α | 0.00** | 0.00** | 0.00** | 0.00** |
Et−1(πt+S) | 0.16** | 0.17** | 0.17** | 0.16** |
Δulct−j | 0.36** | 0.35** | 0.34** | 0.33** |
Δmpt−k | 0.12** | 0.12** | 0.11** | 0.12** |
gapt−1 | 0.07** | 0.08** | 0.08** | 0.07** |
Adj R2 | 0.60 | 0.60 | 0.60 | 0.60 |
Notes: The sample period is 1990:Q1–2013:Q2; ** represents significance at the 1 per cent level; where multiple lags are included, coefficients are the sum of the lags |
Footnotes
See Bishop et al (2013) for a discussion of the revisions process. [16]
Due to the time required for estimation, we re-estimate the model every four quarters using real-time data. We use these parameter estimates, combined with real-time national accounts data, to produce estimates for the subsequent three quarters. [17]
We exclude Model 3 because it is identified using the unemployment rate. [18]
The similar forecasting performance of the models may be the result of timing issues. In Table 9 we use the timing of the RBA's Statement on Monetary Policy to simulate the RBA staff's forecasting experience, where applicable. At that time, GDP for the previous quarter is not available because the ABS release the national accounts with a delay of a little over two months. The forecast specification of Equation (5) includes lags that are a fair way down the lag structure and, therefore, lack forecasting power. [19]
The output from the estimation procedures outlined in Section 3 is in one-quarter changes. The HP filter is run over a levels index constructed using these quarterly growth rates. We introduce levels versions of our model measures in Appendix B. [20]
One explanation for the similarity of coefficients is that, since our measure of the output gap is based on the accumulation of quarterly growth rates, quarter-to-quarter errors in GDP(A) will wash out to some degree, leaving all gap estimates quite similar. [21]